Comparing the performance of three common species distribution modelling frameworks for freshwater environments through application to eel species in New Zealand
CANADIAN JOURNAL OF FISHERIES AND AQUATIC SCIENCES(2023)
摘要
Globally, many freshwater species are depleting and require population-level assessments. Many species distribution mod-elling frameworks are available for such assessments, but comparisons are needed to understand their predictive performance under different settings. K-fold cross-validation techniques were employed to compare the performance of three commonly used frameworks: machine learning, spatiotemporal modelling, and Gaussian process (GP) modelling. Through application to New Zealand populations of longfin eel (Anguilla dieffenbachii) and shortfin eel (Anguilla australis), area under the receiver operating characteristic curve (AUC) and true skill statistic (TSS) model performance metrics were estimated. All modelling frameworks produced approximately consistent distribution maps but differed in predictive performance. AUC and TSS re-sults indicated that model predictions from the spatiotemporal modelling framework were the most accurate, followed by GP modelling. However, all modelling frameworks performed similarly when training and test data were spatially independent. In addition to having the best predictive performance, the spatiotemporal modelling framework showed the greatest promise for advancement in population-level assessment and is therefore recommended. The results are useful for freshwater ecolo-gists and resource managers to make informed decisions on the appropriateness of a modelling framework for their research objective.
更多查看译文
关键词
spatiotemporal model,regularized random forests,Gaussian random field model,species distribution modelling,model comparison,freshwater environments
AI 理解论文
溯源树
样例
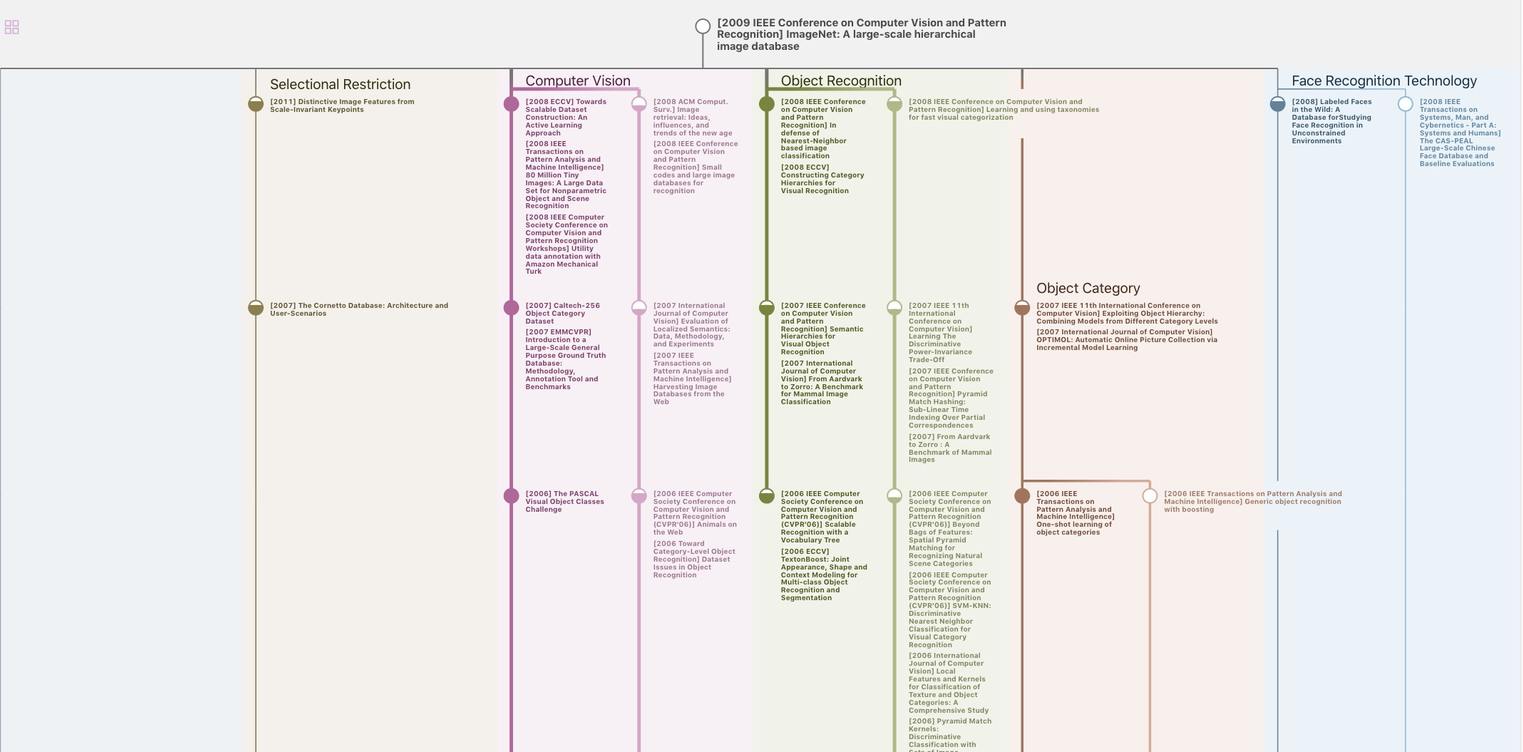
生成溯源树,研究论文发展脉络
Chat Paper
正在生成论文摘要