A Gaussian process guided super resolution sampling strategy for the efficient recovery of complex surfaces
MEASUREMENT SCIENCE AND TECHNOLOGY(2023)
摘要
Accurate recovery of complex surfaces of manufactured artefacts frequently requires intensive sampling, resulting in inefficient measurements for some point-by-point probe instruments. To tackle this problem, we fully exploit Gaussian process (GP) to guide the super resolution (SR) model to perform efficient and accurate sampling. The model makes use of a kernel-based GP method to model these low-frequency geometric features, while a pretrained SR method with multiple residual attention blocks is used to focus on the high-frequency features and further improve the details of the surface. In addition to geometric errors and distance information, global uncertainty from the statistical properties of the GP and an additional feature error from the SR are combined as critical criteria to select the most informative points of the surface. The effectiveness of the proposed method was demonstrated through several experiments on synthetic and real-world data, showing that the proposed method achieves state-of-the-art performance for pointwise measurements.
更多查看译文
关键词
Gaussian processes,super resolution,complex surfaces,adaptive sampling,sampling criteria
AI 理解论文
溯源树
样例
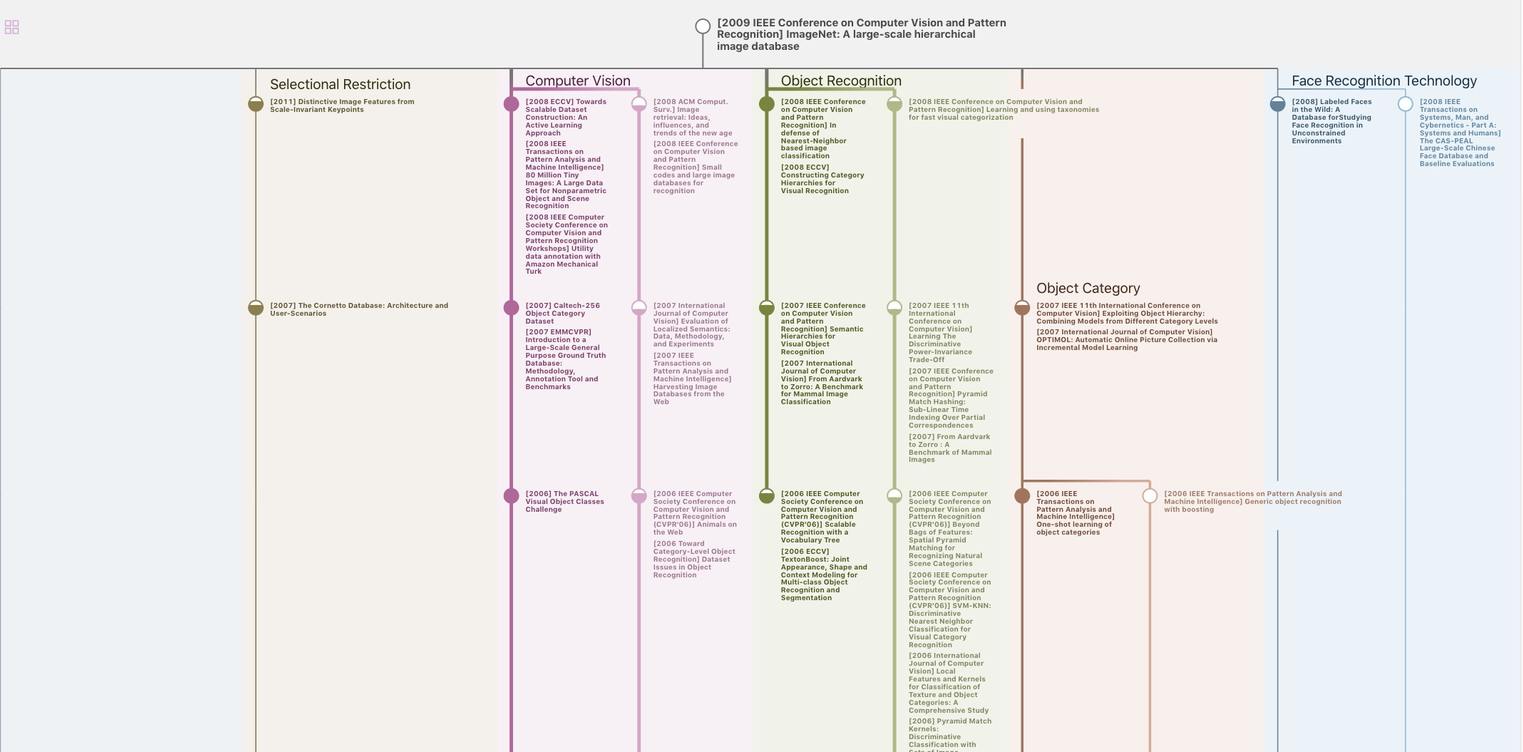
生成溯源树,研究论文发展脉络
Chat Paper
正在生成论文摘要