Fast Sparse Discriminative K-Means for Unsupervised Feature Selection.
IEEE transactions on neural networks and learning systems(2023)
摘要
Embedded feature selection approach guides subsequent projection matrix (selection matrix) learning through the acquisition of pseudolabel matrix to conduct feature selection tasks. Yet the continuous pseudolabel matrix learned from relaxed problem based on spectral analysis deviates from reality to some extent. To cope with this issue, we design an efficient feature selection framework inspired by classical least-squares regression (LSR) and discriminative K-means (DisK-means), which is called the fast sparse discriminative K-means (FSDK) for the feature selection method. First, the weighted pseudolabel matrix with discrete trait is introduced to avoid trivial solution from unsupervised LSR. On this condition, any constraint imposed into pseudolabel matrix and selection matrix is dispensable, which is significantly beneficial to simplify the combinational optimization problem. Second, the l2,p -norm regularizer is introduced to satisfy the row sparsity of selection matrix with flexible p . Consequently, the proposed FSDK model can be treated as a novel feature selection framework integrated from the DisK-means algorithm and l2,p -norm regularizer to optimize the sparse regression problem. Moreover, our model is linearly correlated with the number of samples, which is speedy to handle the large-scale data. Comprehensive tests on various data terminally illuminate the effectiveness and efficiency of FSDK.
更多查看译文
关键词
$\ell_{2,p}$ -norm regularizer,embedded feature selection,least-square regression (LSR),sparse discriminative K-means,trivial solution,weighted pseudolabel matrix
AI 理解论文
溯源树
样例
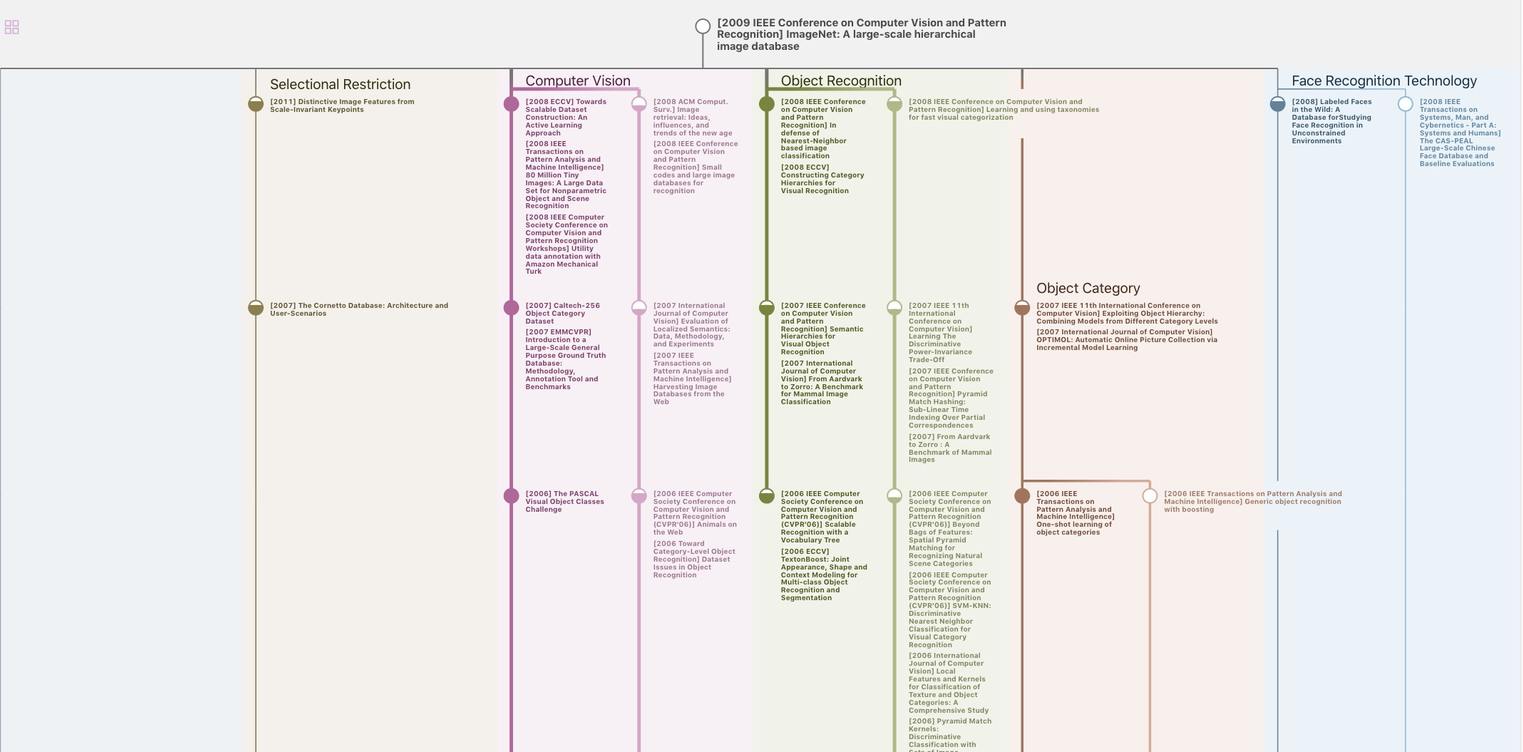
生成溯源树,研究论文发展脉络
Chat Paper
正在生成论文摘要