Identification of Prognostic Biomarkers for Gastric Cancer Using a Machine Learning Method
JOURNAL OF BIOLOGICAL REGULATORS AND HOMEOSTATIC AGENTS(2023)
摘要
Background: Gastric cancer (GC) is one of the leading causes of cancer-related deaths worldwide. Therefore, identifying prog-nostic biomarkers for GC is important to improve the clinical outcomes of patients. Methods: Univariate Cox survival analysis and random survival forest (RSF) were performed on all genes in The Cancer Genome Atlas (TCGA) cohort I (n = 261) to screen for survival-related seed genes. A forward selection algorithm was used to further determine prognosis-related genes using ribonucleic acid sequencing (RNA-seq) or clinically integrated RNA-seq data, followed by the construction of prognostic models. The concordance index (C-index) and Akaike information criterion (AIC) were calcu-lated to identify the optimal model, the performance of which was further validated in TCGA cohort II (n = 109) and the Gene Expression Omnibus series 84437 (GSE84437) cohort (n = 431), and compared with five previous prediction models. Results: Four prognostic models were constructed using the machine learning method. Model 3, based on the RSF model and RNA-seq data, was identified as the optimal model (AIC = 1050.76, C-index = 0.74,p = 2.39 x 10-13). Compared with models 1, 2, and 4, model 3 showed the highest predictive accuracy in both the internal (C-index = 0.73,p = 1.48 x 10-2) and external (C-index = 0.62, p = 0.020) validation cohorts. Receiver operating characteristic curves also confirmed the robust ability of the nine-gene signature in model 3 to assess GC prognosis in both TCGA and GSE84437 cohorts, with all areas under curves over 0.65. Furthermore, the prognostic performance of model 3 outperformed that of the other five existing prediction models (C-index = 0.74,p = 2.39 x 10-13).Conclusions: We propose a nine-gene marker with high sensitivity and specificity as a powerful tool for predicting the prognosis of GC.
更多查看译文
关键词
gastric cancer,machine learning,random survival forest,survival analysis,prognostic biomarkers
AI 理解论文
溯源树
样例
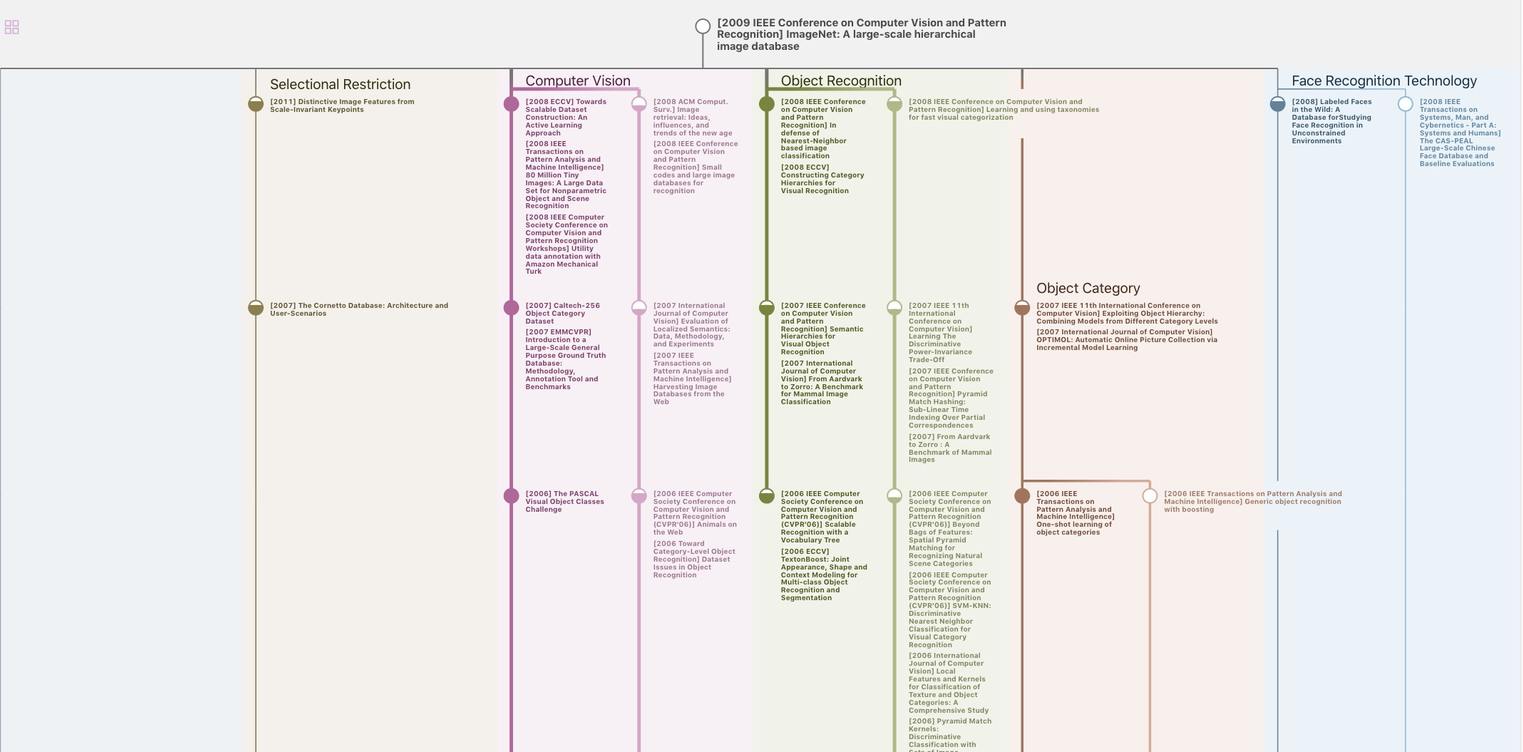
生成溯源树,研究论文发展脉络
Chat Paper
正在生成论文摘要