An improved many-objective artificial bee colony algorithm for cascade reservoir operation
NEURAL COMPUTING & APPLICATIONS(2023)
摘要
Artificial bee colony (ABC) has shown good performance on single-objective and ordinary multi-objective optimization problems. However, ABC faces some difficulties with increasing number of objectives. The selection pressure based on Pareto dominance degrades severely. The original ABC shows weak exploitation ability and slow convergence speed. To help ABC solve many-objective optimization problems (MaOPs), this paper proposes an improved many-objective ABC algorithm based on decomposition and dimension learning (called MaOABC-DDL). Firstly, an MaOP is converted to several sub-problems by the decomposition. The original fitness function is not available because of multiple objective values. Then, a new fitness function is defined based on the ranking of each objective. Solutions with good fitness values are selected to form an elite set. To improve the convergence, an elite set guided search strategy and dimension learning are designed for the employed bee and onlooker bee stages, respectively. Moreover, the scout bee stage is modified to dynamically allocate computing resources. To verify the performance of MaOABC-DDL, the DTLZ and MaF benchmark problems with 3, 5, 8, and 15 objectives are tested. Results show that MaOABC-DDL can obtain better performance when compared with seven other many-objective evolutionary algorithms. Finally, MaOABC-DDL is applied to cascade reservoir operation. Simulation results show that our approach still achieves promising performance.
更多查看译文
关键词
Artificial bee colony algorithm,Swarm intelligence,Many-objective optimization,Cascade reservoir operation
AI 理解论文
溯源树
样例
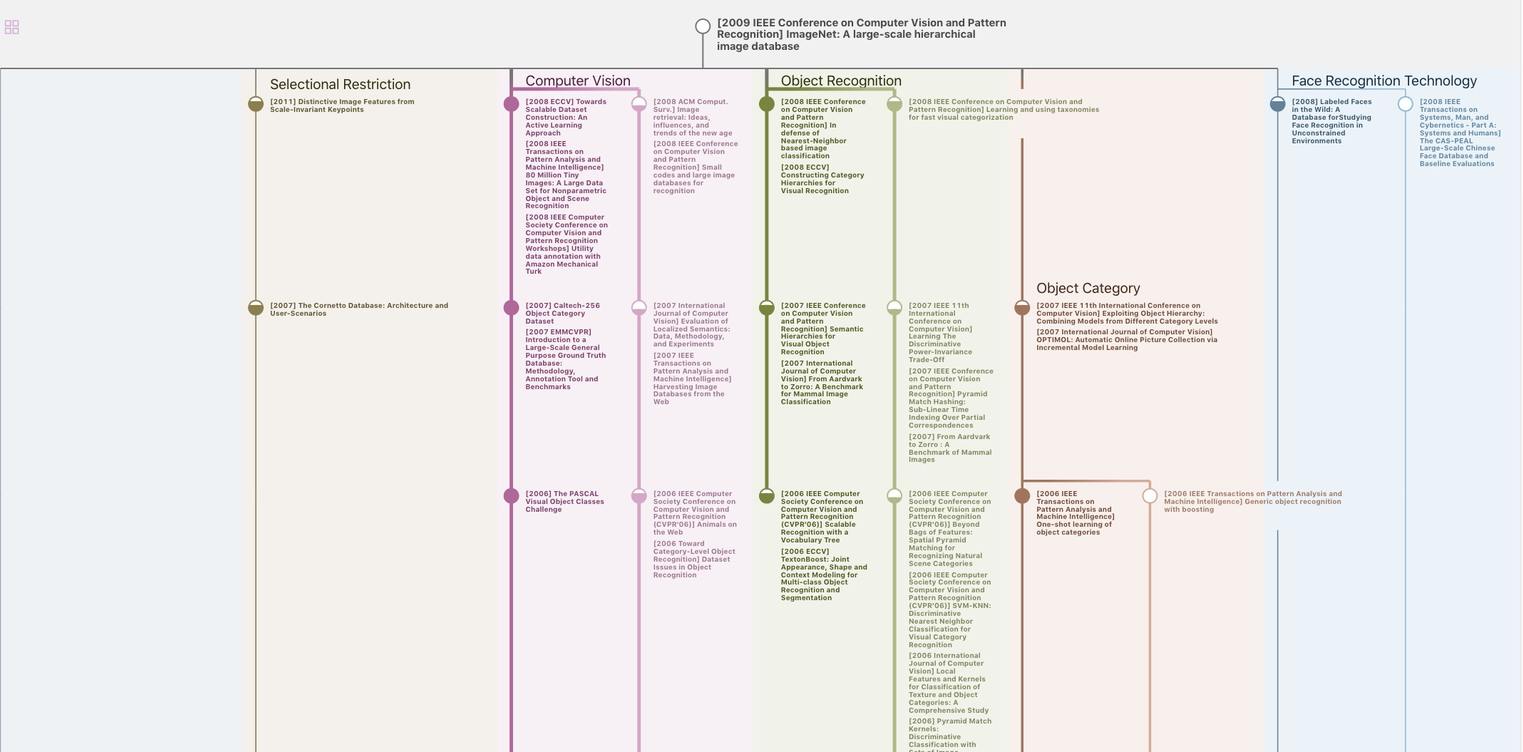
生成溯源树,研究论文发展脉络
Chat Paper
正在生成论文摘要