Noise2Clean: Cross-Device Side-Channel Traces Denoising with Unsupervised Deep Learning
ELECTRONICS(2023)
摘要
Deep learning (DL)-based side-channel analysis (SCA) has posed a severe challenge to the security and privacy of embedded devices. During its execution, an attacker exploits physical SCA leakages collected from profiling devices to create a DL model for recovering secret information from victim devices. Despite this success, recent works have demonstrated that certain countermeasures, such as random delay interrupts or clock jitters, would make these attacks more complex and less practical in real-world scenarios. To address this challenge, we present a novel denoising scheme that exploits the U-Net model to pre-process SCA traces for "noises" (i.e., countermeasures) removal. Specifically, we first pre-train the U-Net model on the paired noisy-clean profiling traces to obtain suitable parameters. This model is then fine-tuned on the noisy-only traces collected from the attacking device. The well-trained model will be finally deployed on the attacking device to remove the noises (i.e., countermeasures) from the measured power traces. In particular, a new inductive transfer learning method is also utilized in our scheme to transfer knowledge learned from the source domain (i.e., profiling device) to the target domain (i.e., attacking device) to improve the model's generalization ability. During our experimental evaluations, we conduct a detailed analysis of various countermeasures separately or combined and show that the proposed denoising model outperforms current state-of-the-art work by a large margin, e.g., a reduction of at least 30% in computation costs and 5x in guessing entropy.
更多查看译文
关键词
deep learning,side-channel analysis,denoising model
AI 理解论文
溯源树
样例
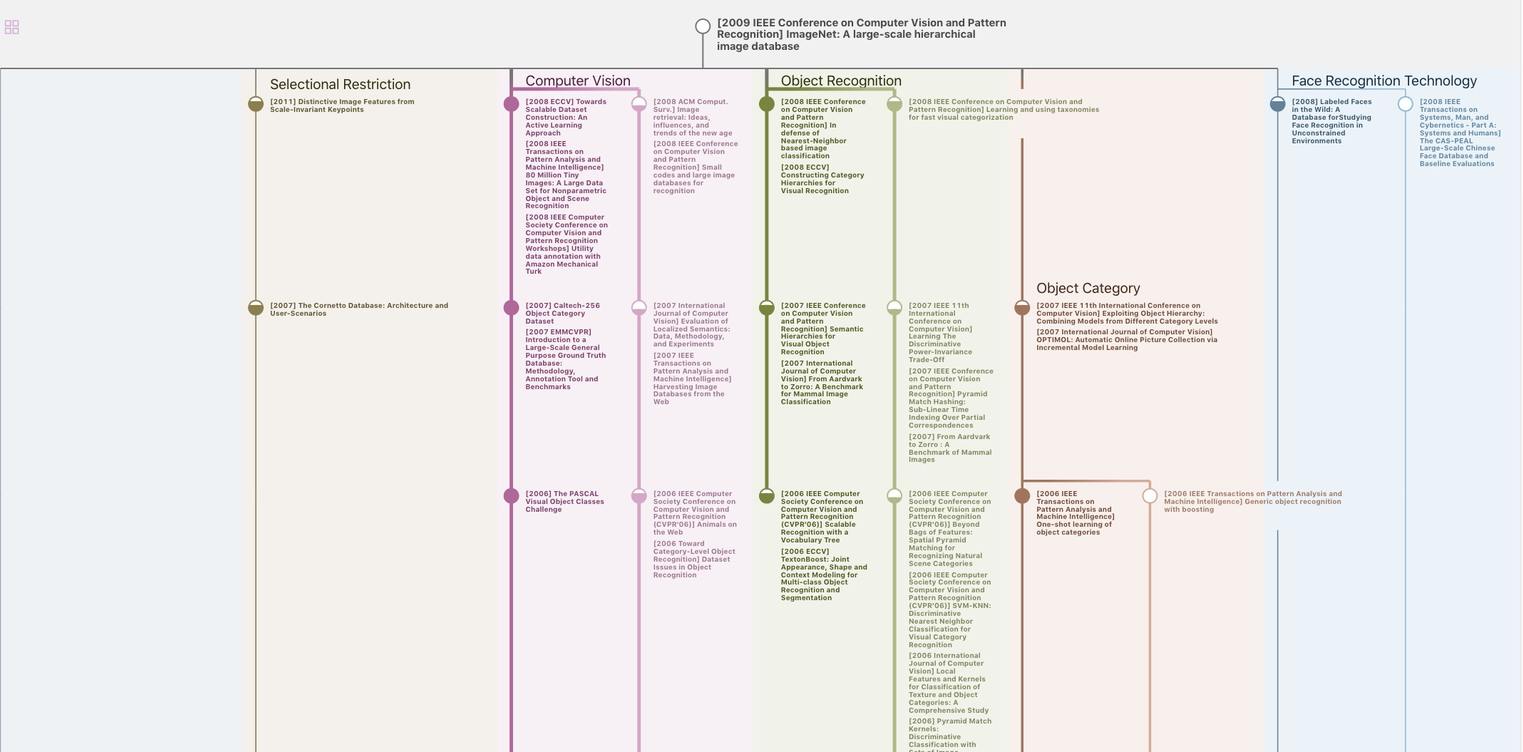
生成溯源树,研究论文发展脉络
Chat Paper
正在生成论文摘要