A comparative study on the performance of field-sampled asphalt mixtures for heavy-duty pavements using laboratory testing and mechanistic-empirical simulations
ROAD MATERIALS AND PAVEMENT DESIGN(2023)
摘要
The demand for durability and resilience in asphalt pavements has led to the progressive implementation of performance-enhancing technologies like bitumen modification using polymers or fibers. This is particularly important in a port environment, where roads are subjected to heavy traffic. The main objective of this study is to contribute to the current state of knowledge on the performance of asphalt mixtures, including polymers and fibers, by conducting a comprehensive laboratory investigation of eight field mixtures, followed by an evaluation of their expected performance in five test sections using the AASHTOWare Pavement ME Design software. The eight mixtures included two lean asphalt mixes to be used as a replacement for granular layers, three base mixtures, and three surface mixtures. Laboratory testing showed that the addition of a blend of 91% polypropylene and 9% glass fibers increased stiffness and resistance to moisture damage. Also, this fiber blend improved cracking resistance when used in a relatively flexible mixture. It was found that top-down cracking predictions followed the trend observed in semicircular bending tests. Conversely, the predicted rutting performance did not align with the rut depth trend in the laboratory. Furthermore, the use of SMA instead of a dense-graded surface layer appeared to increase fatigue cracking in pavement sections with granular bases unless a high-modulus base asphalt layer was used. This study was a first attempt to use advanced pavement performance predictions in Belgium. The data gathered during the future monitoring of the test sections built as part of this study will provide valuable primary data for the local calibration of pavement distress models.
更多查看译文
关键词
Asphalt,polymer-modified binder,fiber,pavement performance,mechanistic-empirical design
AI 理解论文
溯源树
样例
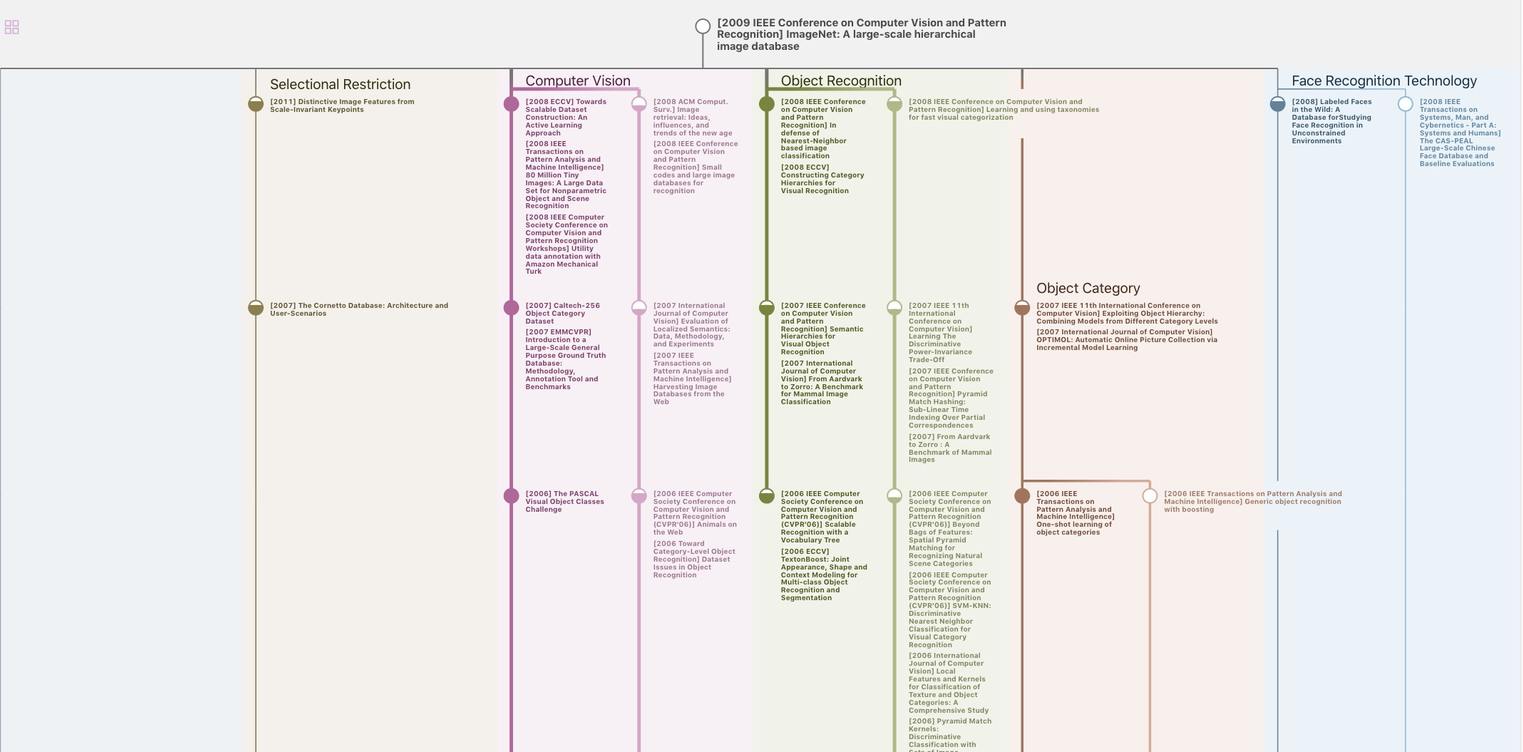
生成溯源树,研究论文发展脉络
Chat Paper
正在生成论文摘要