An automated multi-class skin lesion diagnosis by embedding local and global features of Dermoscopy images
MULTIMEDIA TOOLS AND APPLICATIONS(2023)
摘要
Skin cancer is an increasing cause of concern among cancers worldwide. There has been extensive research carried out all over the globe for the early detection of skin cancer to increase the life expectancy of patients. The decision support systems and Computer-aided diagnosis systems aid in detecting cancer at an early stage. The increasing ability of Convolutional Neural Networks (CNN) to extract delicate patterns has made it a popular choice in automated decision support systems. This work proposes a novel U-Net segmentation network with Spatial Attention Blocks (SPAB) called SASegNet to segment the skin lesion accurately. The spatial attention blocks emphasize the model to focus on a particular region. The proposed SASegNet model can provide an accuracy of 95% on the PH2 dataset. In this work, EfficientNet B1 is used for classification. The local features from segmentation results are then passed to EfficientNet B1 to extract features for classification. The pre-processed original images are passed to EfficientNet B1 to extract the global features. Finally, these two features are concatenated to extract the best patterns for classification. Experimentation is carried out on the International Skin Imaging Collaboration (ISIC) datasets. The proposed methodology can obtain the Area Under Curve Receiver Operating Characteristic Curve (AUC-ROC) as 0.974, 0.972, 0.962, and 0.937 for the ISIC-2017, 18, 19, and 2020 datasets. The results obtained are the benchmark results to the best of our knowledge. This automated methodology can aid practising dermatologists in a robust diagnosis.
更多查看译文
关键词
Skin lesion analysis,Spatial attention blocks,Segmentation,Classification,Deep learning,Dermoscopy
AI 理解论文
溯源树
样例
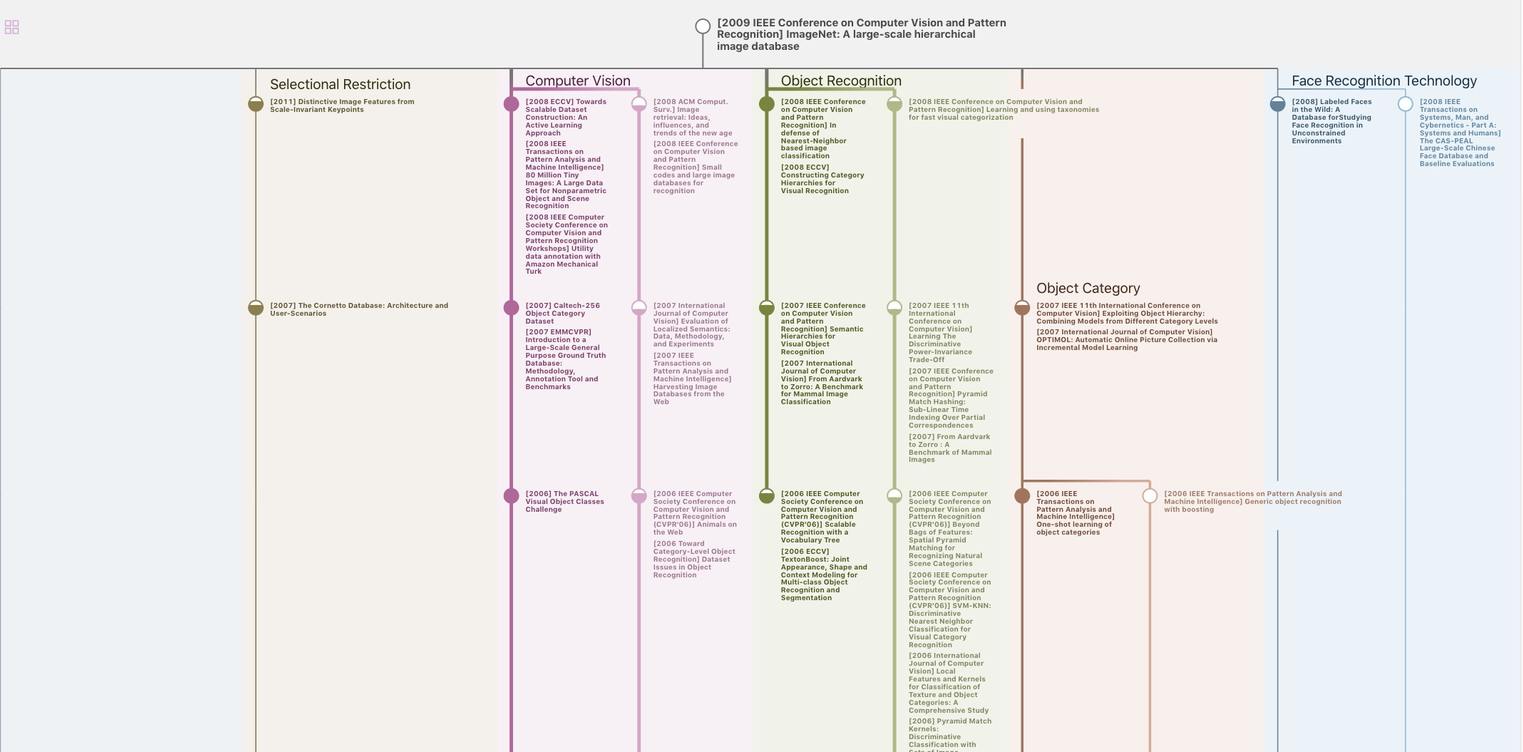
生成溯源树,研究论文发展脉络
Chat Paper
正在生成论文摘要