Weighted residual self-attention graph-based transformer for spectral-spatial hyperspectral image classification
INTERNATIONAL JOURNAL OF REMOTE SENSING(2023)
摘要
Recently, deep learning for hyperspectral image classification has been successfully applied, and some convolutional neural network (CNN)-based models already achieved attractive classification results. Since hyperspectral data is a spectral-spatial cube data that can generally be considered as sequential data along with the spectral dimension, CNN models perform poorly on such a sequential data. Unlike convolutional neural networks (CNNs) that mainly concern with local relationship models in images, transformer has been shown to be a powerful structure for qualifying sequential data. In the SA (self-attention) module of ViT, each token is updated through aggregating all token's features based on the self-attention graph. Through this, tokens can exchange information sufficiently among each other which provides a powerful representation capability. However, as the layers become deeper, the transformer model suffers from network degradation. Therefore, in order to improve the layer-to-layer information exchange and alleviate the network degradation problem, we propose a Weighted Residual Self-attention Graph-based Transformer (RSAGformer) model for hyperspectral image classification with respect to the self-attention mechanism. It effectively solves the network degradation problem of deep transformer model by fusing the self-attention information between adjacent layers and extracts the information of data effectively. Extensive experiment evaluation with six public hyperspectral datasets shows that the RSAGformer yields competitive results for classification.
更多查看译文
关键词
Hyperspectral image classification,transformer,Weighted Residual Self-attention Graph-based Transformer,deep learning
AI 理解论文
溯源树
样例
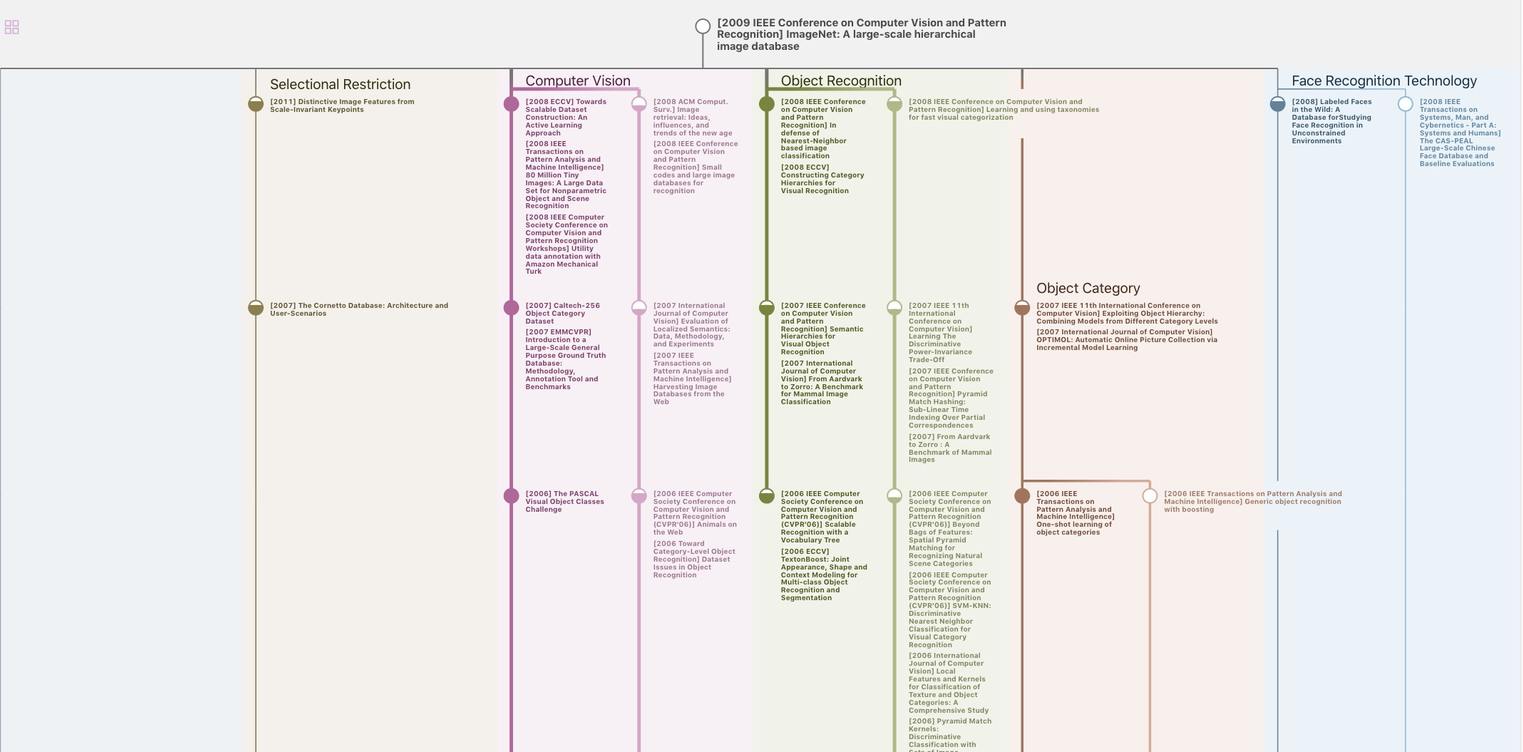
生成溯源树,研究论文发展脉络
Chat Paper
正在生成论文摘要