MFANet: A Collar Classification Network Based on Multi-Scale Features and an Attention Mechanism
MATHEMATICS(2023)
摘要
The collar is an important part of a garment that reflects its style. The collar classification task is to recognize the collar type in the apparel image. In this paper, we design a novel convolutional module called MFA (multi-scale features attention) to address the problems of high noise, small recognition target and unsatisfactory classification effect in collar feature recognition, which first extracts multi-scale features from the input feature map and then encodes them into an attention weight vector to enhance the representation of important parts, thus improving the ability of the convolutional block to combat noise and extract small target object features. It also reduces the computational overhead of the MFA module by using the depth-separable convolution method. Experiments on the collar dataset Collar6 and the apparel dataset DeepFashion6 (a subset of the DeepFashion database) show that MFANet is able to perform at a relatively small number of collars. MFANet can achieve better classification performance than most current mainstream convolutional neural networks for complex collar images with less computational overhead. Experiments on the standard dataset CIFAR-10 show that MFANet also outperforms current mainstream image classification algorithms.
更多查看译文
关键词
deep learning,image classification,collar classification,attention mechanism,multi-scale
AI 理解论文
溯源树
样例
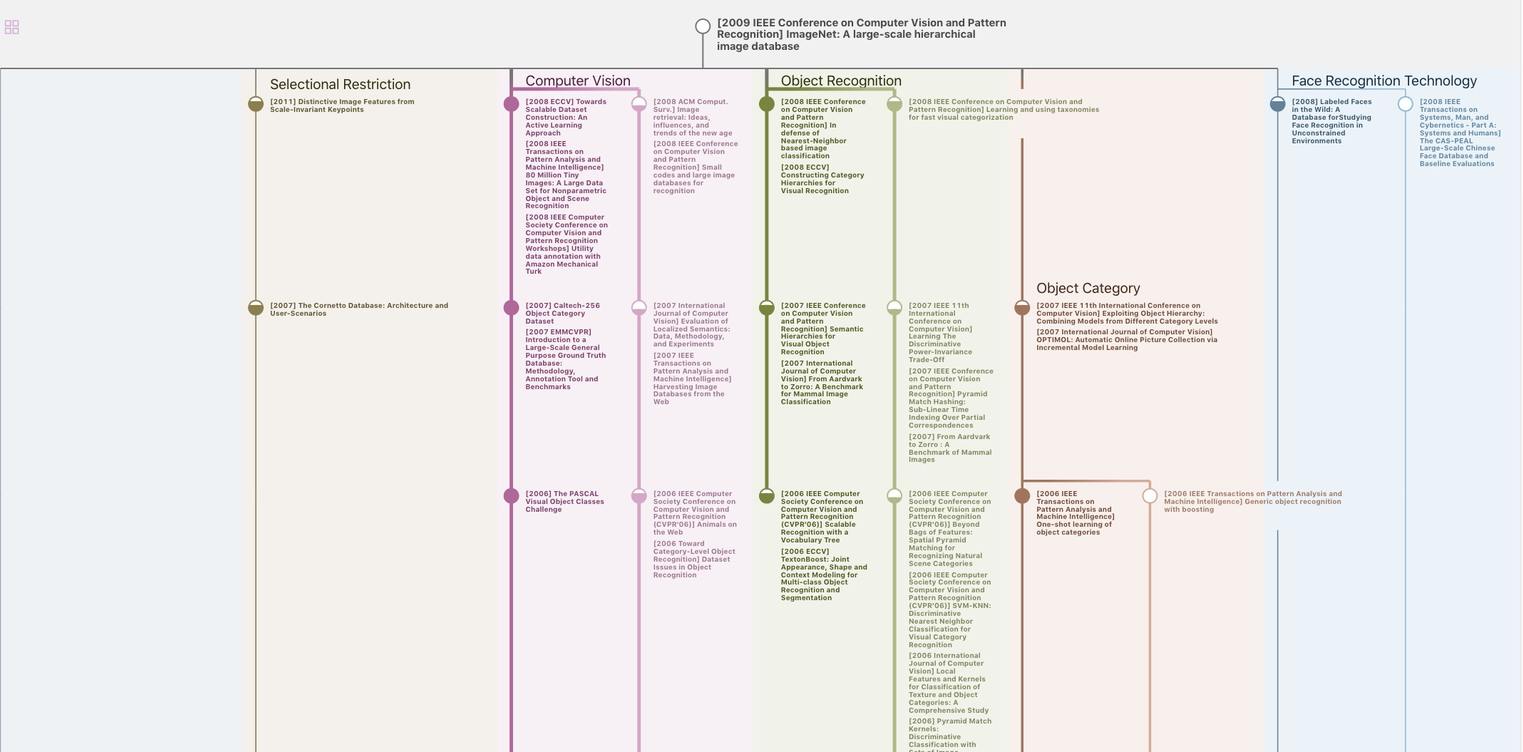
生成溯源树,研究论文发展脉络
Chat Paper
正在生成论文摘要