Participatory Sensing Based Urban Road Condition Classification using Transfer Learning
MOBILE NETWORKS & APPLICATIONS(2023)
摘要
Road surfaces can contain a lot of anomalies such as potholes. Understanding and detecting such irregularities are a key towards building a safe road transport system and a step towards building a smart city. This paper makes an attempt to classify road surface images using transfer learning on pre-trained convolution neural network (CNN) based models. In this work, two types of transfer learning approaches namely fine tuning and feature extraction are employed on various state-of-the-art CNN architectures of recent past such as VGG-16 (by Visual Geometry Group), VGG-19, Inception, ResNet-50 (Residual Network), Xception, DenseNet-121, SEResNet-50 (Squeeze-and-Excitation ResNet model), and EfficientNet-B3. The performance of the models are evaluated based on real-world dataset of road surface images collected by the participants. The evaluation metrics considered are test accuracy, precision, recall, F1-score, specificity, area under the receiver operating characteristic curve (ROC-AUC) and also training and inference time measurements. It is observed that among all the deep CNN models, SEResNet-50 (F1-score = 0.954, ROC-AUC = 0.993), Xception (F1-score = 0.943, ROC-AUC = 0.987) and DenseNet-121 (F1-score = 0.941, ROC-AUC = 0.987) achieve the best performance in terms of the evaluation metrics while fine tuning is considered with data augmentation. Effectiveness of the deep CNN models are also evaluated on various benchmark datasets, namely Road Traversing Knowledge (RTK) dataset and pothole dataset.
更多查看译文
关键词
Deep learning,Convolutional neural networks,Transfer learning,Participatory sensing
AI 理解论文
溯源树
样例
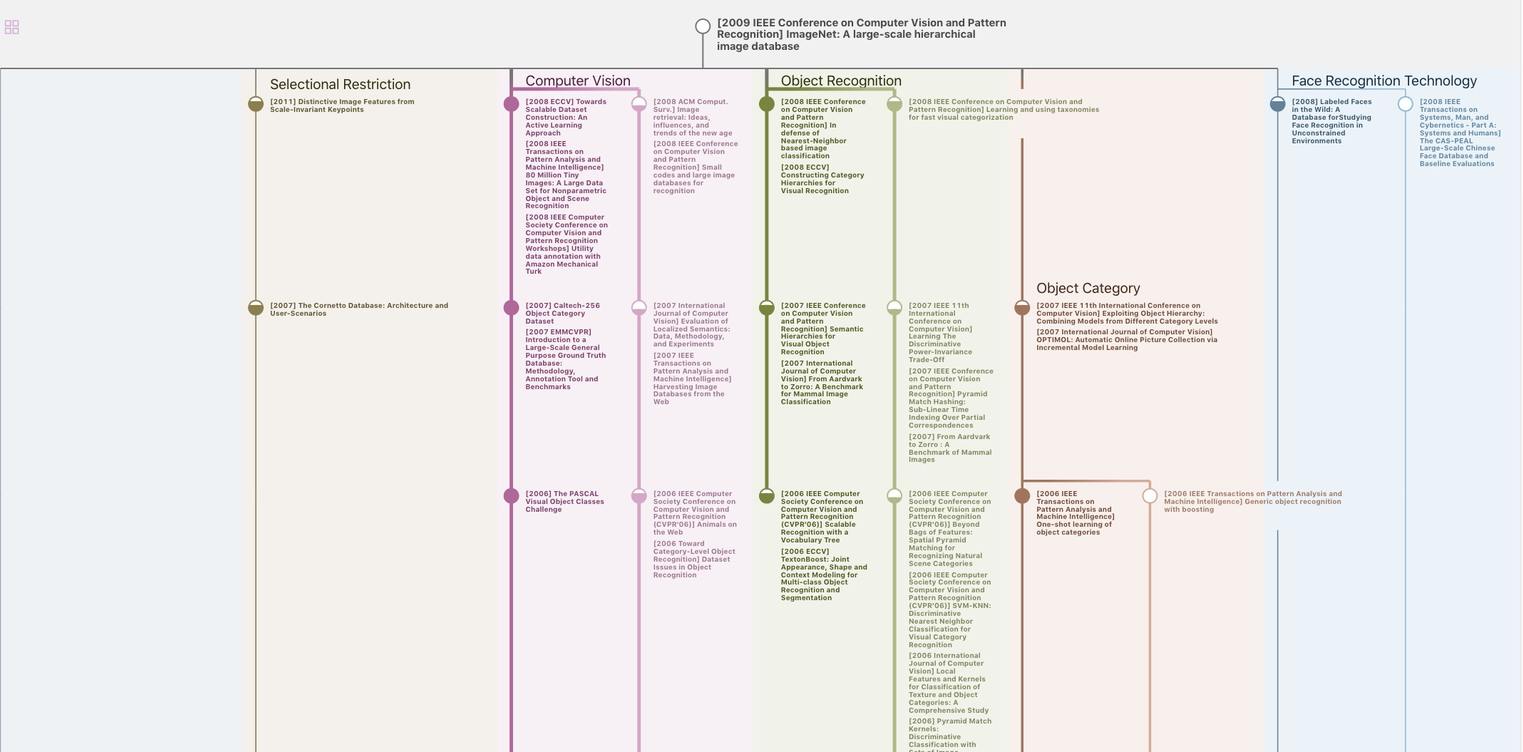
生成溯源树,研究论文发展脉络
Chat Paper
正在生成论文摘要