Performance Evaluation of Rockburst Prediction Based on PSO-SVM, HHO-SVM, and MFO-SVM Hybrid Models
MINING METALLURGY & EXPLORATION(2023)
摘要
Rockburst prediction is crucial in deep hard rock mines and tunnels to make safer working conditions. Due to the complex interaction of many factors involved in rockburst prediction, such as multi-variable and multi-interference factors, three hybrid support vector machine (SVM) models optimized by particle swarm optimization (PSO), Harris hawk optimization (HHO), and moth flame optimization (MFO) are proposed to predict rockburst hazard level (RHL). The RHL is determined according to four kinds of microseismic characteristic parameters including angular frequency ratio, total energy, apparent stress, and convexity radius. Then, six types of microseismic characteristic parameters are taken as input variables in 343 sets of data, including angular frequency ratio and total energy, etc.. And the RHL is taken as the output target of rockburst prediction. The classification performance of PSO-SVM, HHO-SVM, and MFO-SVM hybrid models is evaluated by accuracy (ACC), precision (PRE), and kappa coefficient. Findings reveal that the MFO-SVM model performs best in terms of accuracy, with ACC, PRE, and kappa coefficients reaching 0.9559, 0.9063, and 0.9094 respectively, while PSO-SVM and HHO-SVM have similar performances. However, the PSO-SVM, HHO-SVM, and MFO-SVM all perform better than the unoptimized SVM model. This confirms that the three optimization algorithms significantly enhance the rockburst prediction capacity of the SVM model to help mine practitioners apply machine learning methods to rockburst prediction problems appropriately.
更多查看译文
关键词
Rockburst,Microseismic monitoring,Support vector machine,Particle swarm optimization,Harris hawk optimization,Moth flame optimization
AI 理解论文
溯源树
样例
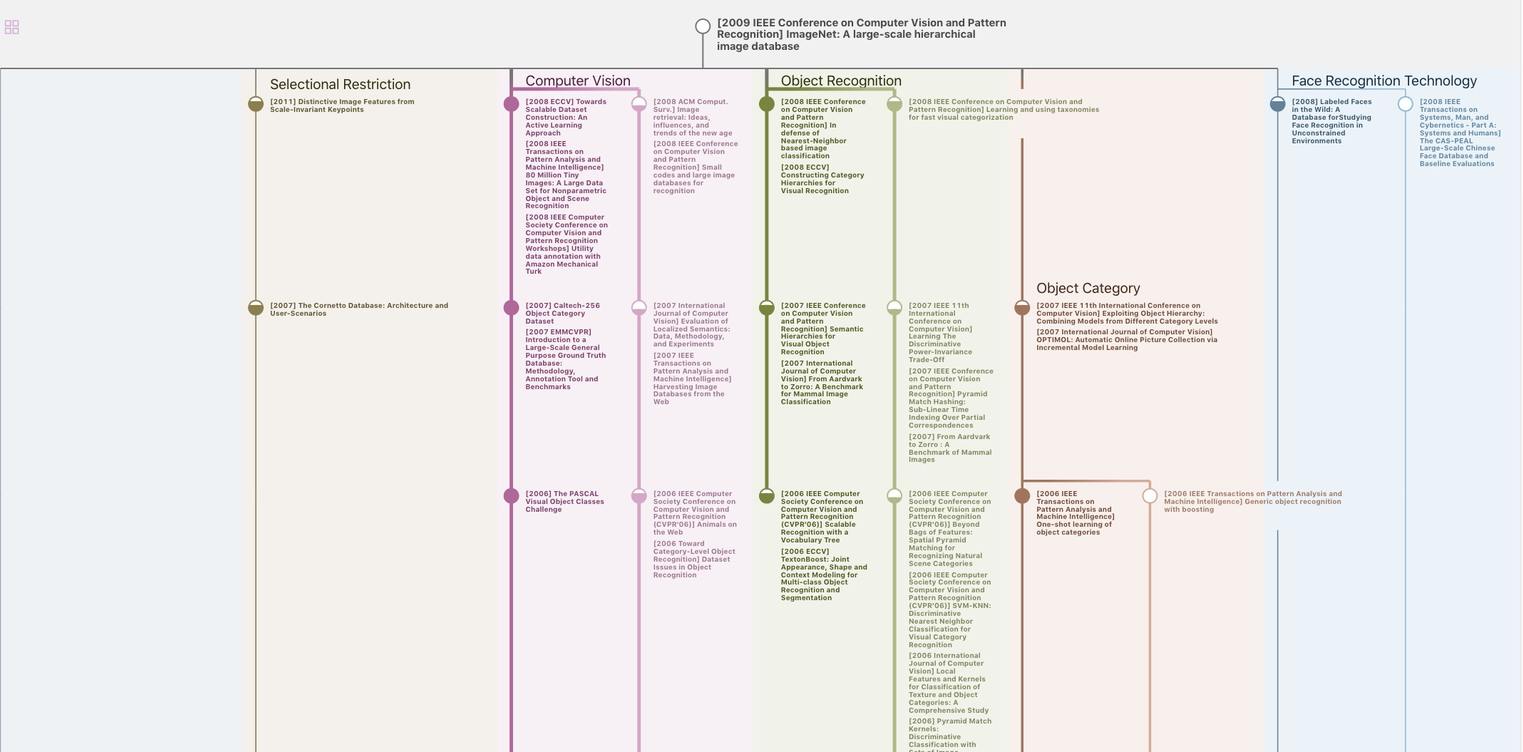
生成溯源树,研究论文发展脉络
Chat Paper
正在生成论文摘要