Deep reinforcement learning based research on low-carbon scheduling with distribution network schedulable resources
IET GENERATION TRANSMISSION & DISTRIBUTION(2023)
摘要
Reducing carbon emissions is a crucial way to achieve the goal of green and sustainable development. To accomplish this goal, electric vehicles (EVs) are considered system-schedulable energy storage devices, suppressing the negative impact of the randomness and fluctuation of renewable energy on the system's operation. In this paper, a coordination control strategy aimed at minimising the carbon emissions of a distribution network between EVs, energy storage devices, and static var compensators (SVCs) is proposed. A model-free deep reinforcement learning (DRL)-based approach is developed to learn the optimal control strategy with the constraint of avoiding system overload caused by random EV access. The twin-delayed deep deterministic policy gradient (TD3) framework is applied to design the learning method. After the model learning is completed, the neural network can quickly generate a real-time low-carbon scheduling strategy according to the system operating situation. Finally, simulation on the IEEE 33-bus system verifies the effectiveness and robustness of this method. On the premise of meeting the charging demand of electric vehicles, this method can optimise the system operation by controlling the charge-discharge process of EVs, effectively absorbing the renewable energy in the system and reducing the carbon emissions of the system operation.
更多查看译文
关键词
artificial intelligence,electric vehicles,energy conservation,energy storage
AI 理解论文
溯源树
样例
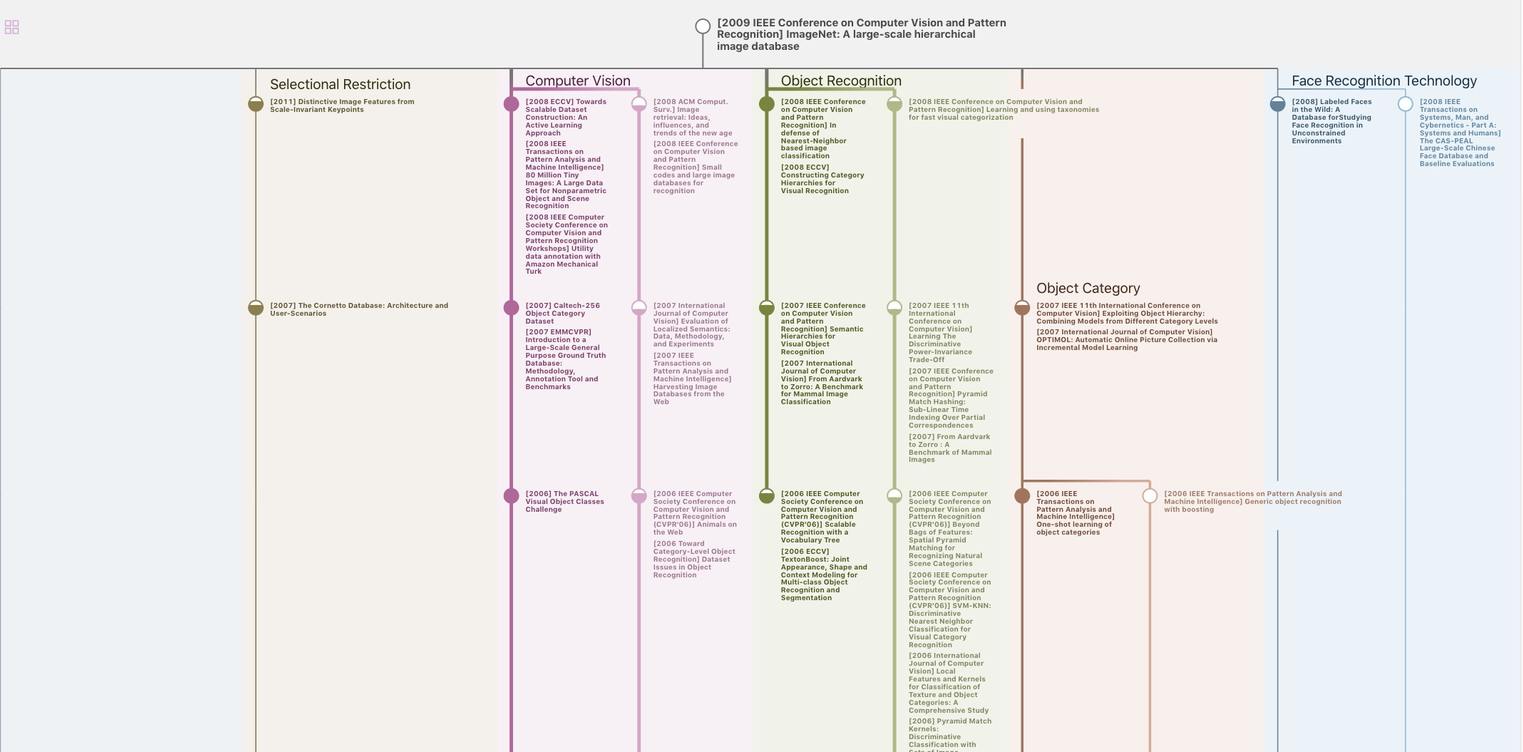
生成溯源树,研究论文发展脉络
Chat Paper
正在生成论文摘要