Automatic ICD-10 Code Association: A Challenging Task on French Clinical Texts
2023 IEEE 36TH INTERNATIONAL SYMPOSIUM ON COMPUTER-BASED MEDICAL SYSTEMS, CBMS(2023)
摘要
Automatically associating ICD codes with electronic health data is a well-known NLP task in medical research. NLP has evolved significantly in recent years with the emergence of pre-trained language models based on Transformers architecture, mainly in the English language. This paper adapts these models to automatically associate the ICD codes. Several neural network architectures have been experimented with to address the challenges of dealing with a large set of both input tokens and labels to be guessed. In this paper, we propose a model that combines the latest advances in NLP and multi-label classification for ICD-10 code association. Fair experiments on a Clinical dataset in the French language show that our approach increases the F-1-score metric by more than 55% compared to state-of-the-art results.
更多查看译文
关键词
natural language processing,icd-10,clinical document,unstructured data,multi-label classification,supervised learning,health,transformers
AI 理解论文
溯源树
样例
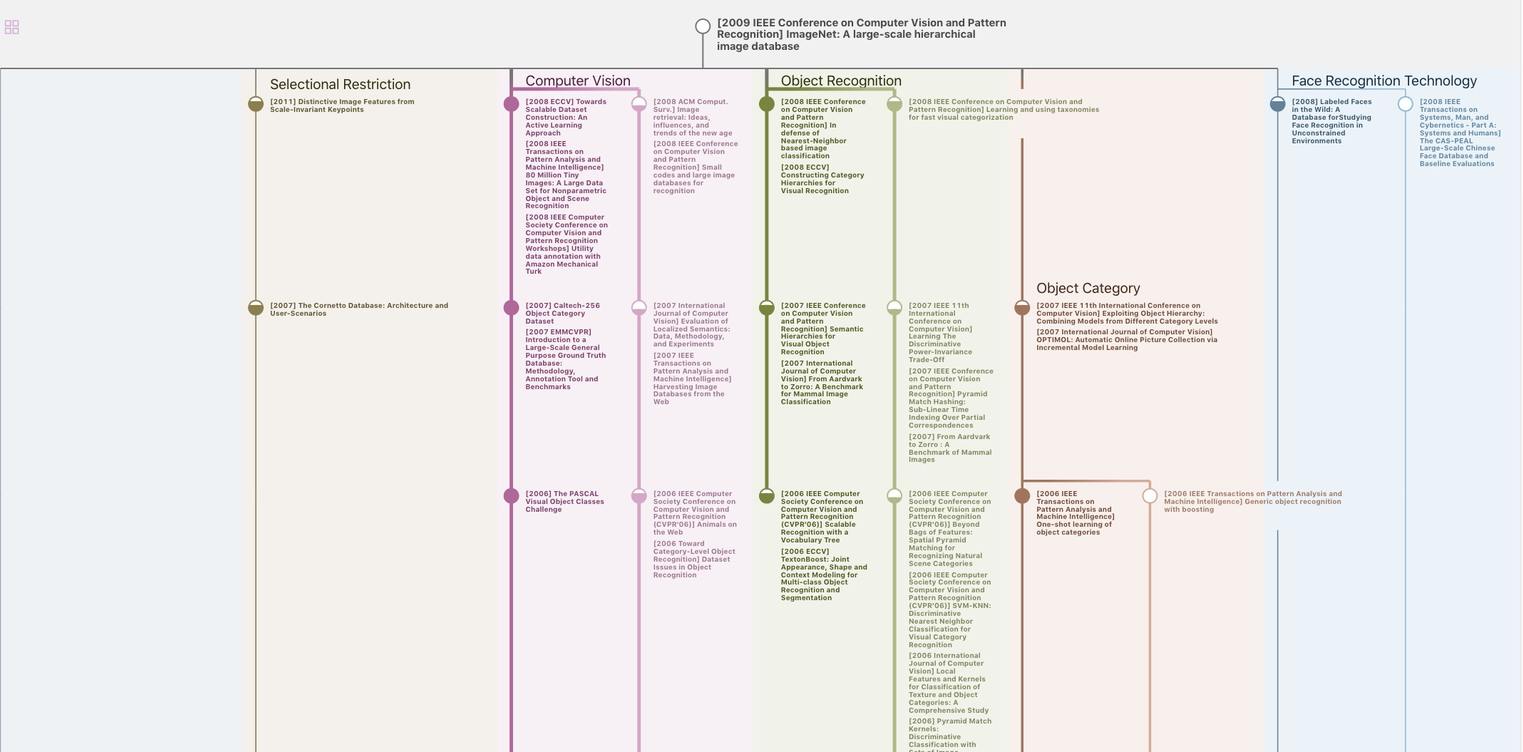
生成溯源树,研究论文发展脉络
Chat Paper
正在生成论文摘要