Truthful and performance-optimal computation outsourcing for aerial surveillance platforms via learning-based auction
Comput. Networks(2023)
摘要
This paper proposes a novel truthful computing algorithm for learning task outsourcing decision-making strategies in edge-enabled unmanned aerial vehicle (UAV) networks. In our considered scenario, a single UAV performs face identification in a monitored target area. The execution of the identification requires a certain computing power, and its complexity and time are dependent on the number of faces in the recorded images. As a consequence, the task cannot be fully executed by a single UAV under high image arrivals or with images that have a high density of faces. In those conditions, UAV can outsource the task to one of the nearby edges. Importantly, the computing task distribution should be energy-efficient and delay-minimal due to the constraints imposed by the UAV platform characteristics and applications. Based on those fundamental requirements, our proposed algorithm conducts sequential decision-making for image sharing with one selected edge. The edge is selected based on a second price auction for truthfulness. Besides the truthfulness guarantees, deep learning based approximation for the auction solution is used for revenue-optimality. Our evaluation demonstrates that the proposed algorithm achieves the desired performance.
更多查看译文
关键词
Unmanned aerial networks (UAVs),Surveillance,Auction
AI 理解论文
溯源树
样例
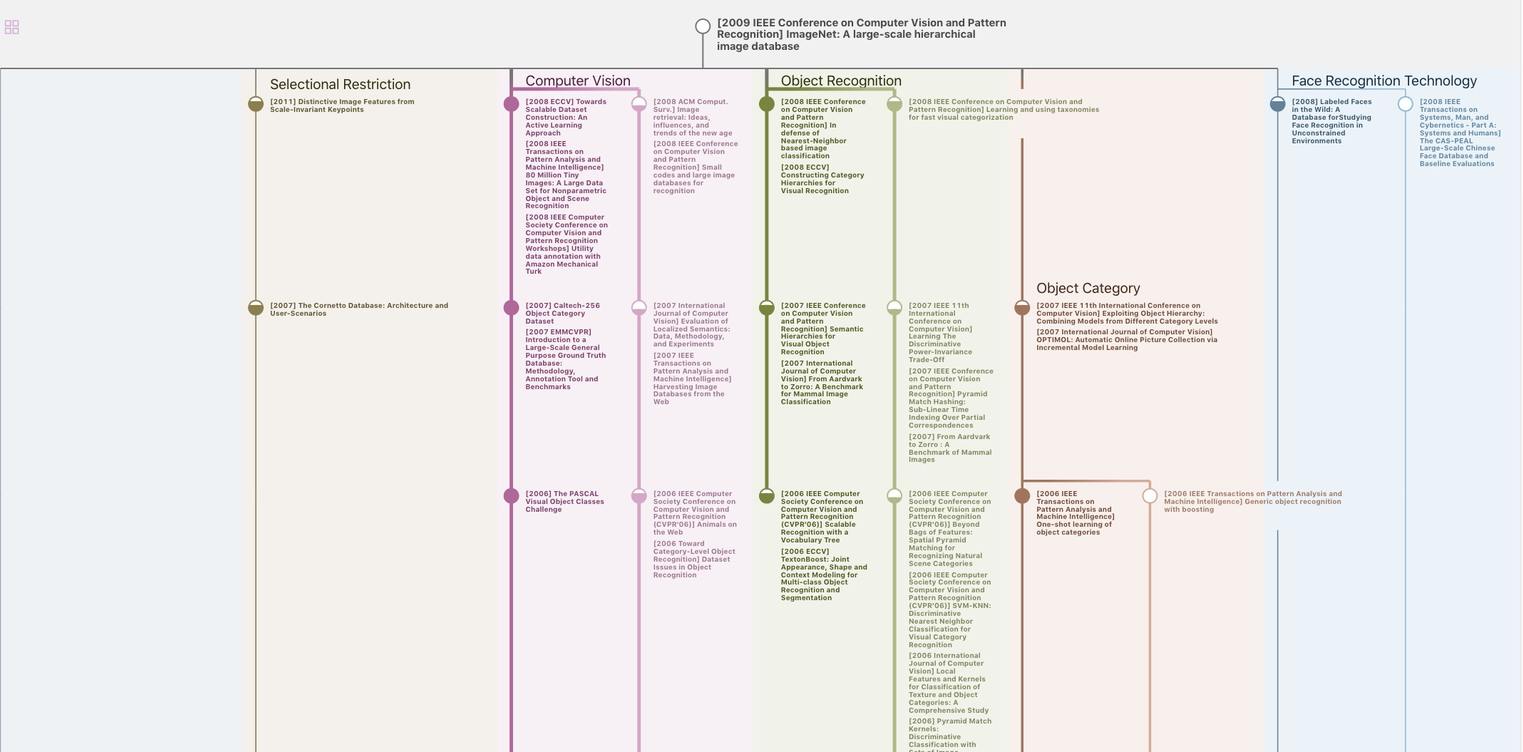
生成溯源树,研究论文发展脉络
Chat Paper
正在生成论文摘要