ContextLoc++: A Unified Context Model for Temporal Action Localization
IEEE transactions on pattern analysis and machine intelligence(2023)
摘要
Effectively tackling the problem of temporal action localization (TAL) necessitates a visual representation that jointly pursues two confounding goals, i.e., fine-grained discrimination for temporal localization and sufficient visual invariance for action classification. We address this challenge by enriching the local, global and multi-scale contexts in the popular two-stage temporal localization framework. Our proposed model, dubbed ContextLoc++, can be divided into three sub-networks: L-Net, G-Net, and M-Net. L-Net enriches the local context via fine-grained modeling of snippet-level features, which is formulated as a query-and-retrieval process. Furthermore, the spatial and temporal snippet-level features, functioning as keys and values, are fused by temporal gating. G-Net enriches the global context via higher-level modeling of the video-level representation. In addition, we introduce a novel context adaptation module to adapt the global context to different proposals. M-Net further fuses the local and global contexts with multi-scale proposal features. Specially, proposal-level features from multi-scale video snippets can focus on different action characteristics. Short-term snippets with fewer frames pay attention to the action details while long-term snippets with more frames focus on the action variations. Experiments on the THUMOS14 and ActivityNet v1.3 datasets validate the efficacy of our method against existing state-of-the-art TAL algorithms.
更多查看译文
关键词
unified contextloc++ model,localization,action
AI 理解论文
溯源树
样例
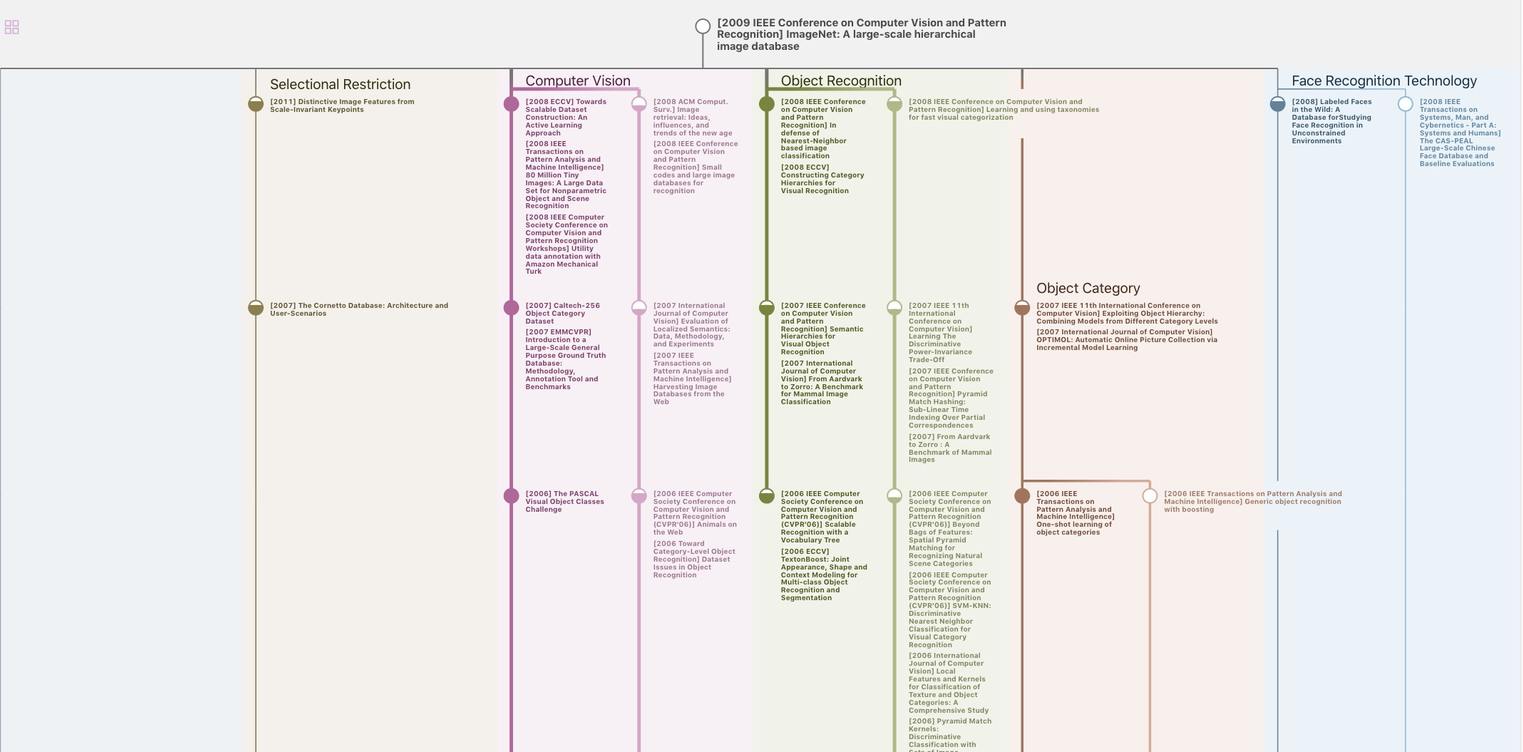
生成溯源树,研究论文发展脉络
Chat Paper
正在生成论文摘要