MCE-ST: Classifying crop stress using hyperspectral data with a multiscale conformer encoder and spectral-based tokens
International Journal of Applied Earth Observations and Geoinformation(2023)
摘要
Recently, transformers have achieved great success in a number of computer vision tasks due to their excellent ability to capture long-range feature dependencies. In contrast, convolutional neural networks (CNNs) are good at extracting local features. Given that the capture of short- and long-range band dependencies are both important for hyperspectral data classification, we propose MCE-ST, a convolution-transformer (conformer) based framework capable of exploiting the complementary strengths of transformers and CNNs. In contrast to the conventional transformer, which uses a linear projection for tokenization, the proposed MCE-ST uses a convolution-based tokenization method to extract local dependency between spectral bands. Moreover, since different hyperspectral samples may have different spans of local relationships, a multiscale conformer encoder (MCE) comprising two separate branches of depth-wise dilated convolution with different kernel sizes is used to extract the different spans of the local interactions between tokens. We conducted experiments on four salt stress datasets and one cassava disease dataset. The results show that the proposed MCE-ST outperforms the state-of-the-art techniques for crop stress classification using hyperspectral data. The code for MCE-ST is publicly available at https://github.com/Weejaa04/MCE-ST-GitHub.
更多查看译文
关键词
Stress classification,Disease classification,Hyperspectral,Deep learning,Transformer,Spectral-to-tokens
AI 理解论文
溯源树
样例
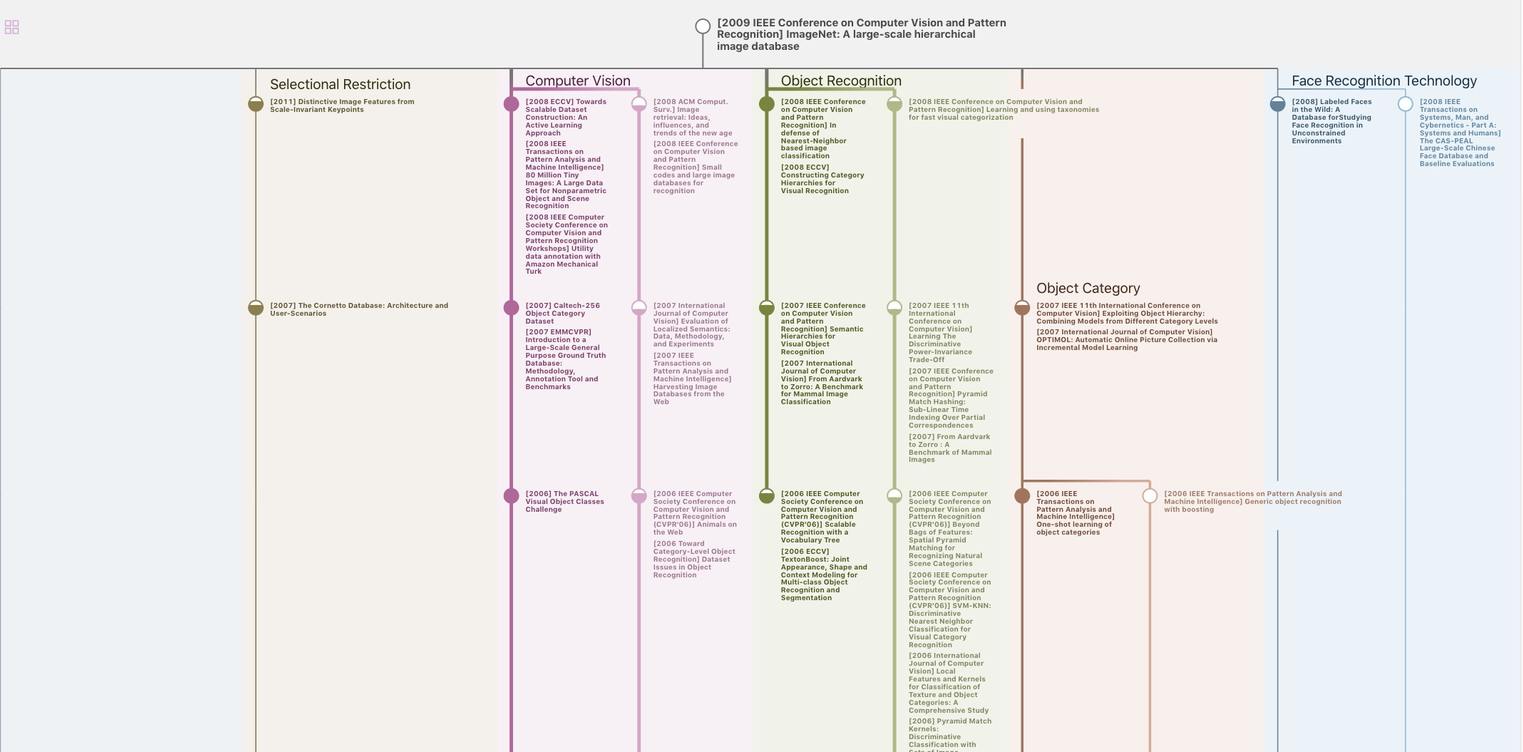
生成溯源树,研究论文发展脉络
Chat Paper
正在生成论文摘要