Iterative-AMC: a novel model compression and structure optimization method in mechanical system safety monitoring
STRUCTURAL HEALTH MONITORING-AN INTERNATIONAL JOURNAL(2023)
摘要
With the rapid development of artificial intelligence, various fault diagnosis methods based on the deep neural networks have made great advances in mechanical system safety monitoring. To get the high accuracy for the fault diagnosis, researchers tend to adopt the deep network layers and amount of neurons or kernels in each layer. This results in a large redundancy and the structure uncertainty of the fault diagnosis networks. Moreover, it is hard to deploy these networks on the embedded platforms because of the large scales of the network parameters. This brings huge challenges to the practical application of the intelligent diagnosis algorithms. To solve the above problems, an iterative automatic machine compression method, named Iterative-AMC, is proposed in this paper. The proposed method aims to automatically compress and optimize the structure of the large-scale neural networks. Experiments are carried out based on two test benches. With the proposed Iterative-AMC method, the problems of the parameter redundancy and the structure uncertainty can be addressed. The scale of the original network can be greatly compressed, and the compressed fault diagnosis network is successfully deployed on a small-scale FPGA chip.
更多查看译文
关键词
Bearing fault diagnosis,neural network compression,reinforcement learning,the deep deterministic policy gradient,network pruning,FPGA
AI 理解论文
溯源树
样例
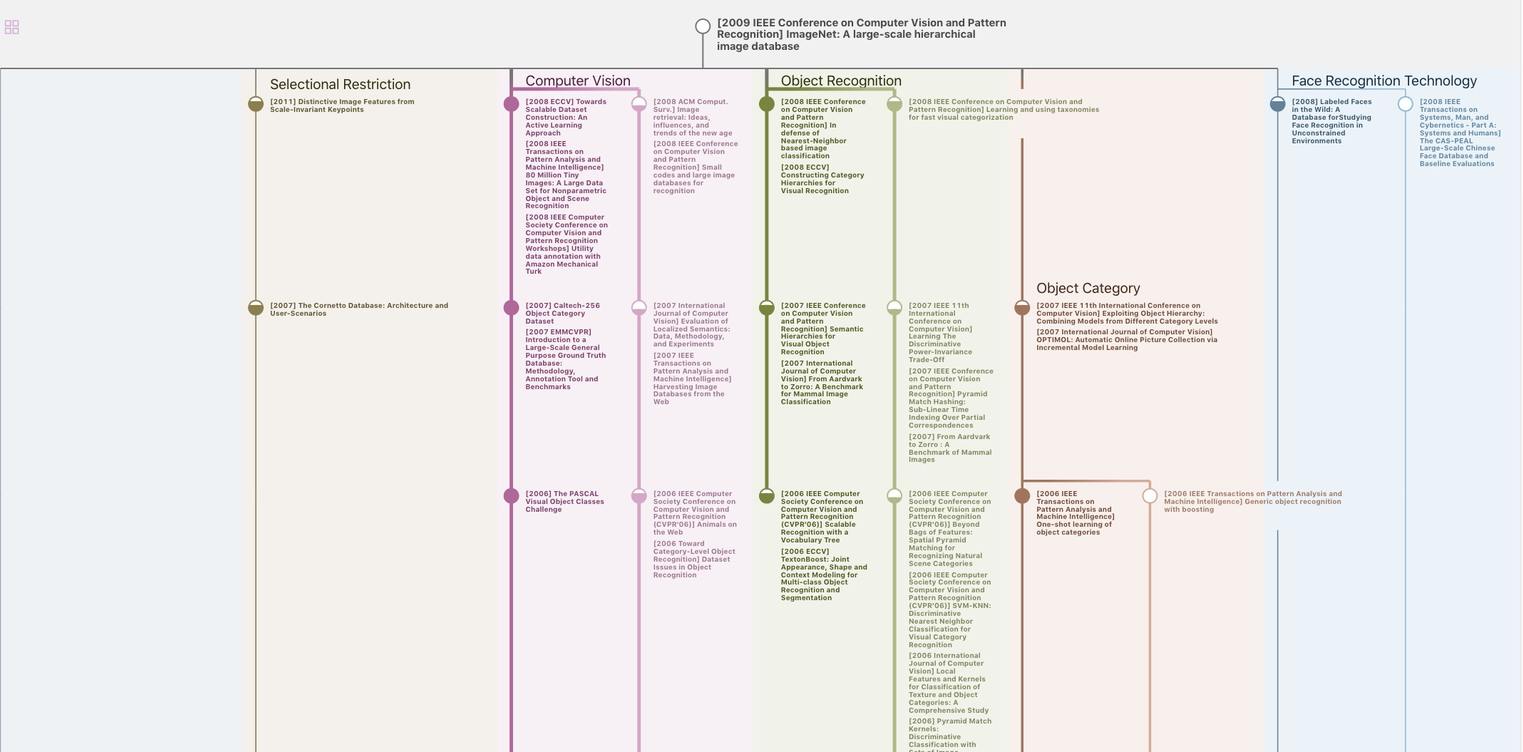
生成溯源树,研究论文发展脉络
Chat Paper
正在生成论文摘要