Probabilistic predictions for partial least squares using bootstrap
AICHE JOURNAL(2023)
摘要
Modeling the uncertainty in partial least squares (PLS) is made difficult because of the nonlinear effect of the observed data on the latent space that the method finds. We present an approach, based on bootstrapping, that automatically accounts for these nonlinearities in the parameter uncertainty, allowing us to equally well represent confidence intervals for points lying close to or far away from the latent space. To show the opportunities of this approach, we develop applications in determining the Design Space for industrial processes and model the uncertainty of spectroscopy data. Our results show the benefits of our method for accounting for uncertainty far from the latent space for the purposes of Design Space identification, and match the performance of well established methods for spectroscopy data.
更多查看译文
关键词
Design Space identification,model uncertainty,partial least squares,PLS,probabilistic prediction
AI 理解论文
溯源树
样例
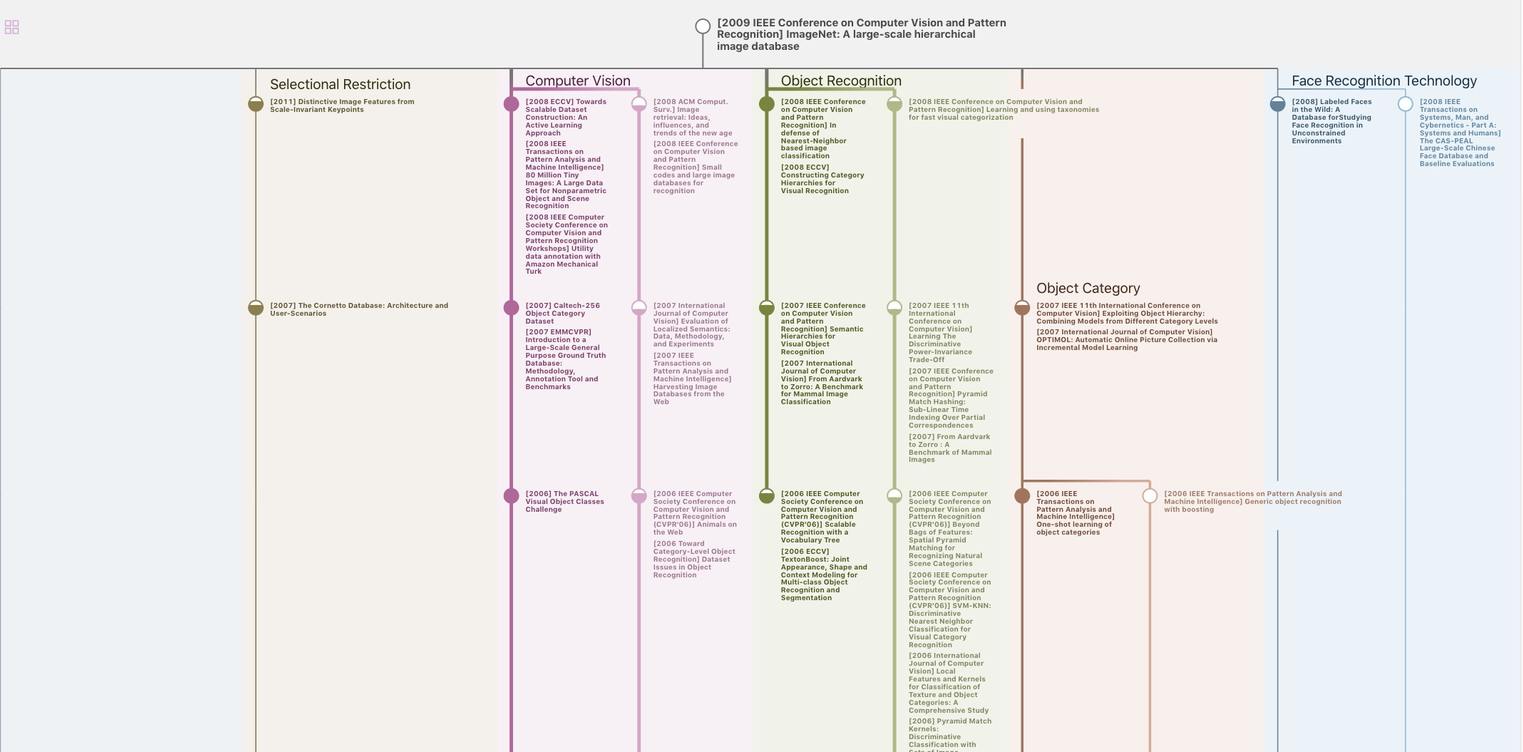
生成溯源树,研究论文发展脉络
Chat Paper
正在生成论文摘要