Exploring the PV Power Forecasting at Building Façades Using Gradient Boosting Methods
Energies(2023)
摘要
Solar power forecasting is of high interest in managing any power system based on solar energy. In the case of photovoltaic (PV) systems, and building integrated PV (BIPV) in particular, it may help to better operate the power grid and to manage the power load and storage. Power forecasting directly based on PV time series has some advantages over solar irradiance forecasting first and PV power modeling afterwards. In this paper, the power forecasting for BIPV systems in a vertical façade is studied using machine learning algorithms based on decision trees. The forecasting scheme employs the skforecast library from the Python environment, which facilitates the implementation of different schemes for both deterministic and probabilistic forecasting applications. Firstly, deterministic forecasting of hourly BIPV power was performed with XGBoost and Random Forest algorithms for different cases, showing an improvement in forecasting accuracy when some exogenous variables were used. Secondly, probabilistic forecasting was performed with XGBoost combined with the Bootstrap method. The results of this paper show the capabilities of Random Forest and gradient boosting algorithms, such as XGBoost, to work as regressors in time series forecasting of BIPV power. Mean absolute error in the deterministic forecast, using the most influencing exogenous variables, were around 40% and close below 30% for the south and east array, respectively.
更多查看译文
关键词
BIPV,PV power forecasting,machine learning,gradient boosting algorithms
AI 理解论文
溯源树
样例
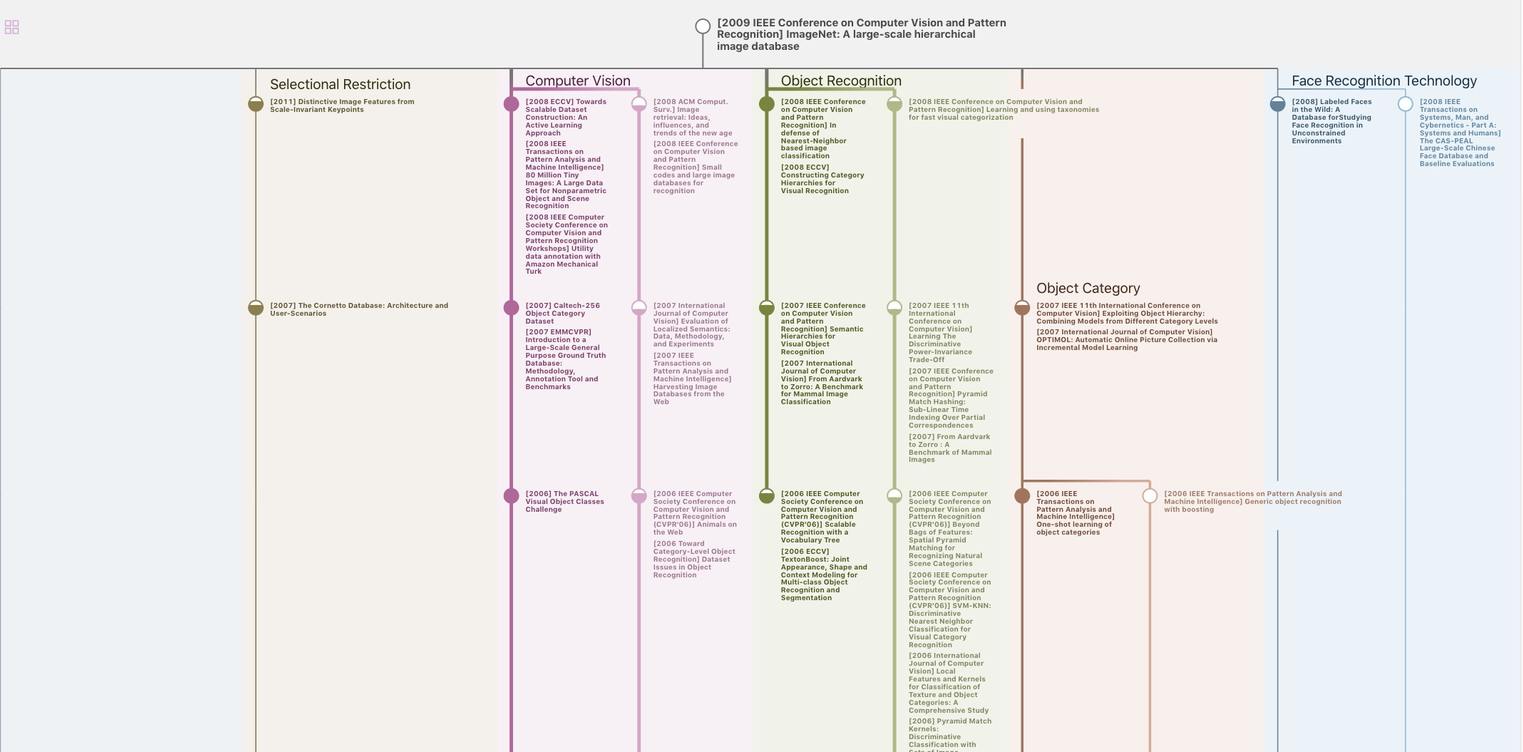
生成溯源树,研究论文发展脉络
Chat Paper
正在生成论文摘要