An Improved 3D-2D Convolutional Neural Network Based on Feature Optimization for Hyperspectral Image Classification
IEEE Access(2023)
摘要
As a new technology in the field of remote sensing, hyperspectral remote sensing has been widely used in land classification, mineral exploration, environmental monitoring, and other areas. In recent years, deep learning has achieved outstanding results in hyperspectral image classification tasks. However, problems such as low classification accuracy for small sample classes in unbalanced datasets and lack of robustness of the models usually lead to unstable classification performance of hyperspectral images. Therefore, from the perspective of feature optimization, we propose an improved hybrid convolutional neural network for hyperspectral image feature extraction and classification. Different from the current simple multi-scale feature extraction, we first optimize the features of each scale, and then perform multi-scale feature fusion. To this end, we use 3D dilated convolution to design a multi-level feature extraction block (MFB), which can be used to extract features with different correlation strengths at a fixed scale. Then, we construct a spatial multi-scale interactive attention (SMIA) module in the spatial feature enhancement phase, which can refine the multi-scale features through the attention weights of multi-scale feature interaction, and further improve the quality of spatial features. Finally, experiments were performed on different datasets, including balanced and unbalanced samples. The results show that the proposed model is more accurate and the extracted features are more robust.
更多查看译文
关键词
Feature extraction,Hyperspectral imaging,Kernel,Image classification,Convolutional neural networks,Three-dimensional displays,Hybrid convolutional neural network,feature extraction,attention mechanism,spectral-spatial classification,unbalanced dataset
AI 理解论文
溯源树
样例
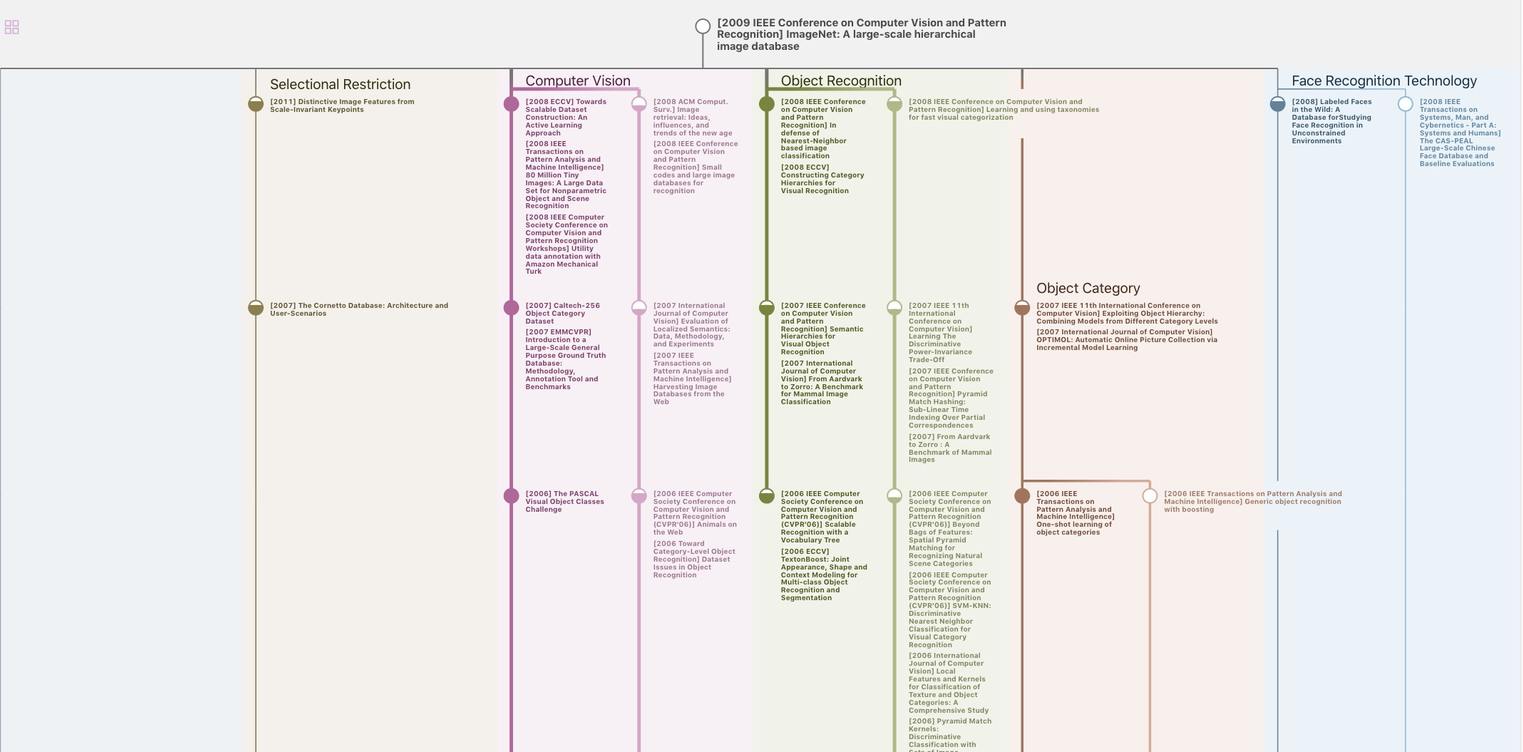
生成溯源树,研究论文发展脉络
Chat Paper
正在生成论文摘要