Knowledge Graph-Based Reinforcement Federated Learning for Chinese Question and Answering
IEEE TRANSACTIONS ON COMPUTATIONAL SOCIAL SYSTEMS(2024)
摘要
Knowledge question and answering (Q & A) is widely used. However, most existing semantic parsing methods in Q & A usually use cascading, which can incur error accumulation. In addition, using only one institution's Q & A data definitely will limit the Q & A performance, while data privacy prevents sharing between institutions. This article proposes a knowledge graph-based reinforcement federated learning (KGRFL)-based Q & A approach to address these challenges. We design an end-to-end multitask semantic parsing model MSP-bidirectional and auto-regressive transformers (BART) that identifies question categories while converting questions into SPARQL statements to improve semantic parsing. Meanwhile, a reinforcement learning (RL)-based model fusion strategy is proposed to improve the effectiveness of federated learning, which enables multi-institution joint modeling and data privacy protection using cross-domain knowledge. In particular, it also reduces the negative impact of low-quality clients on the global model. Furthermore, a prompt learning-based entity disambiguation method is proposed to address the semantic ambiguity problem because of joint modeling. The experiments show that the proposed method performs well on different datasets. The Q & A results of the proposed approach outperform the approach of using only a single institution. Experiments also demonstrate that the proposed approach is resilient to security attacks, which is required for real applications.
更多查看译文
关键词
Data models,Semantics,Federated learning,Knowledge graphs,Data privacy,Transformers,Task analysis,Knowledge graph,multitask semantic parsing MSP-bidirectional and auto-regressive transformers (BART),prompt learning,question and answering (Q&A),reinforcement federated learning (RFL)
AI 理解论文
溯源树
样例
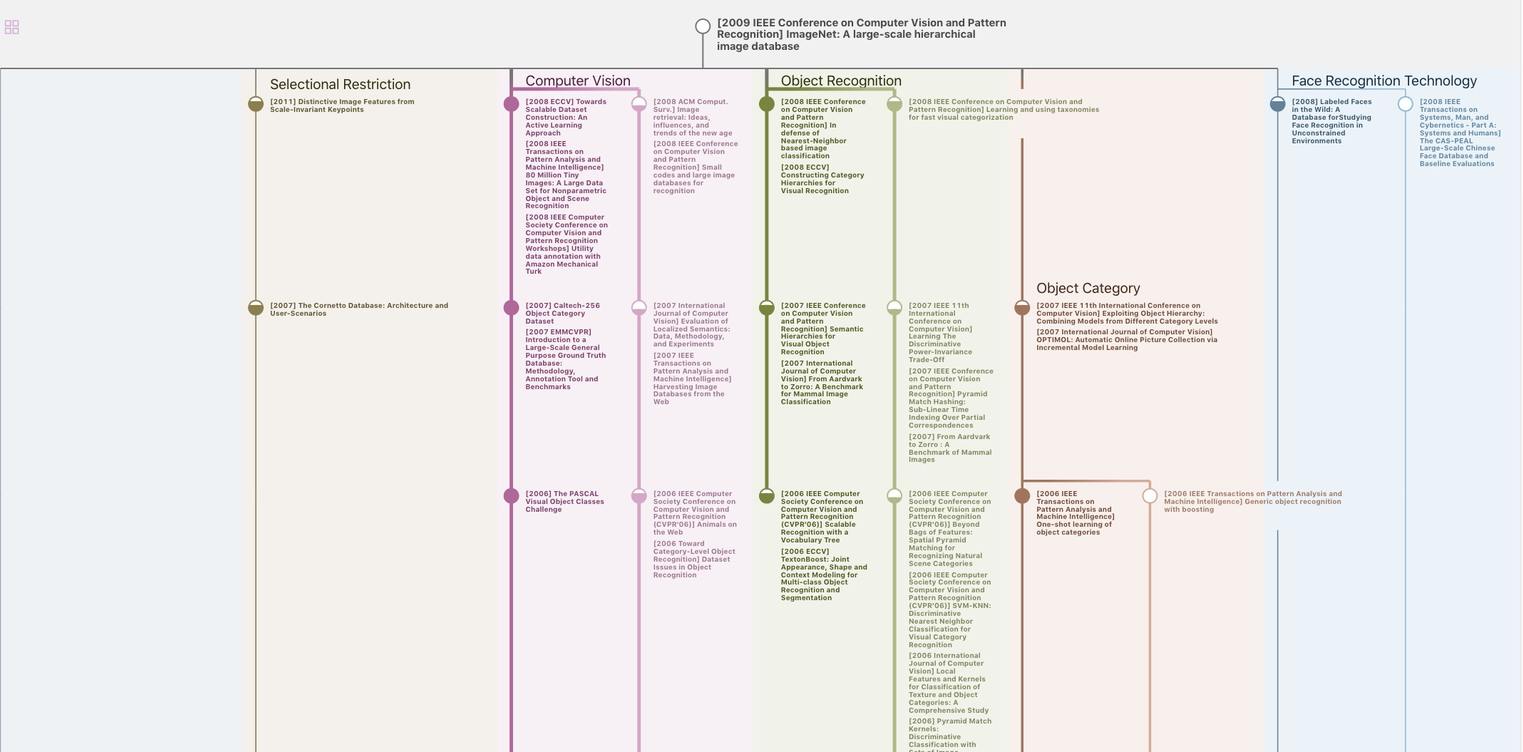
生成溯源树,研究论文发展脉络
Chat Paper
正在生成论文摘要