MDAFormer: Multi-level difference aggregation transformer for change detection of VHR optical imagery
INTERNATIONAL JOURNAL OF APPLIED EARTH OBSERVATION AND GEOINFORMATION(2023)
摘要
Accurate detection of land cover changes on the basis of very-high-resolution remote sensing is essential for many practical applications, like urban management, ecological health monitoring, and disaster loss assessment. Modern change detection (CD) methods are mostly based on convolutional neural network (CNN). Despite the good performance, CNN-based methods are restricted by the receptive field size and lack the ability of exploring the long-range dependencies in space-time. To address this issue, we propose a transformer-based CD model, which we called "multi-level difference aggregation transformer (MDAFormer)". The proposed MDAFormer first employs a pair of deep-aware transformer encoders to learn the deep feature representations. Also, a feature difference aggregation module can be utilized to bridge the semantic gaps by aggregating multi-level feature differences, which generates distinguished feature maps. In the decoder, these feature differences are upsampled to the same resolution and further aggregated together, followed by a progressive residual recovery module to refine these features. Finally, a weighted multi-scale constraint loss function is employed to mitigate the impact of class imbalance. The experimental results on three datasets demonstrate the effectiveness of MDAFormer in CD tasks.
更多查看译文
关键词
Change detection,Remote sensing images,Feature difference aggregation,Long-range dependency,Transformer
AI 理解论文
溯源树
样例
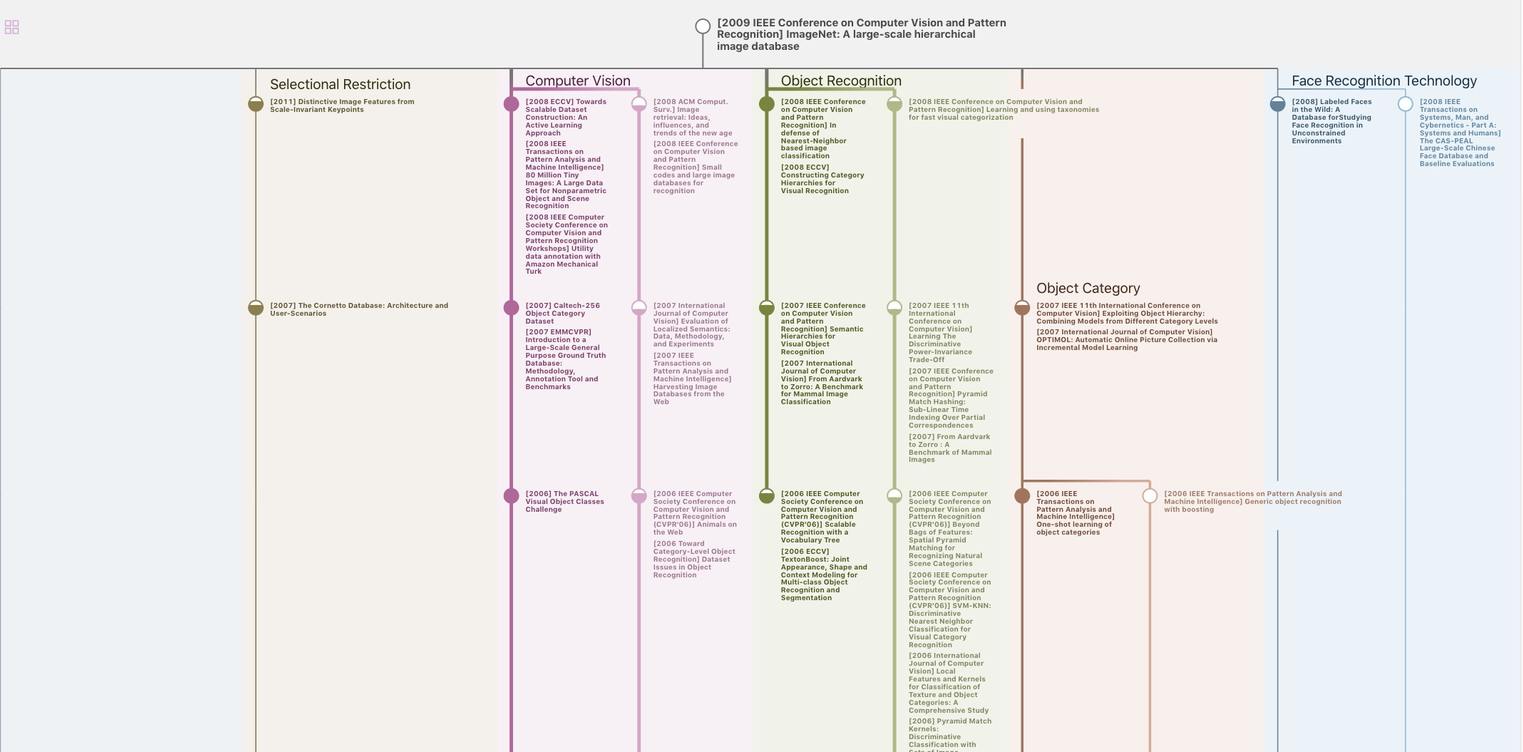
生成溯源树,研究论文发展脉络
Chat Paper
正在生成论文摘要