SRes-NeRF: Improved Neural Radiance Fields for Realism and Accuracy of Specular Reflections.
MMM (1)(2023)
摘要
The Neural Radiance Fields (NeRF) is a popular view synthesis technique that represents a scene using a multilayer perceptron (MLP) combined with classic volume rendering and uses positional encoding techniques to increase image resolution. Although it can effectively represent the appearance of a scene, they often fail to accurately capture and reproduce the specular details of surfaces and require a lengthy training time ranging from hours to days for a single scene. We address this limitation by introducing a representation consisting of a density voxel grid and an enhanced MLP for a complex view-dependent appearance and model acceleration. Modeling with explicit and discretized volume representations is not new, but we propose Swish Residual MLP (SResMLP). Compared with the standard MLP+ReLU network, the introduction of layer scale module allows the shallow information of the network to be transmitted to the deep layer more accurately, maintaining the consistency of features. Introduce affine layers to stabilize training, accelerate convergence and use the Swish activation function instead of ReLU. Finally, an evaluation of four inward-facing benchmarks shows that our method surpasses NeRF's quality, it only takes about 18 min to train from scratch for a new scene and accuracy capture the specular details of the scene surface. Excellent performance even without positional encoding.
更多查看译文
关键词
Scene representation, View synthesis, Image-based rendering, Volume rendering, 3D deep learning, Spectral bias
AI 理解论文
溯源树
样例
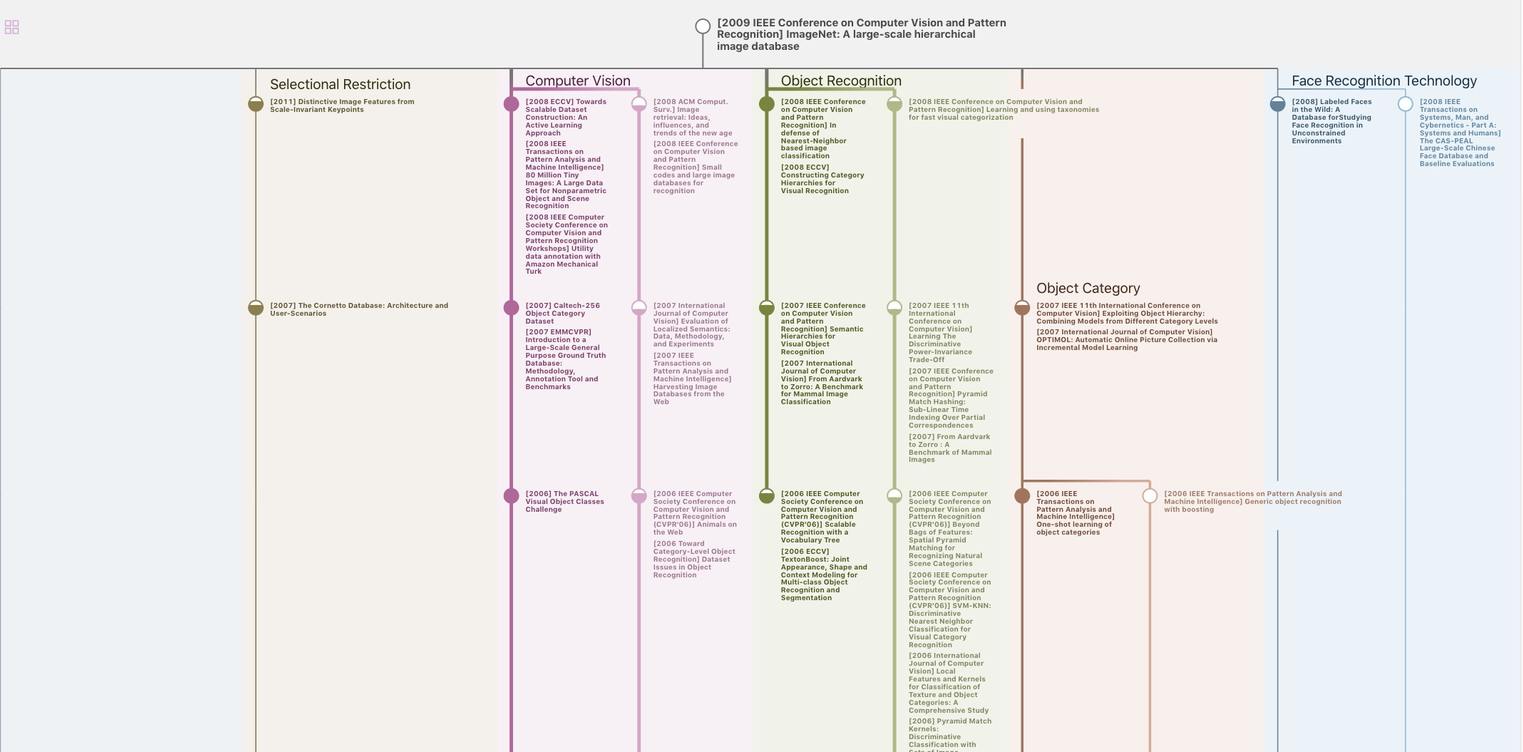
生成溯源树,研究论文发展脉络
Chat Paper
正在生成论文摘要