Energy efficient federated learning in internet of vehicles: A game theoretic scheme
TRANSACTIONS ON EMERGING TELECOMMUNICATIONS TECHNOLOGIES(2023)
摘要
A promising alternative to conventional centralized machine learning that protects privacy is federated learning. Energy efficiency, meanwhile, appears as a major problem in federated learning for the Internet of Vehicles due to frequent model aggregations. To reduce the convergence time, existing research focuses on improving vehicular communication. However, it increases the computational and communication load on servers and disregards the true energy costs of vehicles. Thus, we propose an energy-efficient federated learning scheme based on two-stage game theories. Specifically, the payoff function first takes into account the energy cost as well as the contribution of each vehicle, which wins the fair payment based on the Shapley value via a cooperative game. Second, under the fairness constraint, energy-efficient vehicles cooperatively form several coalitions for further federated worker selection through multiple rounds of the hedonic game. Additionally, we also develop a multiagent reinforcement learning algorithm that is leveraged for coalition formation to lower the energy cost in the game. Furthermore, we demonstrate that the game in our scheme is Nash stable. Extensive experimental results show that the scheme improves energy efficiency by around 68.8% in the best case compared to the most widely used method.
更多查看译文
关键词
learning,vehicles,internet
AI 理解论文
溯源树
样例
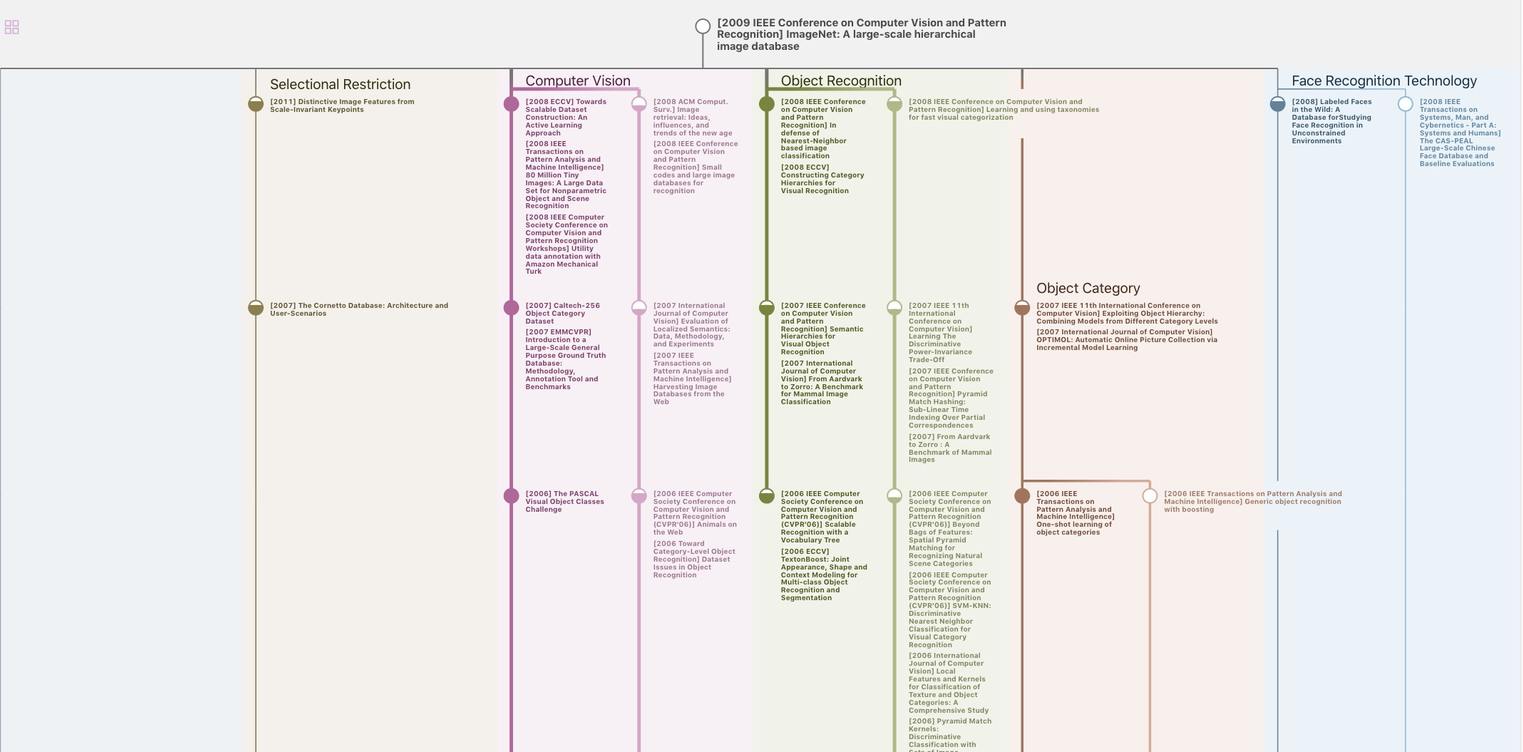
生成溯源树,研究论文发展脉络
Chat Paper
正在生成论文摘要