GRLC: Graph Representation Learning With Constraints
IEEE TRANSACTIONS ON NEURAL NETWORKS AND LEARNING SYSTEMS(2023)
摘要
Contrastive learning has been successfully applied in unsupervised representation learning. However, the generalization ability of representation learning is limited by the fact that the loss of downstream tasks (e.g., classification) is rarely taken into account while designing contrastive methods. In this article, we propose a new contrastive-based unsupervised graph representation learning (UGRL) framework by 1) maximizing the mutual information (MI) between the semantic information and the structural information of the data and 2) designing three constraints to simultaneously consider the downstream tasks and the representation learning. As a result, our proposed method outputs robust low-dimensional representations. Experimental results on 11 public datasets demonstrate that our proposed method is superior over recent state-of-the-art methods in terms of different downstream tasks. Our code is available at https://github.com/LarryUESTC/GRLC.
更多查看译文
关键词
Task analysis,Representation learning,Semantics,Self-supervised learning,Training,Mutual information,Computer science,Data mining,graph neural networks,graph representation learning,machine learning
AI 理解论文
溯源树
样例
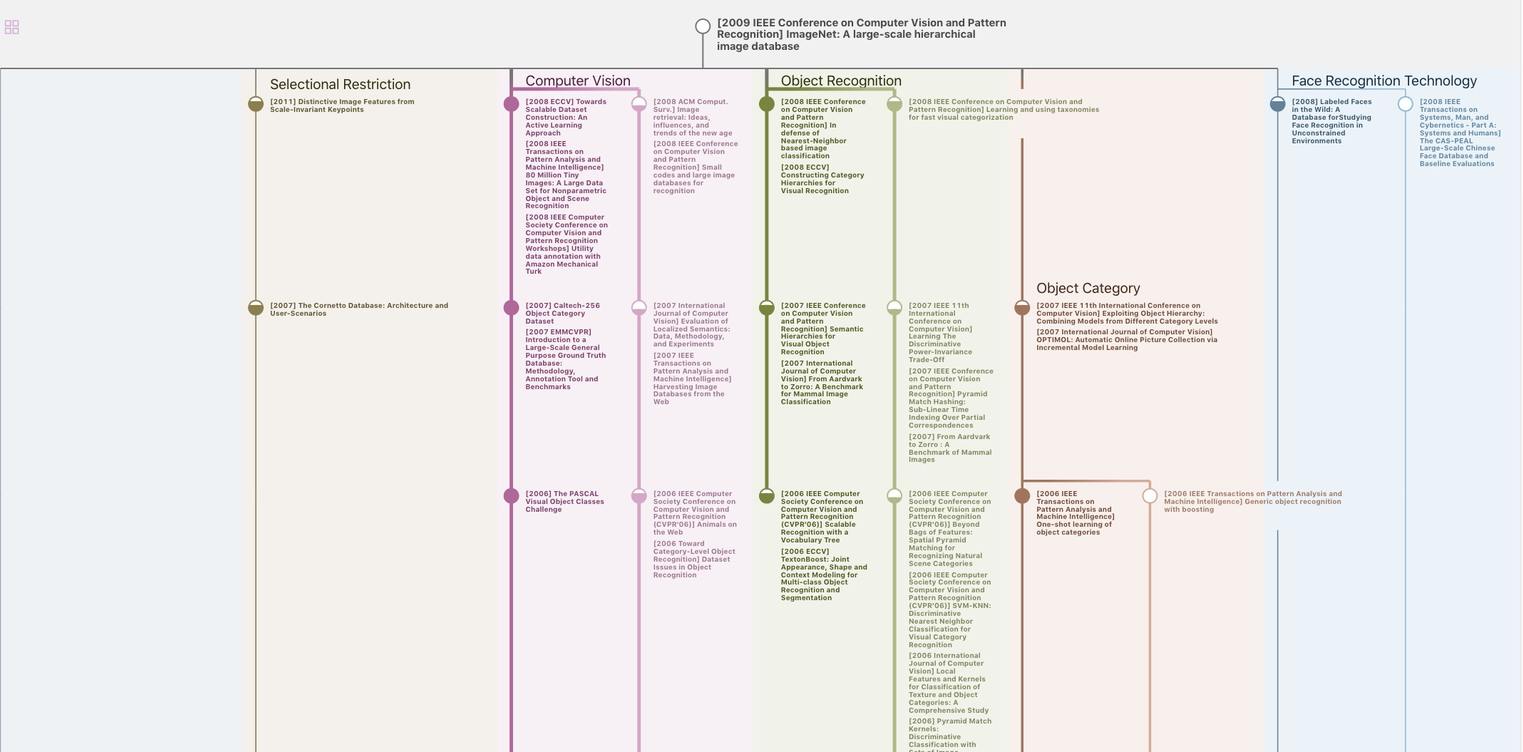
生成溯源树,研究论文发展脉络
Chat Paper
正在生成论文摘要