Deep Learning Model Ensemble for the Accuracy of Classification Degenerative Arthritis
CMC-COMPUTERS MATERIALS & CONTINUA(2023)
摘要
Artificial intelligence technologies are being studied to provide scientific evidence in the medical field and developed for use as diagnos-tic tools. This study focused on deep learning models to classify degen-erative arthritis into Kellgren-Lawrence grades. Specifically, degenerative arthritis was assessed by X-ray radiographic images and classified into five classes. Subsequently, the use of various deep learning models was investigated for automating the degenerative arthritis classification process. Although research on the classification of osteoarthritis using deep learning has been conducted in previous studies, only local models have been used, and an ensemble of deep learning models has never been applied to obtain more accurate results. To address this issue, this study compared the classification performance of deep learning models, including VGGNet, DenseNet, ResNet, TinyNet, EfficientNet, MobileNet, Xception, and ViT, on a dataset com-monly used for osteoarthritis classification tasks. Our experimental results verified that even without applying a separate methodology, the performance of the ensemble was comparable to that of existing studies that only used the latest deep learning model and changed the learning method. From the trained models, two ensembles were created and evaluated: weight and specialist. The weight ensemble showed an improvement in accuracy of 1%, and the proposed specialist ensemble improved accuracy, precision, recall, and F1 score by 5%, 6%, 6%, and 6%, respectively, compared with the results of prior studies.
更多查看译文
关键词
Knee osteoarthritis,deep learning,convolutional neural network,Kellgren-Lawrence grade,classification,knee X-ray
AI 理解论文
溯源树
样例
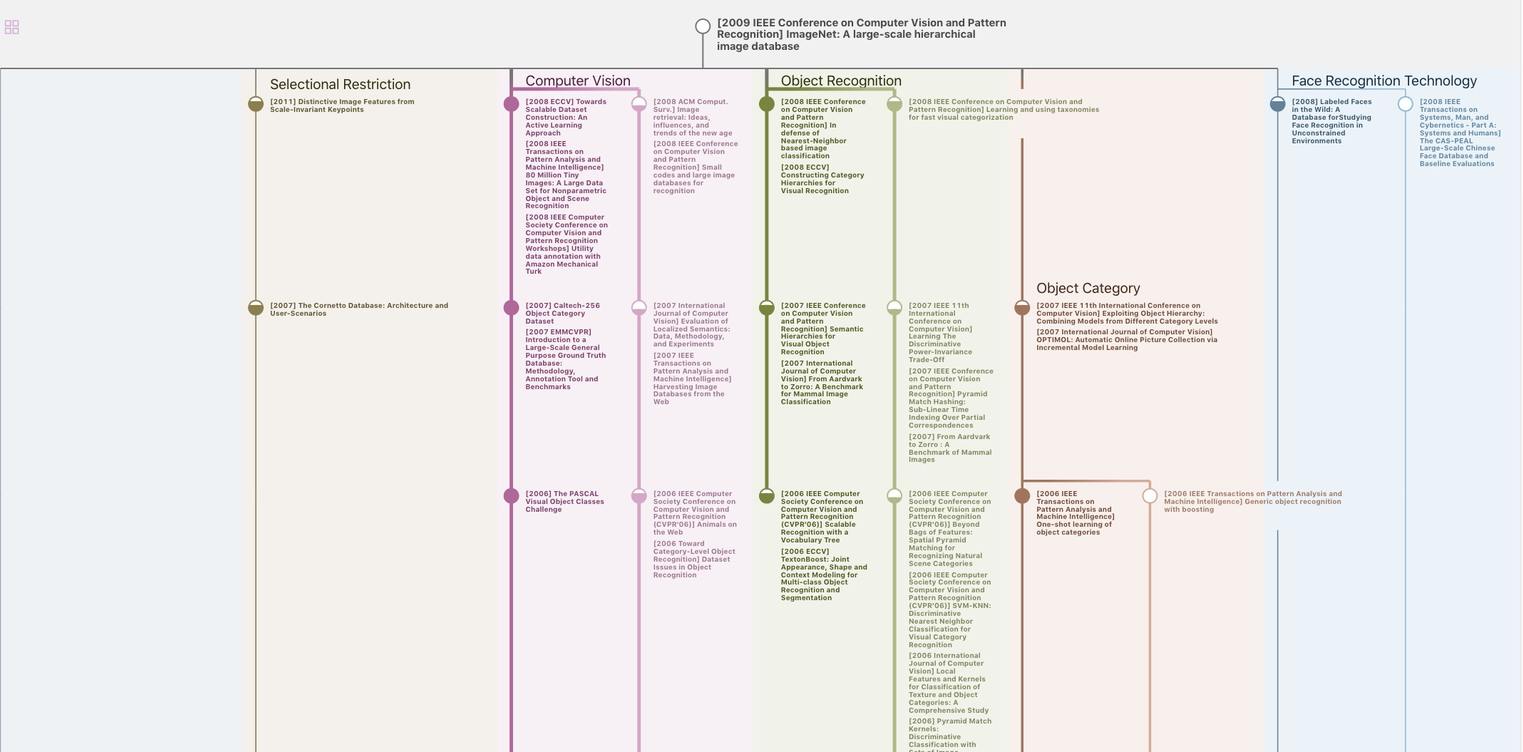
生成溯源树,研究论文发展脉络
Chat Paper
正在生成论文摘要