Evaluation of Joint Modeling Techniques for Node Embedding and Community Detection on Graphs.
ASONAM(2022)
摘要
Novel joint techniques capture both the microscopic context and the mesoscopic structure of networks by leveraging two previously separated fields of research: node representation learning (NRL) and community detection (CD). However, several limitations exist in the literature. First, a comprehensive comparison between these joint NRL-CD techniques is non-existent. Second, baseline techniques, datasets, evaluation metrics, and classification algorithms differ significantly between each method. Thirdly, the literature lacks a synchronized experimental approach, thus rendering comparison between these methods strenuous. To overcome these limitations, we present a uni-fied experimental setup mutually comparing six joint NRL-CD techniques and comparing them with corresponding NRL/CD baselines in three different settings: non-overlapping and over-lapping CD and node classification. Our results show that joint methods underperform on the node classification task but achieve relatively solid results for overlapping community detection. Our research contribution is two-fold: first, we show specific weaknesses of selected joint techniques in different tasks and data sets; and second, we suggest a more thorough experimental setup to benchmark joint techniques with simpler NRL and CD techniques.
更多查看译文
关键词
Node Representation Learning,Community Detection,Joint Modeling Techniques
AI 理解论文
溯源树
样例
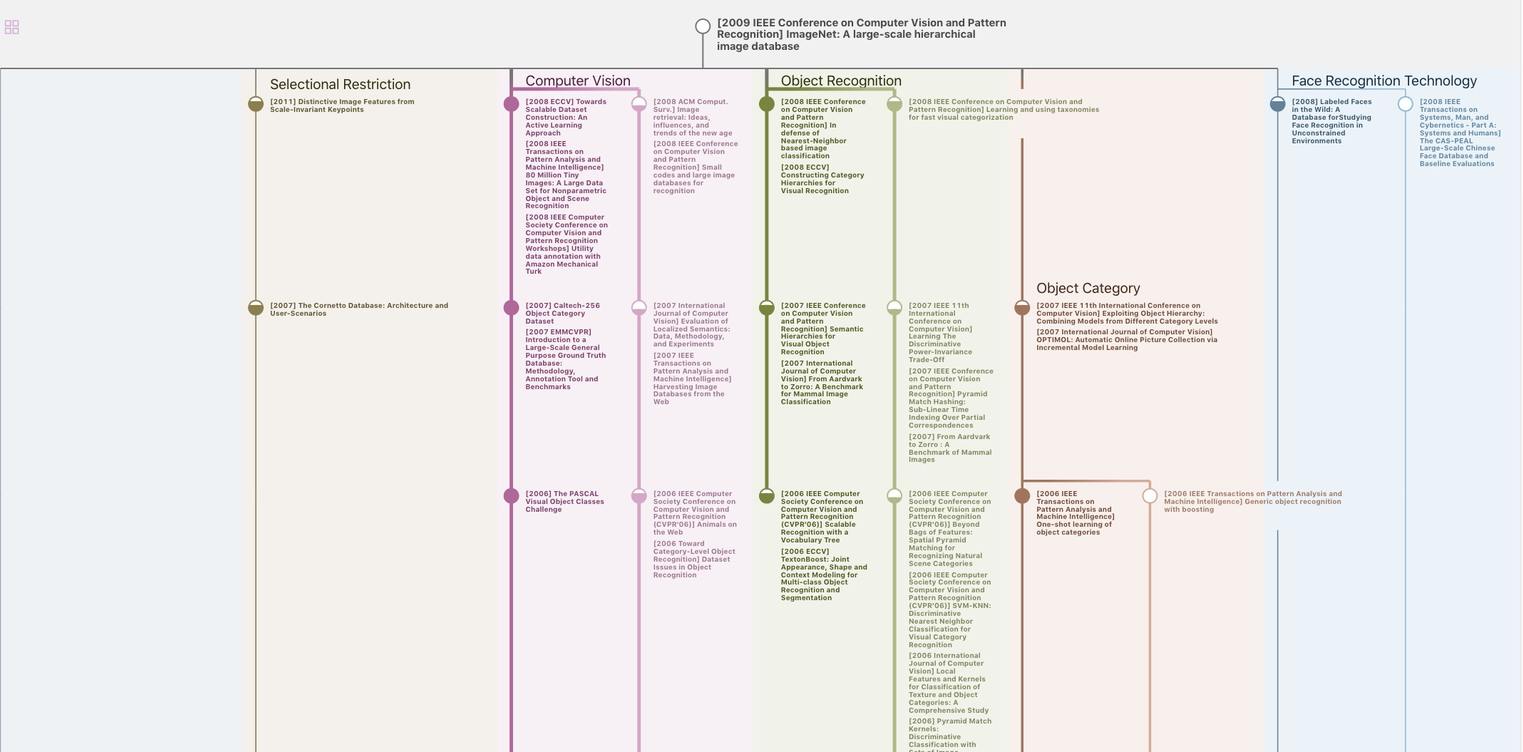
生成溯源树,研究论文发展脉络
Chat Paper
正在生成论文摘要