ASALD: Adaptive Sparse Augmented Lagrangian Deblurring of Underwater Images with Optical Priori
Imaging science journal/The imaging science journal(2022)
摘要
Owing to absorption, reflection, diffraction and, deplorable conditions, the capturing of underwater images present more challenges for the consumer. The proposed work focuses on Enhanced Augmented Lagrangian for image deblurring with additional performance-enhancing optical and sparse derivative prior to model the underwater imaging. The proposed method using Augmented Lagragian with Optical and Sparse Derivative prior is novel in the following ways: (i) usage of optical prior modeled after underwater imaging conditions, taking into account deformations and distortions that accompany the capture of underwater images (ii) sparse derivative prior helps to optimize fast convergence through regularization and faster computation with better edge preservation; (iii) the deblurring begins with the sparsest derivative beforehand and the final deblurred result achieving good dB improvements through sparse regulation. The weights with penalty and regularization ensure that each iteration through steep descent reaches a global minimum. The proposed algorithm performs better as compared to state-of-the-art approaches.
更多查看译文
关键词
Optical prior,point spread function,augmented lagrangian,penalty function,sparsity regularization,iterative minimization,derivative prior,steepest descent
AI 理解论文
溯源树
样例
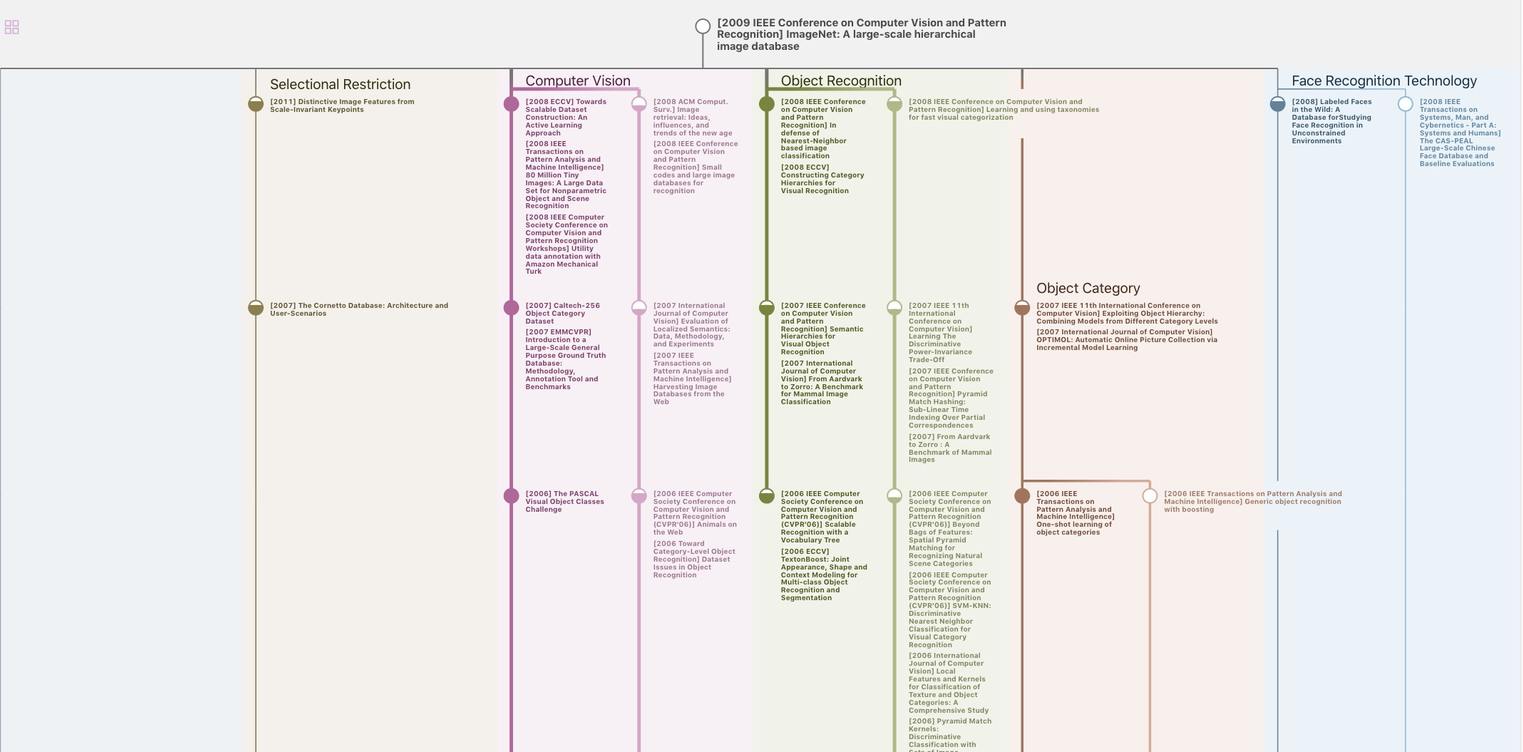
生成溯源树,研究论文发展脉络
Chat Paper
正在生成论文摘要