Investigating the Impact of Calibration on the Quality of Explanations
ANNALS OF MATHEMATICS AND ARTIFICIAL INTELLIGENCE(2023)
摘要
Predictive models used in Decision Support Systems (DSS) are often requested to explain the reasoning to users. Explanations of instances consist of two parts; the predicted label with an associated certainty and a set of weights, one per feature, describing how each feature contributes to the prediction for the particular instance. In techniques like Local Interpretable Model-agnostic Explanations (LIME), the probability estimate from the underlying model is used as a measurement of certainty; consequently, the feature weights represent how each feature contributes to the probability estimate. It is, however, well-known that probability estimates from classifiers are often poorly calibrated, i.e., the probability estimates do not correspond to the actual probabilities of being correct. With this in mind, explanations from techniques like LIME risk becoming misleading since the feature weights will only describe how each feature contributes to the possibly inaccurate probability estimate. This paper investigates the impact of calibrating predictive models before applying LIME. The study includes 25 benchmark data sets, using Random forest and Extreme Gradient Boosting (xGBoost) as learners and Venn-Abers and Platt scaling as calibration methods. Results from the study show that explanations of better calibrated models are themselves better calibrated, with ECE and log loss for the explanations after calibration becoming more conformed to the model ECE and log loss. The conclusion is that calibration makes the models and the explanations better by accurately representing reality.
更多查看译文
关键词
Predicting with confidence,Calibration,Explainable artificial intelligence,Decision support systems,Venn Abers,Uncertainty in explanations
AI 理解论文
溯源树
样例
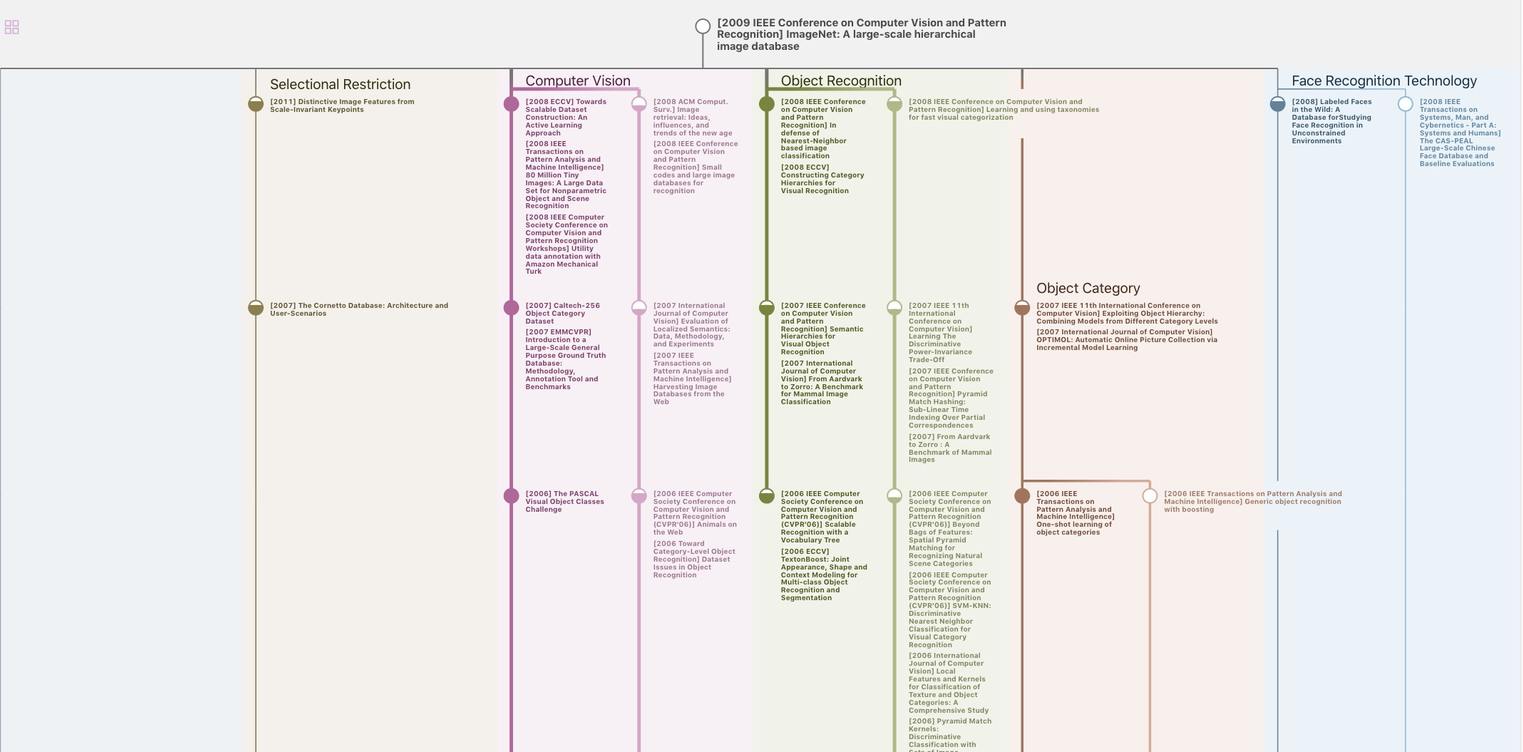
生成溯源树,研究论文发展脉络
Chat Paper
正在生成论文摘要