Stochastic variational inference for scalable non-stationary Gaussian process regression
STATISTICS AND COMPUTING(2023)
摘要
A natural extension to standard Gaussian process (GP) regression is the use of non-stationary Gaussian processes, an approach where the parameters of the covariance kernel are allowed to vary in time or space. The non-stationary GP is a flexible model that relaxes the strong prior assumption of standard GP regression, that the covariance properties of the inferred functions are constant across the input space. Non-stationary GPs typically model varying covariance kernel parameters as further lower-level GPs, thereby enabling sampling-based inference. However, due to the high computational costs and inherently sequential nature of MCMC sampling, these methods do not scale to large datasets. Here we develop a variational inference approach to fitting non-stationary GPs that combines sparse GP regression methods with a trajectory segmentation technique. Our method is scalable to large datasets containing potentially millions of data points. We demonstrate the effectiveness of our approach on both synthetic and real world datasets.
更多查看译文
关键词
Approximate Bayesian inference,Variational inference,Machine learning,Large-scale data,Gaussian process,Non-stationary
AI 理解论文
溯源树
样例
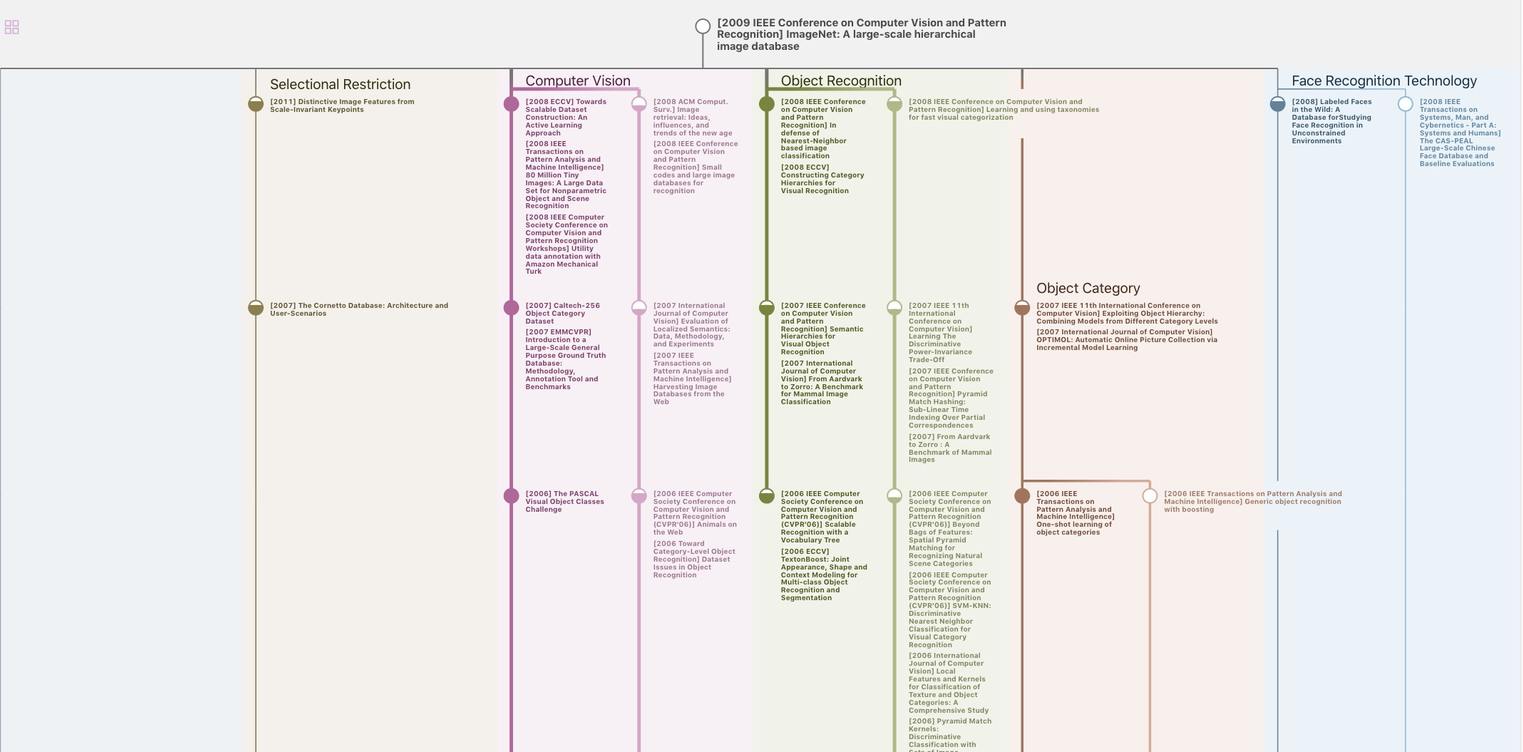
生成溯源树,研究论文发展脉络
Chat Paper
正在生成论文摘要