FedSeq: A Hybrid Federated Learning Framework Based on Sequential In-Cluster Training
IEEE SYSTEMS JOURNAL(2023)
摘要
Federated learning (FL) enables multiple devices to collaboratively accomplish a machine learning task by iteratively exchanging their model updates instead of raw data with a parameter server (PS), thus protecting users' data privacy. Nevertheless, the communication efficiency and the nonindependent and identically distributed (non-IID) data are remaining intractable challenges in FL. This article proposes a hybrid FL framework called FedSeq, based on user clustering and sequential in-cluster training, to improve the communication efficiency and the test accuracy, especially on non-IID data. The FedSeq first divides users into multiple clusters and selects a cluster head (CH) on behalf on each cluster to upload the model updates to the PS, thus drastically reducing the uplink communication overhead. Within each cluster, a sequential training method is designed, which enables the CHs' models witness more categories of training data and go through more meta-updates per training epoch, thus promoting the test accuracy. Moreover, we also provide the convergence analysis of FedSeq on IID data with Random user clustering, with specific experiments validating our theoretical analysis from a simulation perspective. In the experiments, we compare our FedSeq with other baselines, including FedSGD, FedAVG, FedProx, FedCluster, and HierFAVG, of which the results demonstrate that FedSeq outperforms other FL paradigms in terms of model accuracy and training efficiency. We also test FedSeq with Random and Low-Energy Adaptive Clustering Hierarchy clustering strategies to demonstrate the robustness of FedSeq to different clustering strategies. The FedSeq offers a potential solution to cope with non-IID data and reduce uplink communication overhead in FL.
更多查看译文
关键词
Training,Data models,Adaptation models,Servers,Training data,Load modeling,Convergence,Federated learning (FL),sequential in-cluster training,user clustering
AI 理解论文
溯源树
样例
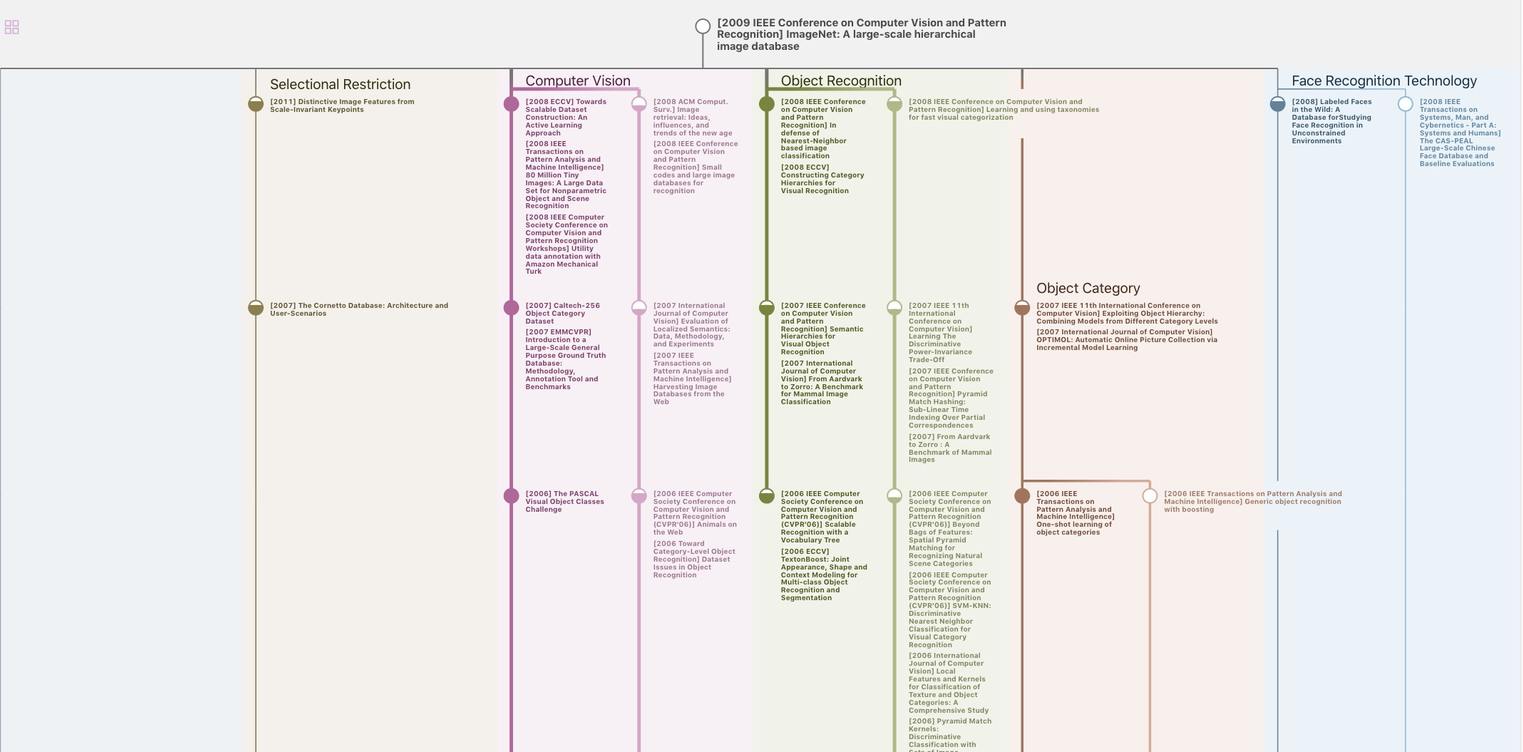
生成溯源树,研究论文发展脉络
Chat Paper
正在生成论文摘要