Document-level relation extraction with two-stage dynamic graph attention networks
KNOWLEDGE-BASED SYSTEMS(2023)
摘要
Document-level Relation Extraction (RE) aims to infer complex semantic relations between entities in a document. Previous approaches leverage a multi-classification model to predict relation types between each entity pair. However, in contrast to sentence-level RE, document-level RE contains various entities expressed by mentions appearing across multiple sentences in a document. Therefore, the amount of negative instances ('no relationship') significantly outnumbers that of other positive instances in document-level RE. In addition, most existing methods construct static graphs with heuristic rules to capture the interactions among entities. However, these heuristic rules ignore the specificities of the documents. In this study, we propose a novel two-stage framework to extract document-level relations based on dynamic graph attention networks, namely TDGAT. In the first stage, we capture the relational links of the entity pairs using a binary classification model. In the second stage, we extract fine-grained relations among entities, including the type of 'NA (no relationship)'. To reduce error propagation, we regard the entity pair links predicted in the first stage as the prior information and leverage them to reconstruct the document-level graphs of the second stage. In this manner, we can provide extra head and tail entity connection information for predicting relations in a document. Furthermore, we propose a dynamic graph strategy to explore the multi-hop interactions between related information. The experimental results show that our framework outperforms most existing models on the public document-level dataset DocRED. The extensive analysis demonstrates the effectiveness of our TDGAT in extracting inter-sentence relations.(c) 2023 Elsevier B.V. All rights reserved.
更多查看译文
关键词
Document-level relation extraction,Graph attention networks,Dynamic graph,Two-stage framework,Pretrained language models
AI 理解论文
溯源树
样例
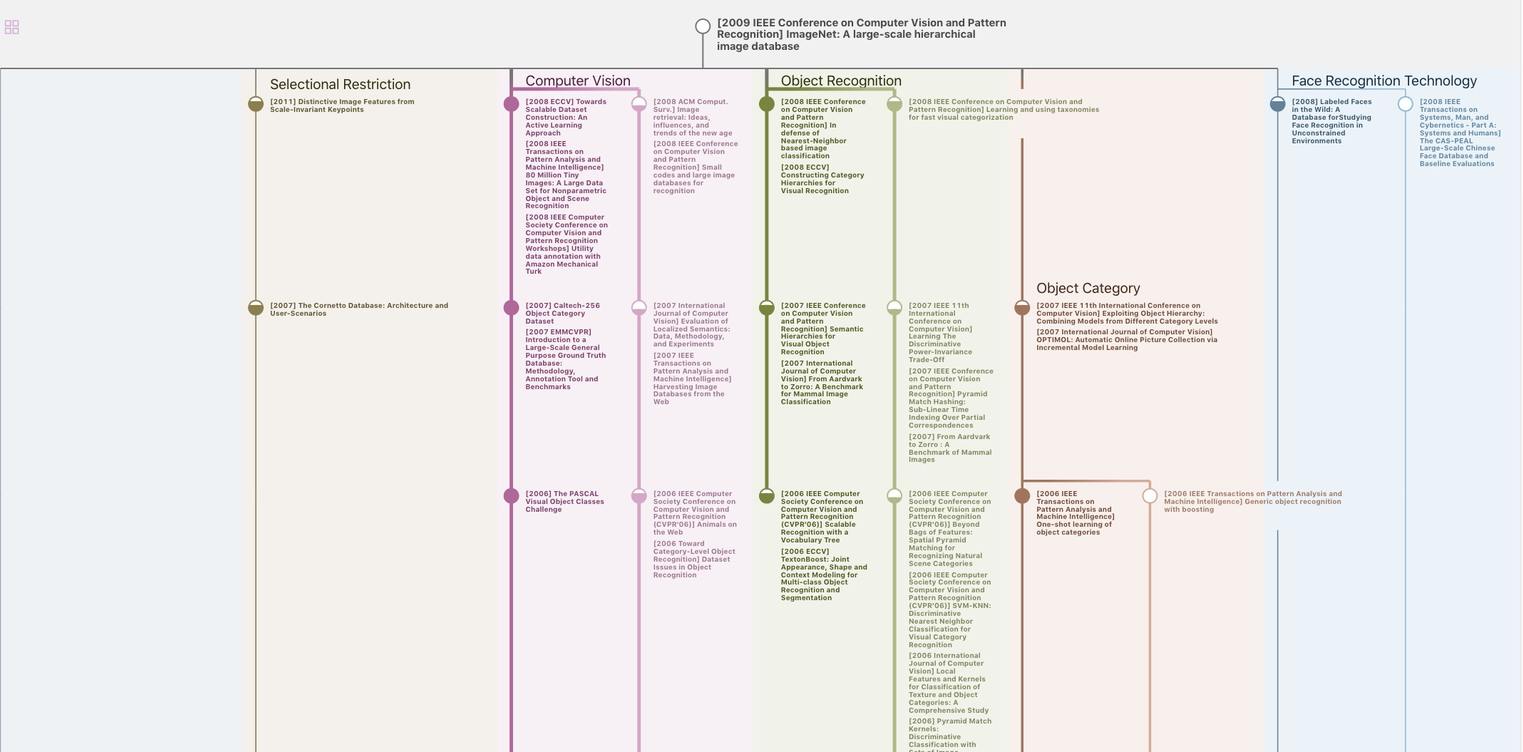
生成溯源树,研究论文发展脉络
Chat Paper
正在生成论文摘要