Characterisation for spatial distribution of mining-induced stress through deep learning algorithm on SHM data
GEORISK-ASSESSMENT AND MANAGEMENT OF RISK FOR ENGINEERED SYSTEMS AND GEOHAZARDS(2023)
摘要
The study of mining-induced stress is essential to ensure the safety production of coalmine. Due to the limited number of monitoring points and local monitoring area, the perception of structure status is insufficient. This study aims to present a deep learning (DL) model to derive the stress distribution characteristics of the overall coalmine roof. First, the framework of spatial deduction model termed as transferring convolutional neural network (TCNN) is presented, where the convolutional neural network is transferred on different datasets. According to this framework, the spatial correlations of structural mechanical responses at different heights above roadway roof are learned through numerical simulation. Subsequently, the learned results are transferred to monitoring data to derive the actual state of the overall roof. In order to verify the reliability of the TCNN model, the stress sensor is installed in the derived plane to collect the actual data, and two indicators are adopted to evaluate the reasonability of deduction results. Experimental results indicated that 92.25% features of mining-induced stress distribution are captured by the TCNN model and the deduction error is 2.037 MPa. Therefore, the presented model is reliable to obtain the overall mechanical state of the coalmine roof, and it is supposed to promote the application of DL in underground construction.
更多查看译文
关键词
Underground construction,deep learning,monitoring,coalmine,mining-induced stress
AI 理解论文
溯源树
样例
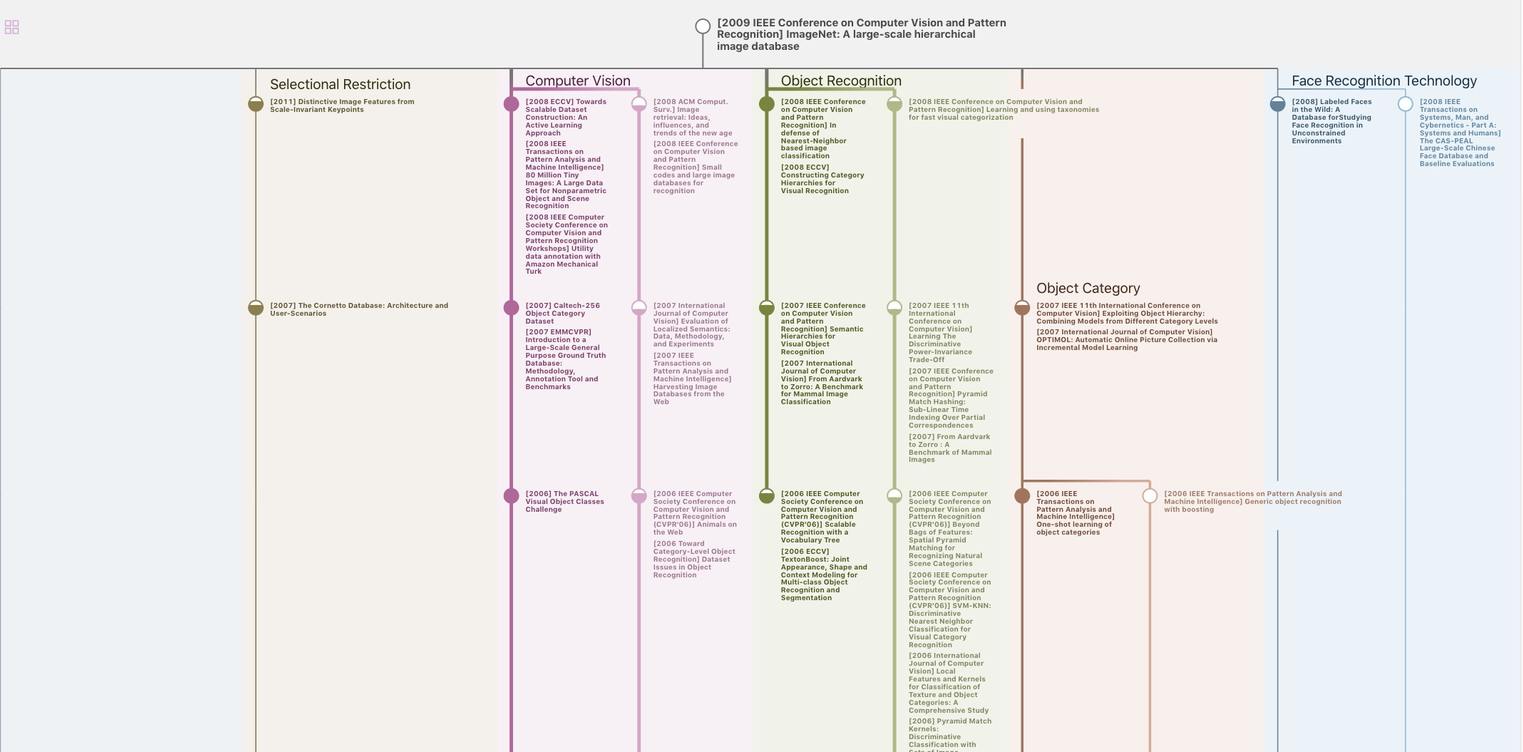
生成溯源树,研究论文发展脉络
Chat Paper
正在生成论文摘要