IGJO: An Improved Golden Jackel Optimization Algorithm Using Local Escaping Operator for Feature Selection Problems
NEURAL PROCESSING LETTERS(2023)
摘要
Feature Selection (FS) is an essential process that is implicated in data mining and machine learning for data preparation by removing redundant and irrelevant features, thereby falling the possible risk associated with the expletive of dimensionality caused by the large dataset. As a result, FS is thought to be a combinatorial NP-hard problem, which refers to a situation where the computation time increases as the problem dimension increases. Recently, researchers have focused on metaheuristic algorithms to perform this task. Therefore, this paper proposes an effective metaheuristic which is a new variant of the recently reported Golden Jackel Optimization (GJO) algorithm called Improved GJO (IGJO). The basic GJO algorithm suffers from a local optima trap when handling large dimensional feature selection problems. Therefore, the effectiveness of the GJO is improved by considering the operators from the gradient-based optimizer. The proposed IGJO is based on the local escaping operator and the direction of population movement to improve the exploration and exploitation ability of the basic GJO algorithm. The superiority of the IGJO algorithm is tested on 23 standard numerical benchmark problems, 29 CEC2017 optimization problems, and 33 CEC2020 constrained real-world engineering design problems. Additionally, the IGJO is transformed to its binary version for the FS problem using a new nonlinear time-varying sigmoid transfer function, and finally, the binary variant is validated on FS problems with different benchmark datasets. The performance of the IGJO is compared with well-known algorithms to validate its superiority. The obtained results show that the IGJO is a reliable tool for numerical optimization problems and FS problems.
更多查看译文
关键词
Feature selection,Golden Jackel optimization,Machine learning,Metaheuristics,Numerical optimization
AI 理解论文
溯源树
样例
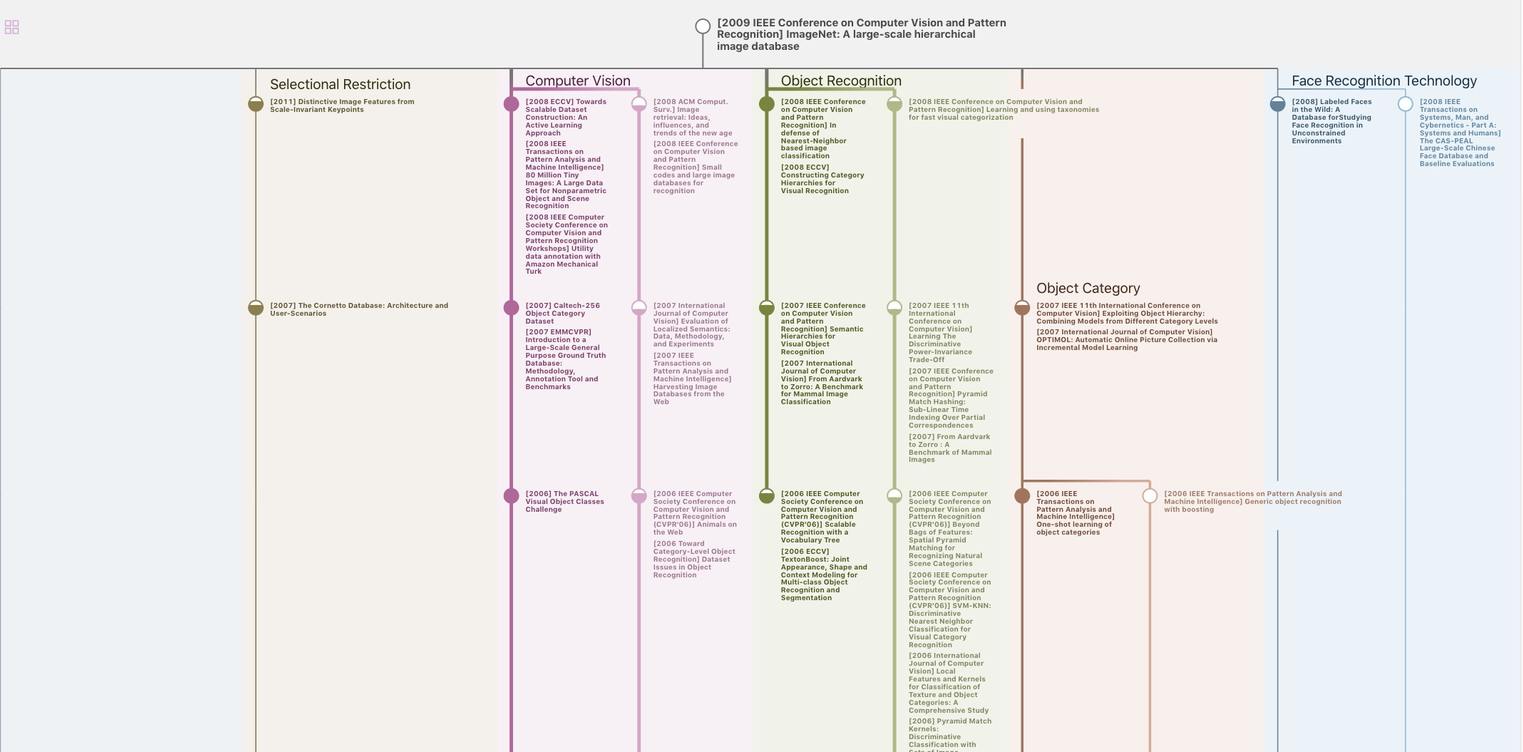
生成溯源树,研究论文发展脉络
Chat Paper
正在生成论文摘要