An Accurate Analogy Based Software Effort Estimation Using Hybrid Optimization and Machine Learning Techniques
Multimedia tools and applications(2023)
摘要
Software engineering’s primary task is analogy-centric effort estimation. In this, by utilizing the existent histories, the effort needed for new software projects was estimated for the respective development along with management. Generally, the Software Effort Estimation (SEE) methodologies’ higher correctness is a non-solvable issue, which was termed as a multi-objective problem. In recent days, Machine Learning (ML) methodologies are utilized by numerous authors for the same process; however, higher performance was not attained. Furthermore, bias and subjectivity issues are the complications faced by the prevailing SEE methodologies. For further improvement of effort estimation, we propose an accurate analogy based SEE (AA-SEE) created on hybrid optimization and ML techniques. The first contribution of the proposed AA-SEE technique is to introduce a multi-swarm coyote optimization (MSCO) algorithm to tune the hyper parameters for ML technique. Because, an accurate hyper parameters needed for effort estimation at the optimal level which reduce the prediction errors. The second contribution is to illustrate the teaching-learning based recurrent neural network (TL-RNN) for effort estimation. The proposed AA-SEE technique can be evaluate through different standard datasets are Albercht, Kitchenham, Maxwell, Deshernais, IKH, Telecom, ISBSG and NASA. Finally, the performance of proposed AA-SEE technique is associated with the existing state-of-art methodologies in footings of accuracy, MMRE, MdMMRE, BMMRE, MMER and MdMMER.
更多查看译文
关键词
Software engineering,Effort estimation,Prediction errors,Hyperparameter tuning,TL-RNN
AI 理解论文
溯源树
样例
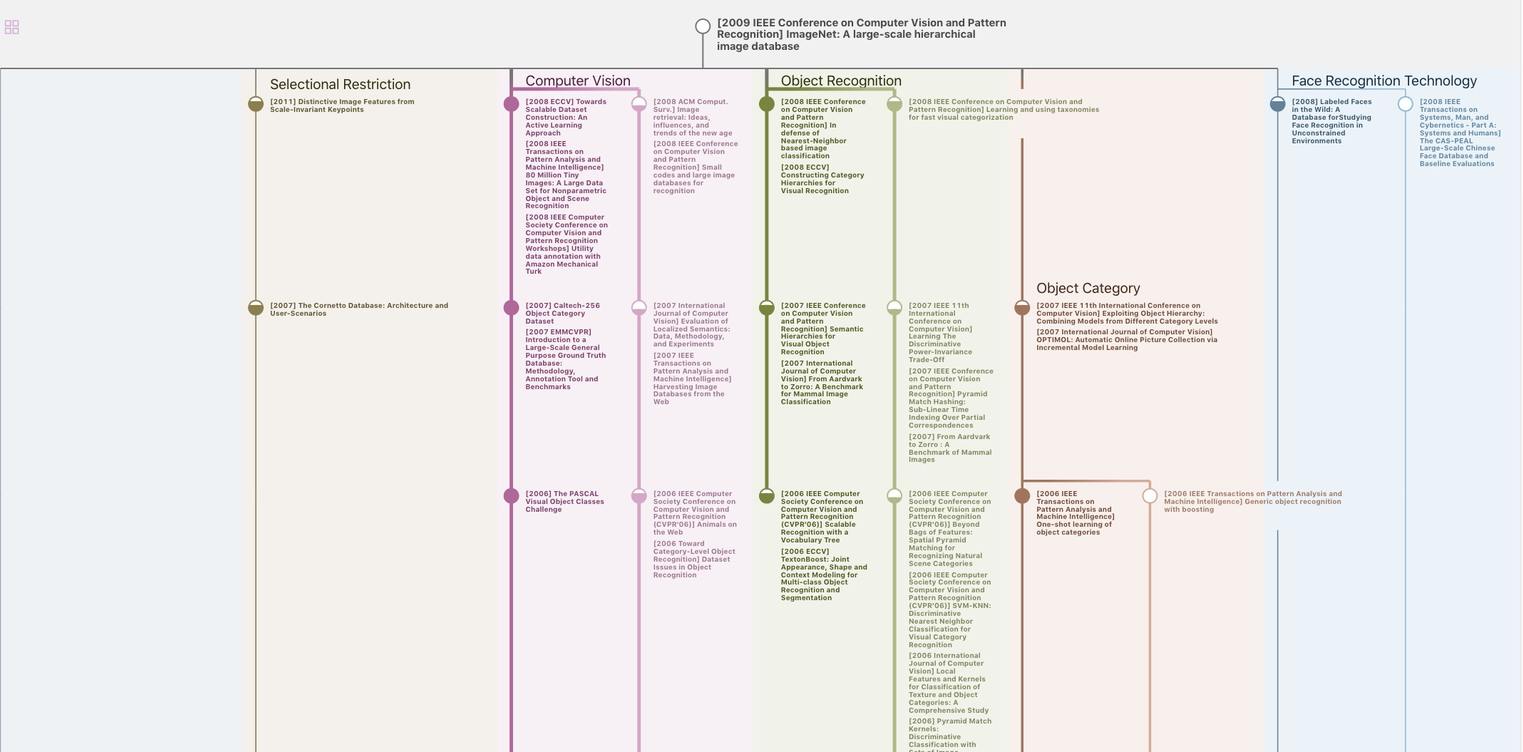
生成溯源树,研究论文发展脉络
Chat Paper
正在生成论文摘要