Quantifying Model Selection Uncertainty Via Bootstrapping and Akaike Weights
International journal of consumer studies(2023)
摘要
Picking one 'winner' model for researching a certain phenomenon while discarding the rest implies a confidence that may misrepresent the evidence. Multimodel inference allows researchers to more accurately represent their uncertainty about which model is 'best'. But multimodel inference, with Akaike weights-weights reflecting the relative probability of each candidate model-and bootstrapping, can also be used to quantify model selection uncertainty, in the form of empirical variation in parameter estimates across models, while minimizing bias from dubious assumptions. This paper describes this approach. Results from a simulation example and an empirical study on the impact of perceived brand environmental responsibility on customer loyalty illustrate and provide support for our proposed approach.
更多查看译文
关键词
Akaike weights,bootstrapping,information criteria,model selection,uncertainty
AI 理解论文
溯源树
样例
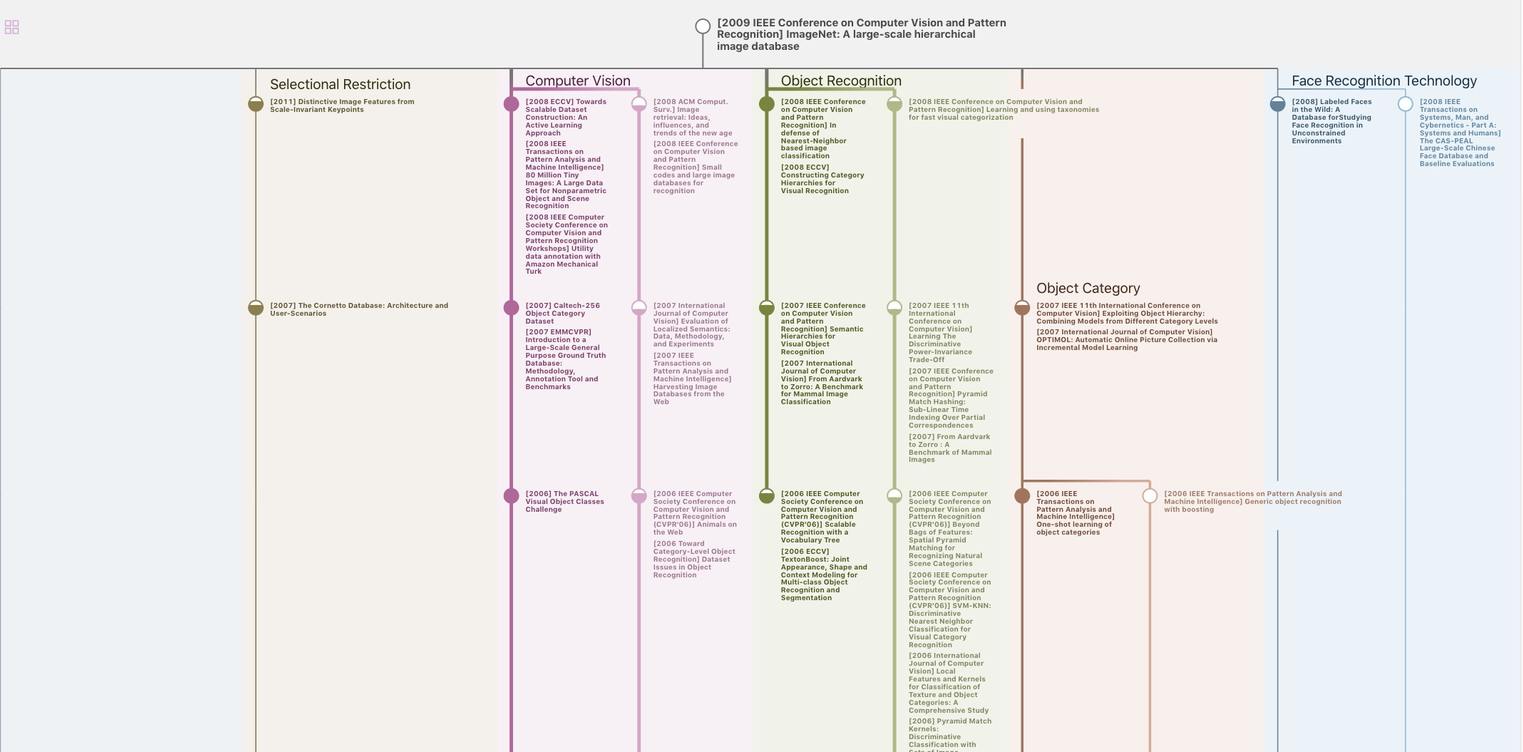
生成溯源树,研究论文发展脉络
Chat Paper
正在生成论文摘要