Two-Stage Decomposition Multi-Scale Nonlinear Ensemble Model with Error-Correction-Coupled Gaussian Process for Wind Speed Forecast
ATMOSPHERE(2023)
摘要
Wind power has great potential in the fields of electricity generation, heating, et cetera, and the precise forecasting of wind speed has become the key task in an effort to improve the efficiency of wind energy development. Nowadays, many existing studies have investigated wind speed prediction, but they often simply preprocess raw data and also ignore the nonlinear features in the residual part, which should be given special treatment for more accurate forecasting. Meanwhile, the mainstream in this field is point prediction which cannot show the potential uncertainty of predicted values. Therefore, this paper develops a two-stage decomposition ensemble interval prediction model. The original wind speed series is firstly decomposed using a complete ensemble empirical mode decomposition with adaptive noise (CEEMDAN) and the decomposed subseries with the highest approximate entropy is secondly decomposed through singular-spectrum analysis (SSA) to further reduce the complexity of the data. After two-stage decomposition, auto-encoder dimensionality reduction is employed to alleviate the accumulated error problem. Then, each reconstructed subsequence will generate an independent prediction result using an elastic neural network. Extreme gradient boosting (Xgboost) is utilized to integrate the separate predicted values and also carry out the error correction. Finally, the Gaussian process (GP) will generate the interval prediction result. The case study shows the best performance of the proposed models, not only in point prediction but also in interval prediction.
更多查看译文
关键词
two-stage decomposition,nonlinear ensemble,residual correction,interval forecast,wind speed prediction
AI 理解论文
溯源树
样例
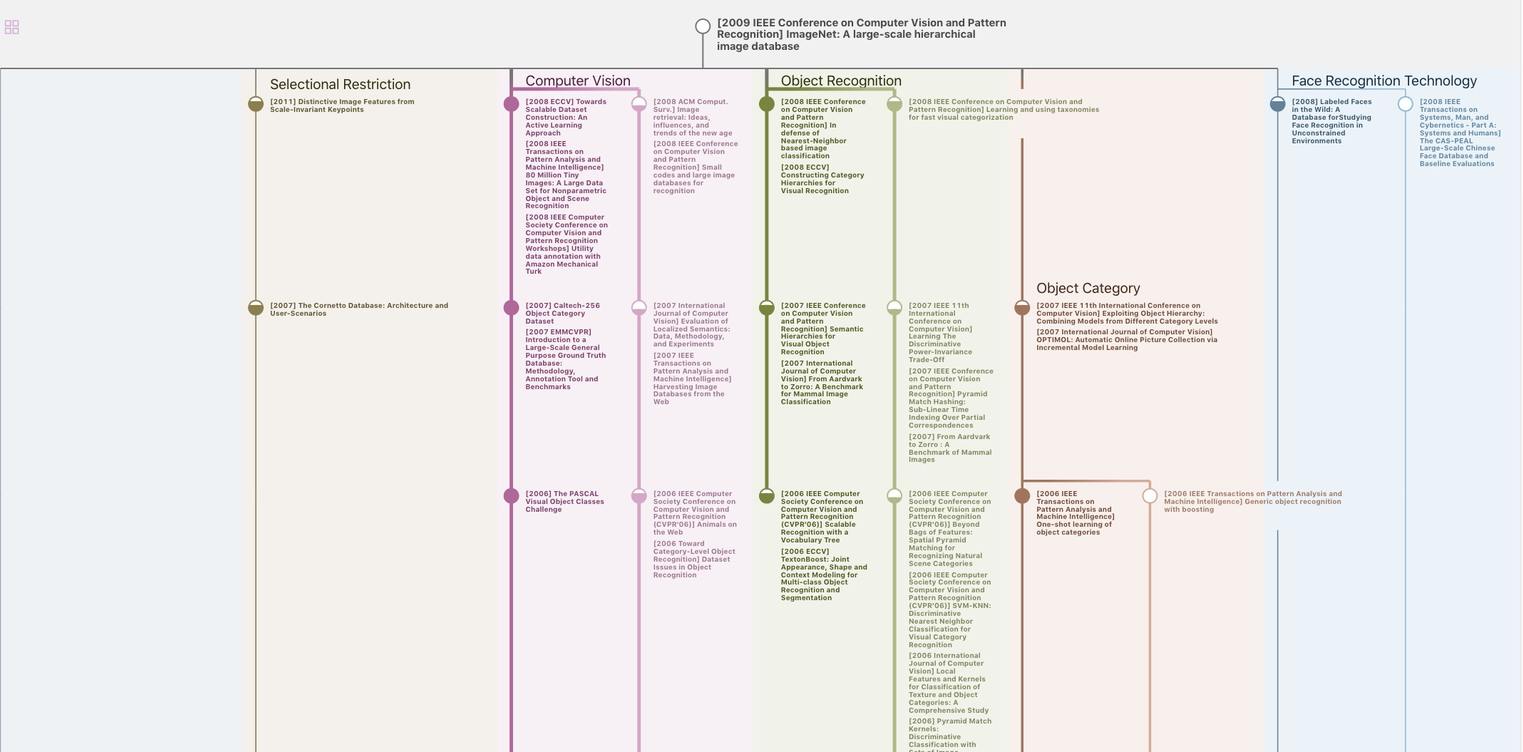
生成溯源树,研究论文发展脉络
Chat Paper
正在生成论文摘要