K-L Estimator: Dealing with Multicollinearity in the Logistic Regression Model
MATHEMATICS(2023)
摘要
Multicollinearity negatively affects the efficiency of the maximum likelihood estimator (MLE) in both the linear and generalized linear models. The Kibria and Lukman estimator (KLE) was developed as an alternative to the MLE to handle multicollinearity for the linear regression model. In this study, we proposed the Logistic Kibria-Lukman estimator (LKLE) to handle multicollinearity for the logistic regression model. We theoretically established the superiority condition of this new estimator over the MLE, the logistic ridge estimator (LRE), the logistic Liu estimator (LLE), the logistic Liu-type estimator (LLTE) and the logistic two-parameter estimator (LTPE) using the mean squared error criteria. The theoretical conditions were validated using a real-life dataset, and the results showed that the conditions were satisfied. Finally, a simulation and the real-life results showed that the new estimator outperformed the other considered estimators. However, the performance of the estimators was contingent on the adopted shrinkage parameter estimators.
更多查看译文
关键词
Kibria-Lukman estimator,logistic regression model,Liu estimator,multicollinearity,ridge regression estimator
AI 理解论文
溯源树
样例
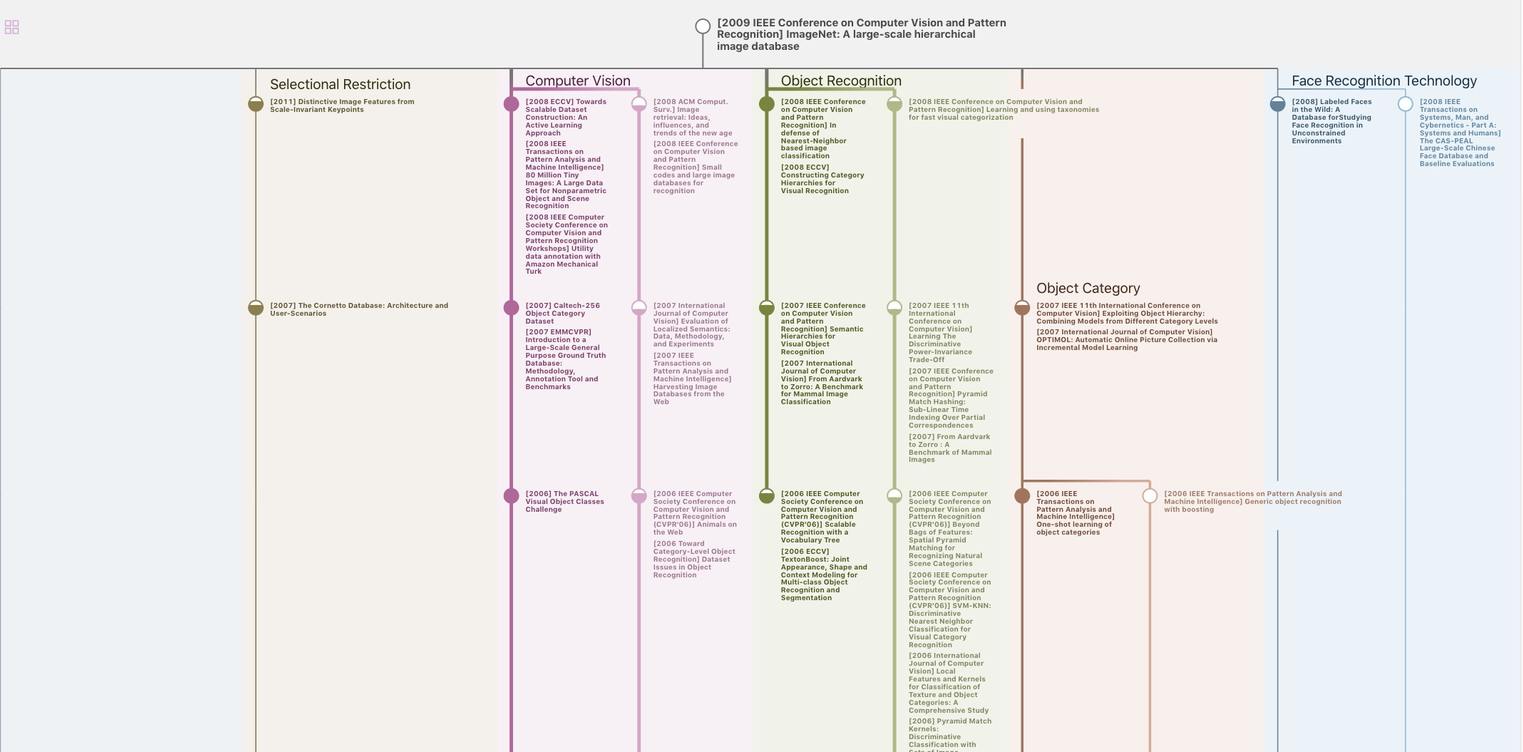
生成溯源树,研究论文发展脉络
Chat Paper
正在生成论文摘要