SPRITZ-PS: Validation of Synthetic Face Images Using a Large Dataset of Printed Documents
Multimedia Tools and Applications(2024)
摘要
Due to the appeal of Generative Adversarial Networks (GANs), synthetic face images generated with GAN models are difficult to differentiate from genuine human faces and may be used to create counterfeit identities. However, these face images contain artifacts presented in irises owing to the irregularity of highlights between the left and right irises. Adversaries can utilize PS documents of these images trying to conceal the artifacts of images, which makes it more challenging to distinguish between pristine and fake images. In order to tackle this, our research introduces a complete dataset and analytical tools that make a substantial contribution to multimedia forensics. This allows for the authentication of documents despite common alterations in the PS documents. Owing to the lack of large-scale reference IRIS datasets in the PS scenario, this study provided a pioneering dataset aiming to set a standard for multimedia forensic investigations. Given face images, we extracted iris images using the Dlib (King, J Mach Learn Res 10(60):1755–1758, 2020) and EyeCool (Wang et al. 2021) models, as described in Guo et al. (2022). However, in some cases, the potential eyelid occlusion phenomenon resulted in incomplete iris images. We utilized a hypergraph convolution-based image inpainting technique to complete the missing pixels in the extracted iris images, thus uncovering the intricate relationships within the iris data. To evaluate the IRIS image dataset and highlight associated issues, we conducted a series of analyses using Siamese Neural Networks, including ResNet50, Xception, VGG16, and MobileNet-v2, to measure the similarities between authentic and synthetic human iris images. Our SNN model, with four different backbones, effectively differentiated between genuine and synthetic iris images. For instance, using the Xception network, we achieved 56.76
更多查看译文
关键词
Multimedia forensics,Adversarial multimedia forensics,Machine and deep learning security,GAN-generated image detection,Printed and scanned IRIS detection,Generative adversarial networks (GANs),Artificial intelligence,CyberSecurity
AI 理解论文
溯源树
样例
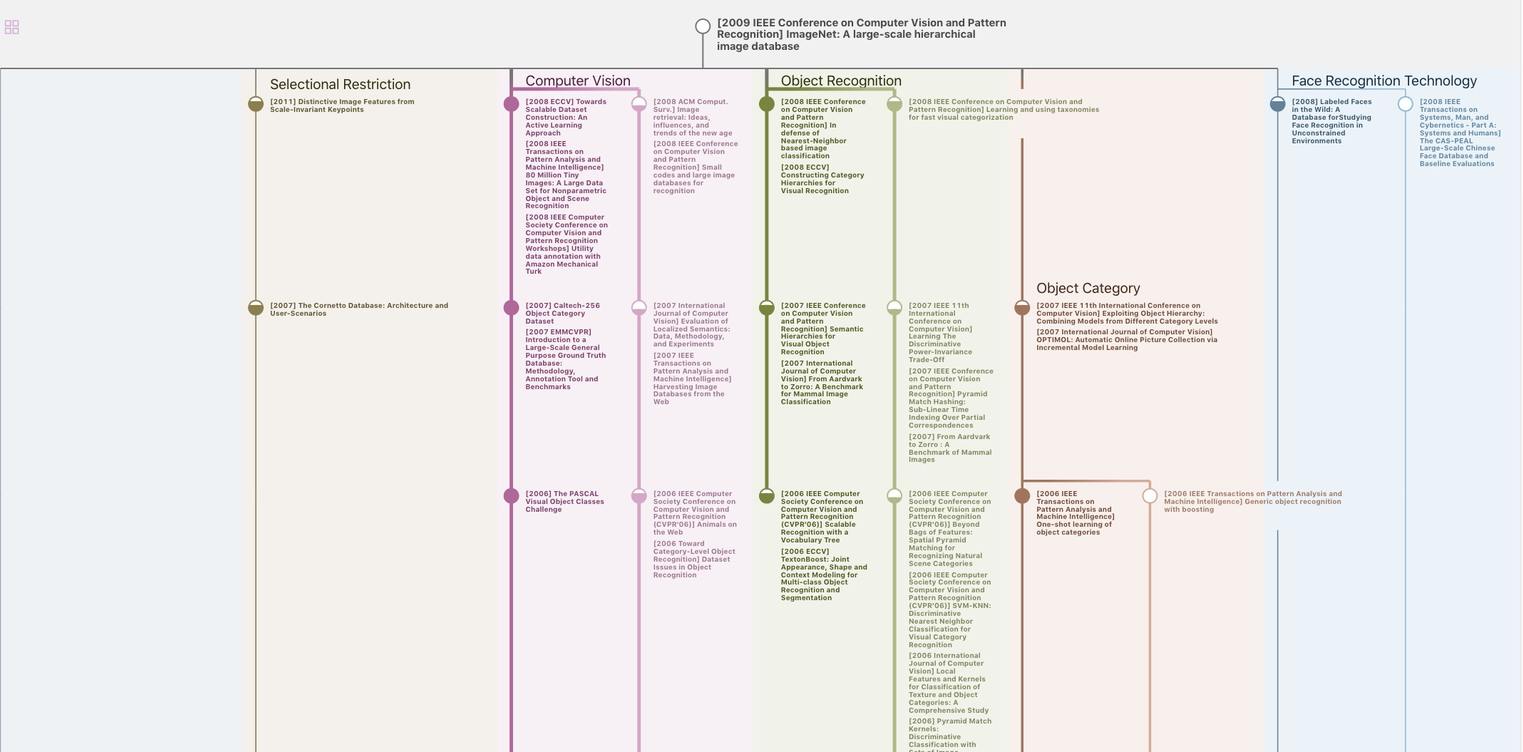
生成溯源树,研究论文发展脉络
Chat Paper
正在生成论文摘要