Exploring the Capabilities of a Lightweight CNN Model in Accurately Identifying Renal Abnormalities: Cysts, Stones, and Tumors, Using LIME and SHAP
APPLIED SCIENCES-BASEL(2023)
摘要
Kidney abnormality is one of the major concerns in modern society, and it affects millions of people around the world. To diagnose different abnormalities in human kidneys, a narrow-beam x-ray imaging procedure, computed tomography, is used, which creates cross-sectional slices of the kidneys. Several deep-learning models have been successfully applied to computer tomography images for classification and segmentation purposes. However, it has been difficult for clinicians to interpret the model's specific decisions and, thus, creating a "black box" system. Additionally, it has been difficult to integrate complex deep-learning models for internet-of-medical-things devices due to demanding training parameters and memory-resource cost. To overcome these issues, this study proposed (1) a lightweight customized convolutional neural network to detect kidney cysts, stones, and tumors and (2) understandable AI Shapely values based on the Shapley additive explanation and predictive results based on the local interpretable model-agnostic explanations to illustrate the deep-learning model. The proposed CNN model performed better than other state-of-the-art methods and obtained an accuracy of 99.52 +/- 0.84% for K = 10-fold of stratified sampling. With improved results and better interpretive power, the proposed work provides clinicians with conclusive and understandable results.
更多查看译文
关键词
convolutional neural network,deep learning,kidney abnormalities,lightweight model,explainable artificial intelligence
AI 理解论文
溯源树
样例
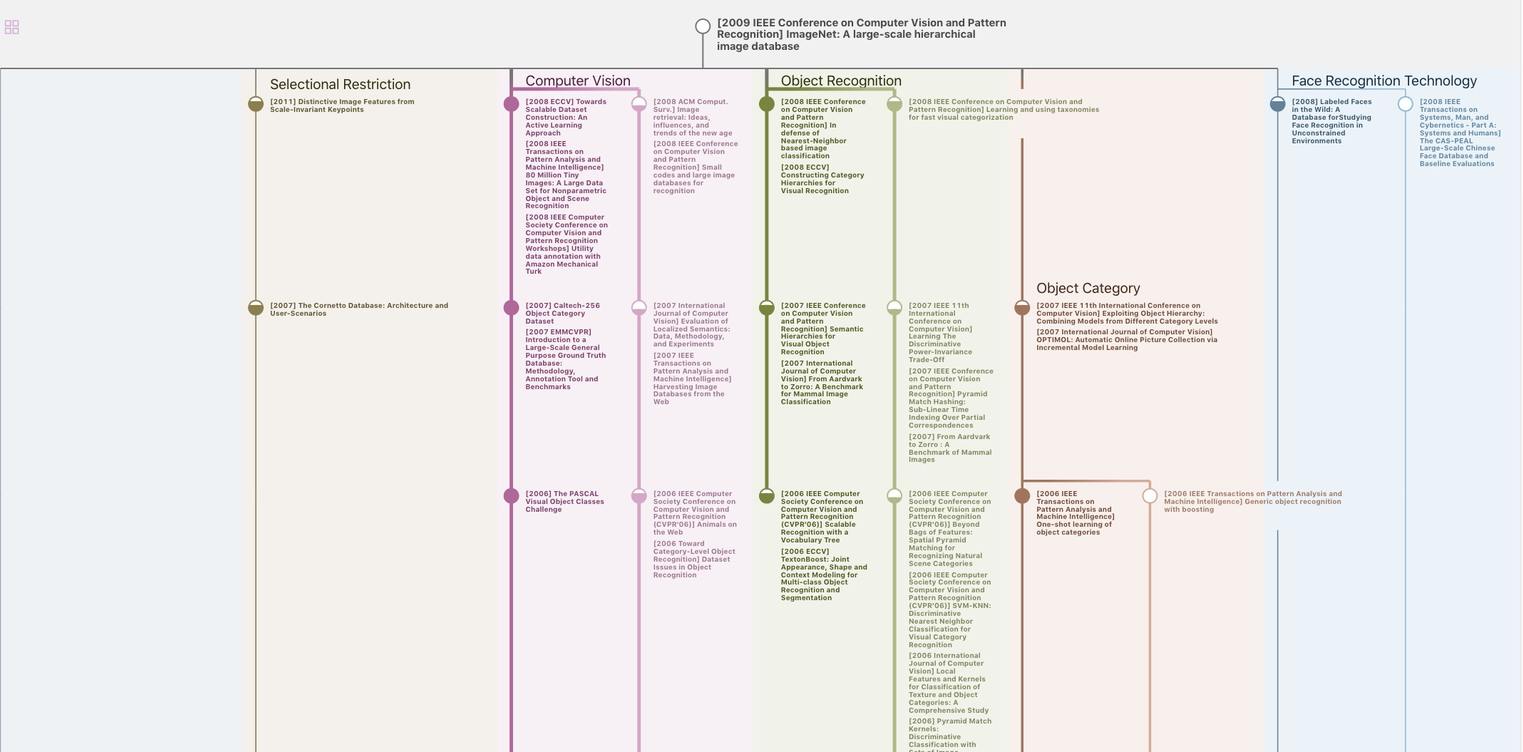
生成溯源树,研究论文发展脉络
Chat Paper
正在生成论文摘要