A novel framework for direct multistep prediction in complex systems
NONLINEAR DYNAMICS(2023)
摘要
Multistep prediction is an open challenge in many real-world systems for a long time. Despite the advantages of previous approaches, e.g., step-by-step iteration, they have some shortcomings, such as accumulated errors, high cost, and low interpretation. To this end, Gaussian process regression and delay embedding are used to create a combination framework, namely spatial–temporal mapping (STM). Delay embedding is employed to reconstruct an isomorphic dynamical structure with the original system through a single time series, which provides the fundamental architecture for multistep predictions (interpretation). Gaussian process regression is used to achieve predictions by identifying a mapping between the reconstructed dynamical structure and the original structure. This combination framework outputs multistep ahead predictions in a single step (low cost). We test the feasibility of STM for both model systems, including the 3-species ecology system, the Lorenz chaotic system, and the Rossler chaotic system, and several real-world systems, involving energy, finance, life science, and climate. STM framework outperforms traditional iterative approaches and has the potential for many other real-world systems.
更多查看译文
关键词
Chaos,Machine learning,Delay embedding,Time series
AI 理解论文
溯源树
样例
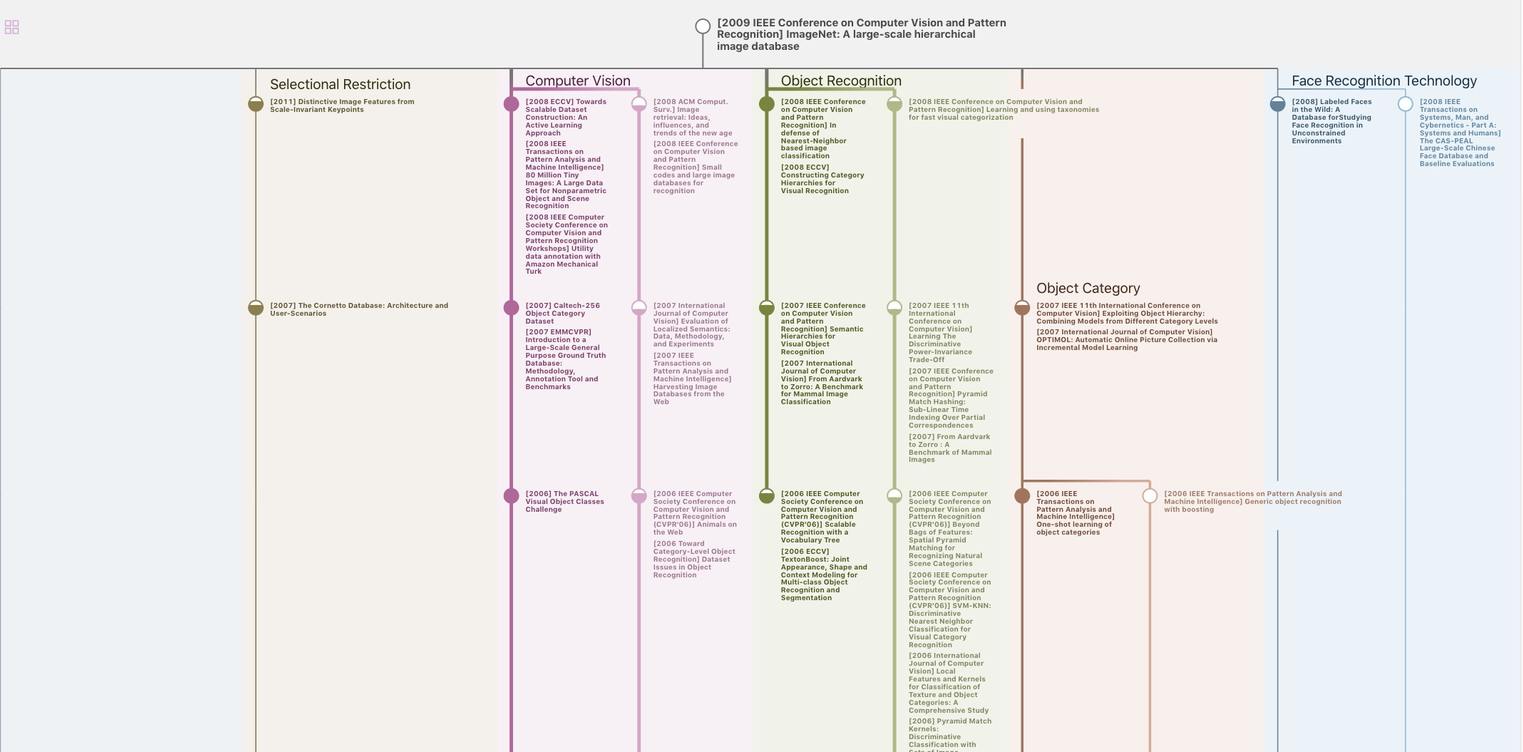
生成溯源树,研究论文发展脉络
Chat Paper
正在生成论文摘要