An adaptive multi-class imbalanced classification framework based on ensemble methods and deep network
NEURAL COMPUTING & APPLICATIONS(2023)
摘要
Data imbalance is one of the most difficult problems in machine learning. The improved ensemble learning model is a promising solution to mitigate this challenge. In this paper, an improved multi-class imbalanced data classification framework is proposed by combining the Focal Loss with Boosting model (FL-Boosting). By addressing the confusion of the second-order derivation of Focal Loss in the traditional ensemble learning model, the proposed model achieves a more efficient and accurate classification of the imbalanced data. More specifically, a Highly Adaptive Focal Loss (HAFL) is proposed to ensure that the model maintains lasting attention to the minority samples, which could be combined with boosting model to build HAFL-Boosting to achieve better performance. The framework has the scalability to adapt to different situations according to typical ensemble learning algorithms such as LightGBM, XGBoost and CatBoost. In addition, to implement the application of the proposed framework on deep models, a two-stage classification method combining ConvNeXt with the improved boosting model is proposed, which could improve the recognition ability to high-dimensional imbalanced data. We evaluate the HAFL-Boosting and the two-stage class imbalance classification method by ablation experiments and benchmark experiments, which demonstrated that the proposed methods obviously improved the scores on several evaluation indexes. The comparative experiments with the latest classification models show that the proposed methods could achieve leading performance from multiple perspectives.
更多查看译文
关键词
Multi-class imbalanced classification framework,Improved focal loss,HAFL-boosting,Two-stage classification method
AI 理解论文
溯源树
样例
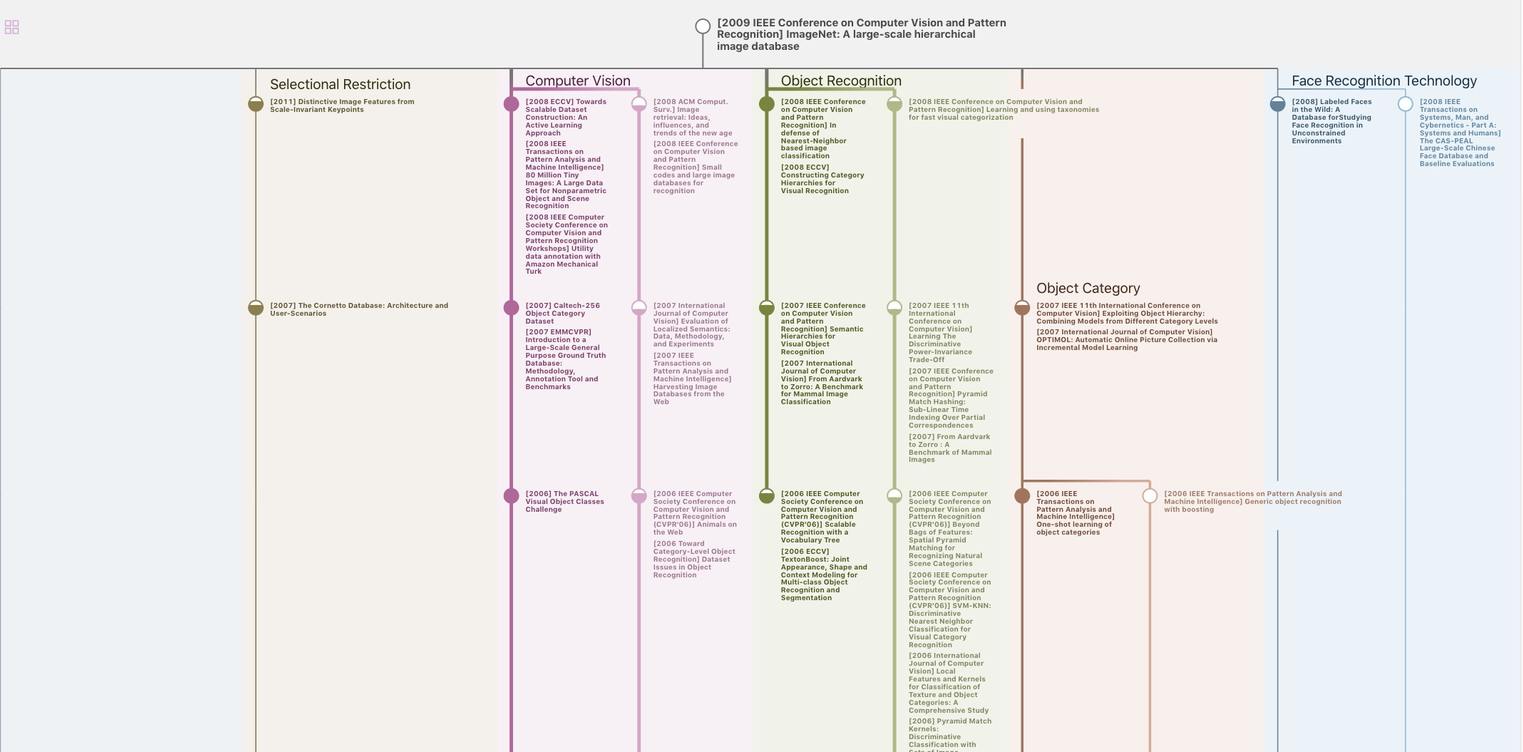
生成溯源树,研究论文发展脉络
Chat Paper
正在生成论文摘要