Predicting Regional Wastewater Treatment Plant Discharges Using Machine Learning and Population Migration Big Data
ACS ES&T WATER(2023)
摘要
Quantifying the temporal variation of wastewater treatment plant (WWTP) discharges is essential for water pollution control and environment protection in metropolitan areas. This study develops an ensemble machine learning (ML) model to predict discharges from WWTPs and to quantify the contribution of extraneous water (mixed precipitation and infiltrated groundwater) by leveraging the power of ML and population migration big data. The approach is applied to predict the discharges at 265 WWTPs in the Guangdong-Hong Kong-Macao Greater Bay Area (GBA) in China. The major conclusions are as follows. First, the ensemble ML model provides an efficient and reliable way to predict WWTP discharges using data easily accessible to the public. The predicted treated sewage amount increased from 20.4 x 106 m3/day in 2015 to 24.5 x 106 m3/day in 2020. Second, the predictors, including daily precipitation, average precipitation of past proceeding days, daily temperature, and population migration, play different roles in predicting different city's discharges. Finally, mixed precipitation and infiltrated groundwater account for, on average, 1.6 and 10.3% of total discharges from WWTPs in the GBA. This study represents the first attempt to bring population migration big data into data-driven environmental engineering modeling and can be easily extended to predict other environmental variables of concern.
更多查看译文
关键词
Guangdong-Hong Kong-Macao Greater Bay Area,wastewater treatment plants,machine learning,Bayesian model averaging,population migration,infiltrated groundwater
AI 理解论文
溯源树
样例
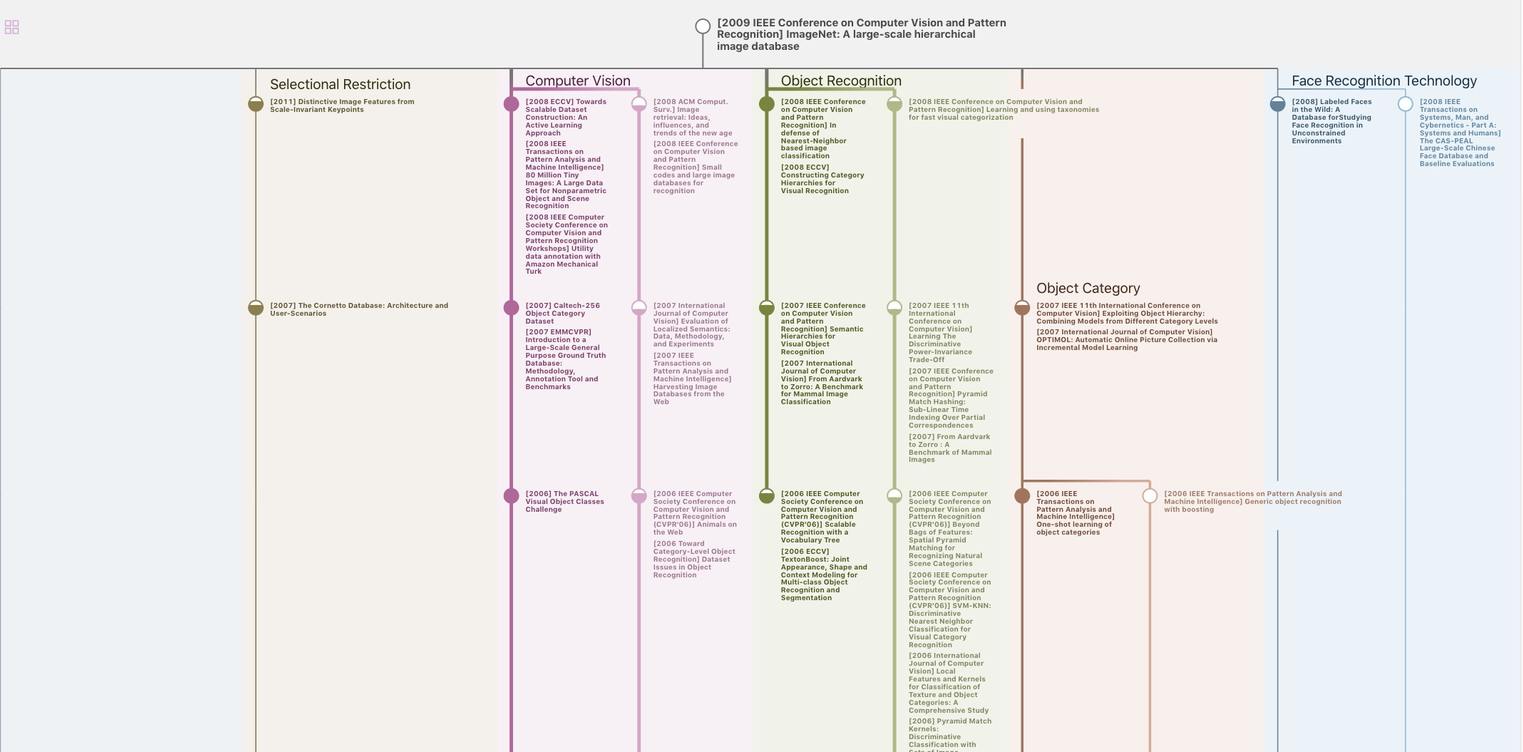
生成溯源树,研究论文发展脉络
Chat Paper
正在生成论文摘要