Estimation of Dynamical Noise Power in Unknown Systems.
IEEE Signal Process. Lett.(2023)
摘要
Noise can be modeled as a sequence of random variables defined on a probability space that may be added to a given dynamical system
$T$
, which is a map on a phase space. In the non-trivial case of dynamical noise
$\lbrace \varepsilon _{n}\rbrace _{n}$
, where
$\varepsilon _{n}$
follows a Gaussian distribution
$\mathcal {N}(0,\sigma ^{2})$
and the system output is
$x_{n} = T(x_{n-1};x_{0})+\varepsilon _{n}$
, without any specific knowledge or assumption about
$T$
, the quantitative estimation of the noise power
$\sigma ^{2}$
is a challenge. Here, we introduce a formal method based on the nonlinear entropy profile to estimate the dynamical noise power
$\sigma ^{2}$
without requiring knowledge of the specific
$T$
function. We tested the correctness of the proposed method using time series generated from Logistic maps and Pomeau-Manneville systems under different conditions. Our results demonstrate that the proposed estimation algorithm can properly discern different noise levels without any a priori information.
更多查看译文
关键词
Noise,complex systems,approximate entropy
AI 理解论文
溯源树
样例
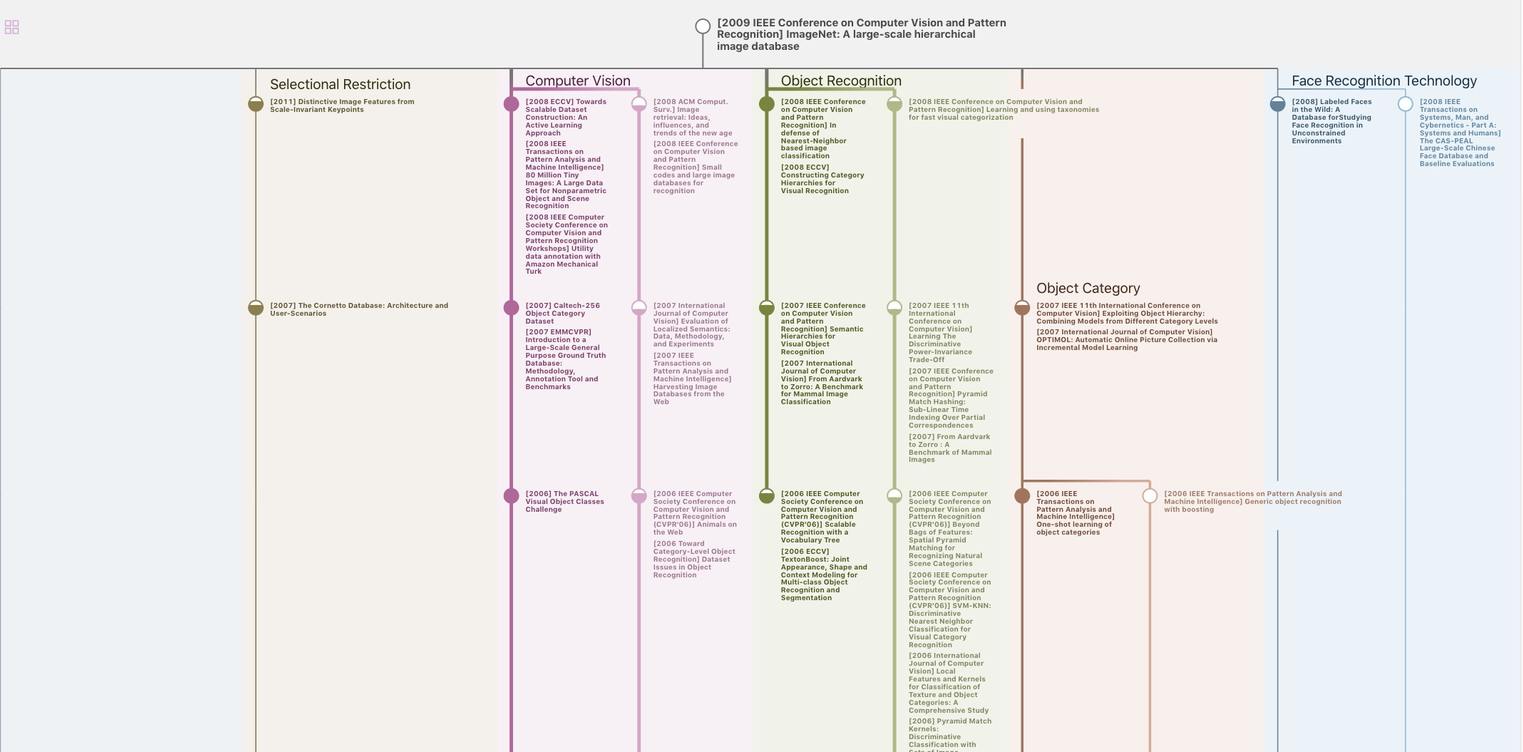
生成溯源树,研究论文发展脉络
Chat Paper
正在生成论文摘要