An enhanced decomposition-based multiobjective evolutionary algorithm with adaptive neighborhood operator and extended distance-based environmental selection
JOURNAL OF SUPERCOMPUTING(2023)
摘要
The multiobjective evolutionary algorithm based on decomposition (MOEA/D) proposed in 2007 has shown to be effective in solving multiobjective optimization problems. However, the fixed neighborhood size prevents MOEA/D series algorithm from effective exploring and exploiting. Meanwhile, since the newly generated offspring can replace the parent solutions in the neighborhood without any constraints, the current solutions of multiple subproblems may converge to a certain region or even a certain point. Obviously, the population diversity is significantly deteriorate. To alleviate these problems, this paper proposes an enhanced MOEA/D with adaptive neighborhood operator and extended distance-based environmental selection (MOEA/D-ANED) to solve many-objective optimization problems (MaOPs). The basic idea is that the adaptive neighborhood operator based on utility assignment is helpful for the algorithm to select a more promising solution, thereby enhancing the search performance in many-objective optimization. In addition, the extended distance-based environmental selection strategy is developed to considerably improve the accuracy and effectiveness of updating the parent solutions of different subproblems. Experimental results on two widely used test suits demonstrate the competitiveness of the proposed algorithm in terms of solution accuracy compared with eight state-of-the-art multiobjective optimization algorithms.
更多查看译文
关键词
Multiobjective optimization,Evolutionary algorithm,Decomposition,Neighborhood adjustment,Extended distance-based selection
AI 理解论文
溯源树
样例
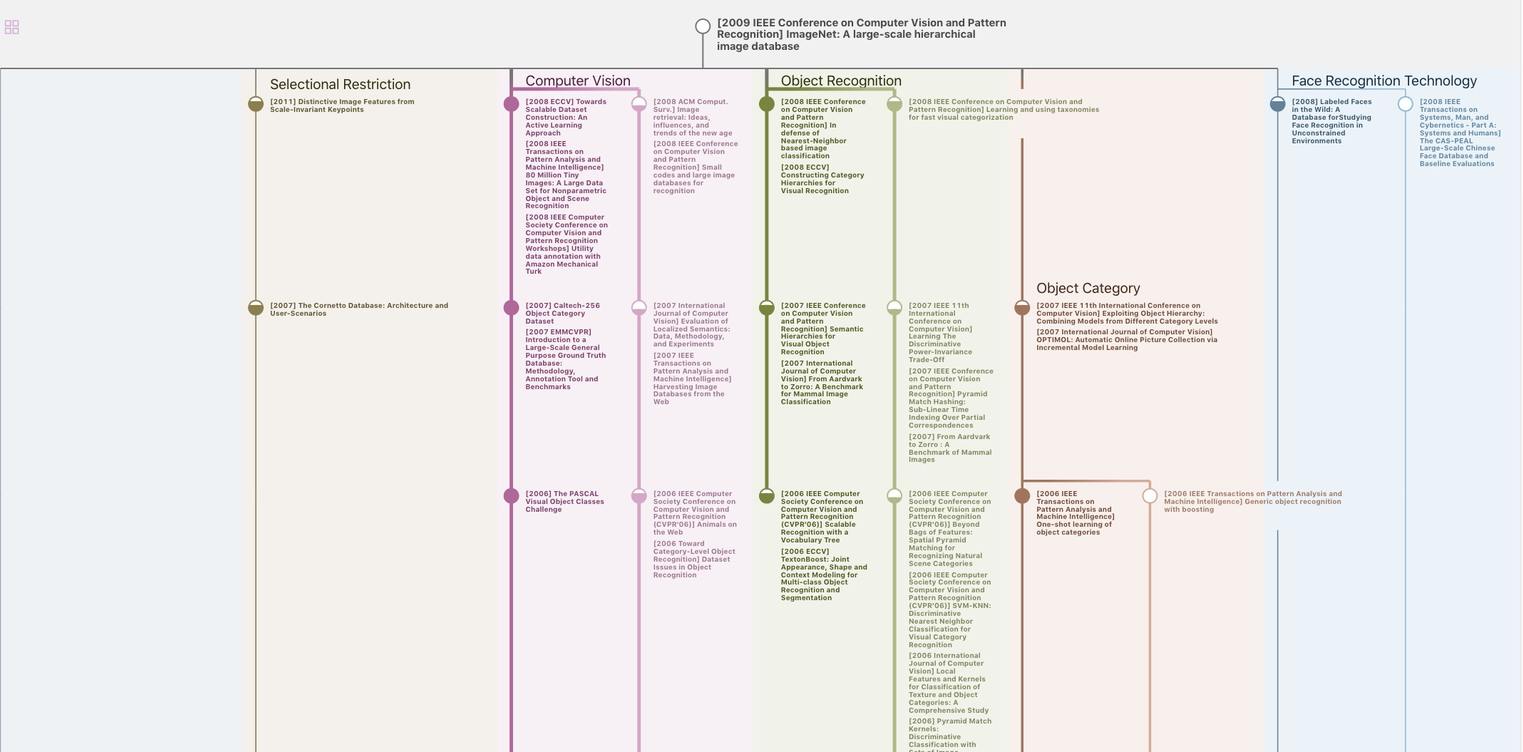
生成溯源树,研究论文发展脉络
Chat Paper
正在生成论文摘要