Efficient computational design of two-dimensional van der Waals heterostructures: Band alignment, lattice mismatch, and machine learning
PHYSICAL REVIEW MATERIALS(2023)
摘要
We develop a computational database, website applications (web-apps), and machine-learning (ML) models to accelerate the design and discovery of two-dimensional (2D) heterostructures. Using density functional theory (DFT) based lattice parameters and electronic band energies for 674 nonmetallic exfoliable 2D materials, we generate 226 779 possible bilayer heterostructures. We classify these heterostructures into type-I, -II, and -III systems according to Anderson's rule, which is based on the relative band alignments of the noninteracting monolayers. We find that type II is the most common and type III the least common heterostructure type. We subsequently analyze the chemical trends for each heterostructure type in terms of the Periodic Table of constituent elements. The band alignment data can also be used for identifying photocatalysts and high-work-function 2D metals for contacts. We validate our results by comparing them to experimental data as well as hybrid-functional predictions. Additionally, we carry out DFT calculations of a few selected systems (MoS2/WSe2, MoS2/h-BN, and MoSe2/CrI3), to compare the band-alignment description with the predictions from Anderson's rule. We develop web-apps to enable users to virtually create combinations of 2D materials and predict their properties. Additionally, we use ML tools to predict band-alignment information for 2D materials. The web-apps, tools, and associated data will be distributed through the JARVIS-HETEROSTRUCTURE website. Our analysis, results, and the developed web-apps can be applied to the screening and design applications, such as finding alternative photocatalysts, photodetectors, and high-work-function (WF) 2D-metal contacts.
更多查看译文
关键词
band alignment,lattice mismatch,two-dimensional
AI 理解论文
溯源树
样例
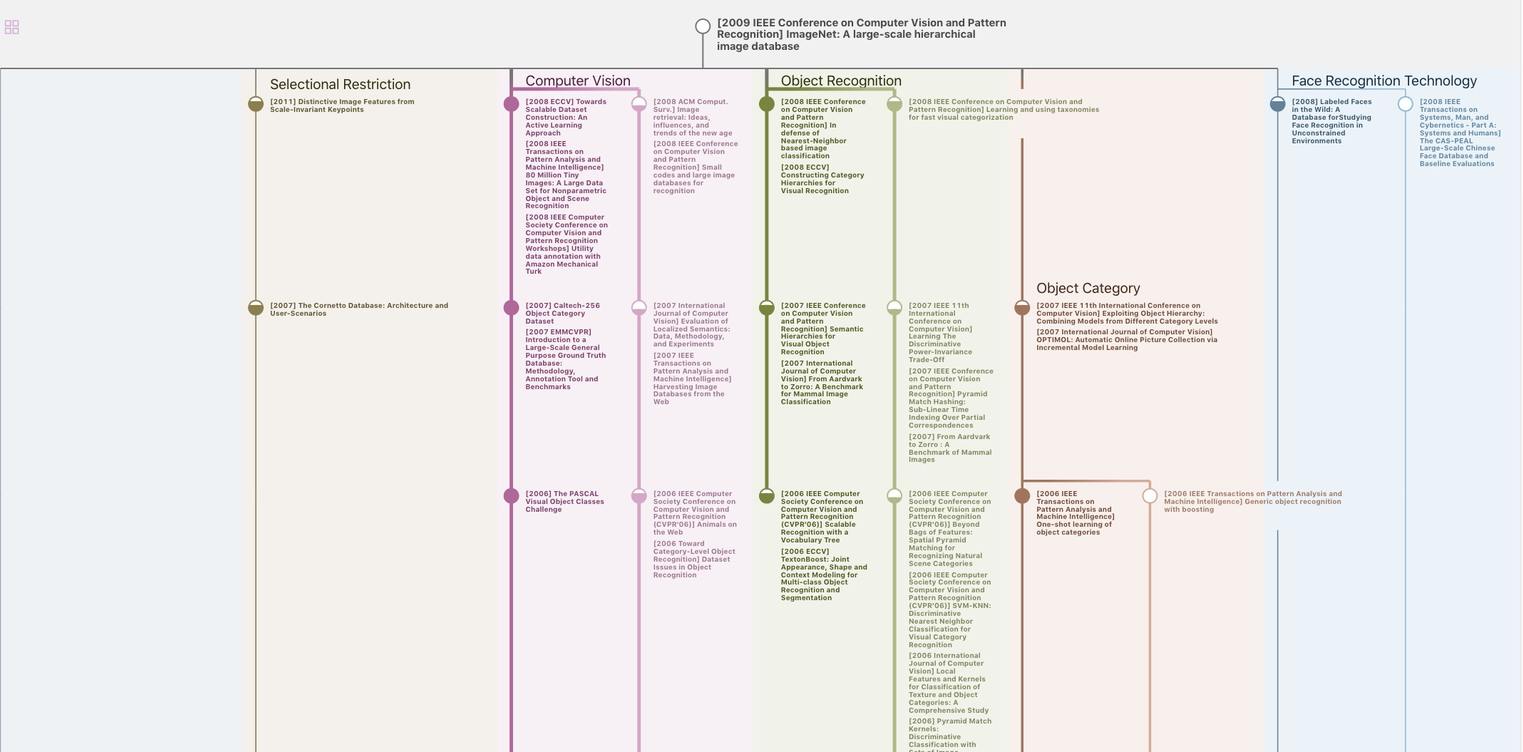
生成溯源树,研究论文发展脉络
Chat Paper
正在生成论文摘要