CebraEM: A practical workflow to segment cellular organelles in volume SEM datasets using a transferable CNN-based membrane prediction
bioRxiv (Cold Spring Harbor Laboratory)(2023)
摘要
Segmentation of large-volume datasets obtained by volume SEM techniques is a challenging task that generally requires a considerable amount of human effort. Despite recent advances in deep learning leading to the successful segmentation of cellular organelles in a variety of datasets, it is still challenging and time-consuming to produce the necessary data for training a convolutional neural network as well as to set up targeted post-processing pipelines to obtain a good quality full-volume semantic instance segmentation. We present CebraEM, a software package that uses a novel workflow for the segmentation of organelles in volume EM datasets, which helps to minimize the annotation time for the generation of training data. It relies on a generic CNN-based membrane prediction, followed by a well-established machine-learning pipeline that includes over-segmentation before random forest classification and graph multi-cut grouping. The workflow was tested for the segmentation of organelles on different datasets originating from various sample preparations and imaging modalities in volume SEM, in each case resulting in state-of-the-art semantic instance segmentations without additional post-processing. Importantly, by considerably simplifying the segmentation problem, CebraEM empowers single users with the ability to efficiently segment hundreds of gigabytes of data.
### Competing Interest Statement
The authors have declared no competing interest.
更多查看译文
关键词
membrane prediction,cellular organelles,volume sem datasets,cnn-based
AI 理解论文
溯源树
样例
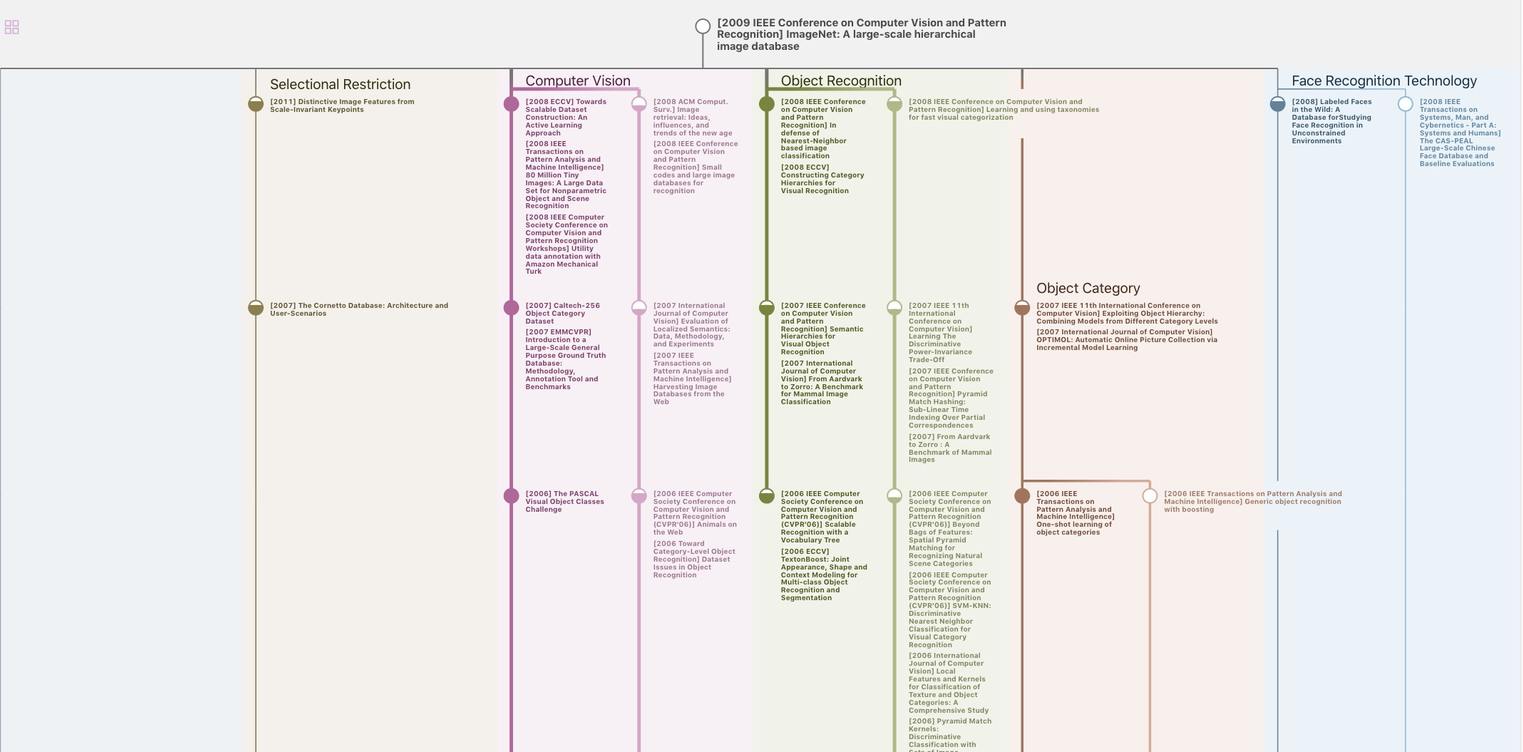
生成溯源树,研究论文发展脉络
Chat Paper
正在生成论文摘要