GC-TripRec: Graph contextualized generative network with adversarial learning for trip recommendation
WORLD WIDE WEB-INTERNET AND WEB INFORMATION SYSTEMS(2023)
摘要
Trip recommendation, which aims to recommend a sequence of point-of-interests (POIs) as a trip to visit, is of great importance to location-based service systems. However, due to data sparsity, existing methods lack the ability to capture complex POI correlations in trips, resulting in limited recommendation performance. In this paper, we propose a graph contextualized trip recommendation model GC-TripRec with adversarial learning to take advantage of graph-based representation learning, so that enhanced trip recommendation can be supported by capturing essential knowledge about complex POI correlations in trips. Specifically, it first models pairwise transition relationships by a global transition graph, upon which global-level POI embedding can be captured by a graph convolution network. We further generate trajectory contextualized POI representation via a trip-level embedding method that compromises transition-based and category-based information of POIs in a single trip. In addition, trip representation is learned through Long Short-Term Memory (LSTM) with an adversarial learning task, such that query representation space would be highly overlapped with trip representation space, enabling getting an excellent trip recommendation system. Comprehensive experiments on four real datasets demonstrate that our proposed GC-TripRec significantly outperforms state-of-the-art methods.
更多查看译文
关键词
Trip recommendation,Graph neural network,Representation learning,Adversarial learning
AI 理解论文
溯源树
样例
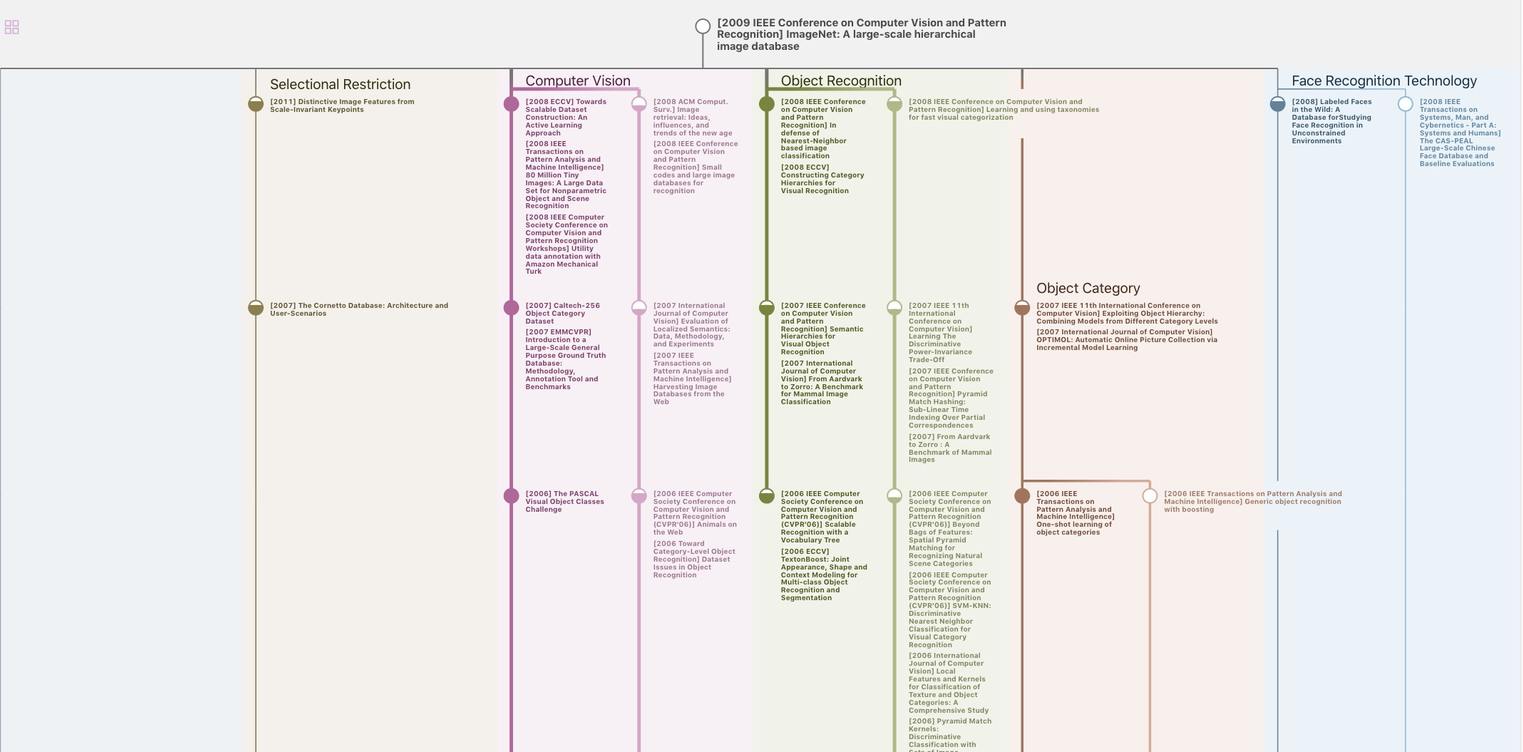
生成溯源树,研究论文发展脉络
Chat Paper
正在生成论文摘要