Forecasting a Nonstationary Time Series Using a Mixture of Stationary and Nonstationary Factors as Predictors
JOURNAL OF BUSINESS & ECONOMIC STATISTICS(2024)
摘要
We develop a method for constructing prediction intervals for a nonstationary variable, such as GDP. The method uses a Factor Augmented Regression (FAR) model. The predictors in the model include a small number of factors generated to extract most of the information in a set of panel data on a large number of macroeconomic variables that are considered to be potential predictors. The novelty of this article is that it provides a method and justification for a mixture of stationary and nonstationary factors as predictors in the FAR model; we refer to this as mixture-FAR method. This method is important because typically such a large set of panel data, for example the FRED-QD, is likely to contain a mixture of stationary and nonstationary variables. In our simulation study, we observed that the proposed mixture-FAR method performed better than its competitor that requires all the predictors to be nonstationary; the MSE of prediction was at least 33% lower for mixture-FAR. Using the data in FRED-QD for the United States, we evaluated the aforementioned methods for forecasting the nonstationary variables, GDP and Industrial Production. We observed that the mixture-FAR method performed better than its competitors.
更多查看译文
关键词
Factor augmented regression,Generated factors,Gross domestic product,High dimensional data,Industrial production,Panel data
AI 理解论文
溯源树
样例
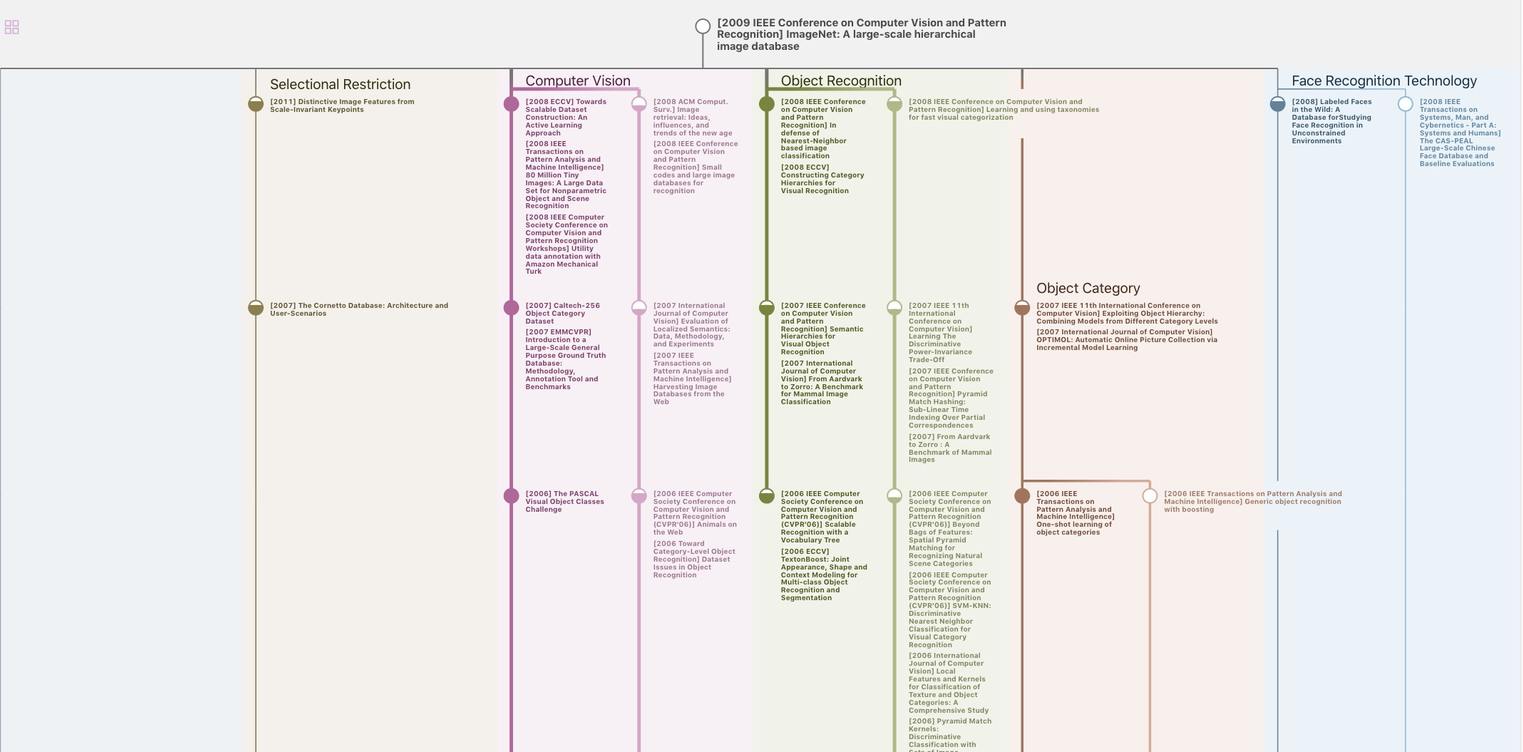
生成溯源树,研究论文发展脉络
Chat Paper
正在生成论文摘要